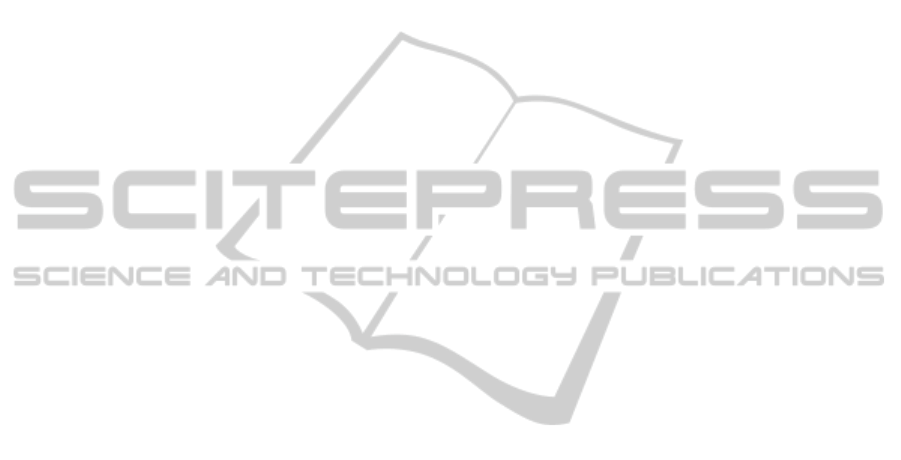
considered by National Health Fund (NFZ) when
negotiating future contracts. The goal of this paper is
to use a discrete event simulation to estimate the
next year’s demand for hospital emergency services
in the region. This, in turn, will help forecast the
volume of services to be contracted with providers
to cover the future needs of the population.
2 LITERATURE REVIEW
The substantial AU/EW simulation literature has
focused on the patient flows and throughput times
inside emergency departments (ED). Some authors
also incorporate the issue of staff scheduling.
Duguay and Chetouane (2007) used the DES model
to reduce patient waiting times and improve service
delivery in an ED in a regional hospital in Canada.
Based on simulation experiments, the authors
formulated a number of qualitative suggestions.
Ahmed and Alkhamis (2009) integrated simulation
with optimisation to analyse patient flows and
evaluate different staffing distributions. They were
able to find the optimal number of ED staff members
to maximise patient throughput and reduce patient
waiting time. Paul and Lin (2011) utilised the DES
model to identify the main causes of reduced ED
throughput. Zeng et al. (2012) used a discrete
simulation to improve the quality of care at an ED.
A simulation-based decision support system to assist
with planning processes in EDs was presented by
Abo-Hamad and Arisha (2013). The authors tested a
number of scenarios, e.g., variation in medical
staffing and “zero-tolerance” policy regarding
exceeding a 6-hour boarding time. They were able to
define the factors that have a great impact on
reducing the average lengths of stays.
Patient flows and staff scheduling are the
elements of a more complex problem, i.e.,
emergency department overcrowding. Because all
ED patients must be provided with medical
assistance, overcrowding is a serious problem and
simulation has proven to be an effective tool to
improve system performance. Paul and Reddy
(2010) searched the ED simulation literature from
1970 to 2006 and found 43 papers that modelled the
EDs and performed computer simulations. The
majority of the models were stochastic and the
preferred modelling technique was discrete event
simulation. Based on the review, the authors
discussed useful insights into the problems of ED
crowding. They were also able to list important
limitations that had to be addressed by future
simulation models.
In most published papers, the object under study is
an isolated ED unit and the goal is to improve the
unit’s internal processes. These models concentrate
on the current work of EDs, try to identify the causes
of overcrowding and suggest strategies to overcome
them. Our approach may be defined as upper-level
strategic modelling because it concerns the broader
issue of covering the demand for hospital emergency
services in an entire region. Our work makes the
following contributions. First, we try to forecast the
next year’s demand for hospital emergency services
to be met by all EDs in the region. Second, we
estimate the expected volume and structure of the
services to be delivered by particular EDs to cover
the next year’s emergency needs separately for every
ED. The general idea behind the study was described
in (Mielczarek and Uziałko-Mydlikowska, 2012b).
3 REGIONAL EMS SYSTEM
Lower Silesia is the fourth largest region in Poland.
It is divided into 5 sub-regions, 29 administrative
districts and 169 communes. The capital of the
region is Wroclaw, which is a large academic and
industrial centre. In 2010, there were 91 hospitals
located in the area with 39 AUs and 13 hospital
EWs. Our study concerns the services offered in
2010 by AUs and EWs located in 2 (of a total of 5)
sub-regions. These 2 sub-regions are referred to in
the paper as the Wrocław Region (WR). This area
encompasses the capital of Lower Silesia (Wrocław)
and 8 other nearby districts (for a total of 9 districts).
In 2010, 12 AUs and 5 EWs operated in WR.
Data for the study were obtained from the NFZ
regional branch in Wrocław for the year 2010. Two
basic data sets were analysed. The first included
information on 183,517 emergency visits by patients
residing in WR who arrived in AUs/EWs located in
the Lower Silesia area. The records from the second
data set revealed 201,636 patients with any
residence codes who were registered in AUs/EWs
deployed in the WR. To protect anonymity, personal
data were deleted from the files.
Regionalisation is not employed in the Polish
healthcare system. This means that patients can
decide where to go for medical assistance. In most
cases, the neighbourhood hospital is selected.
However, to properly estimate the next year’s
demand for hospital emergency services with regard
to WR units, we had to consider patient choices for
the place of treatment.
SIMULTECH2013-3rdInternationalConferenceonSimulationandModelingMethodologies,Technologiesand
Applications
198