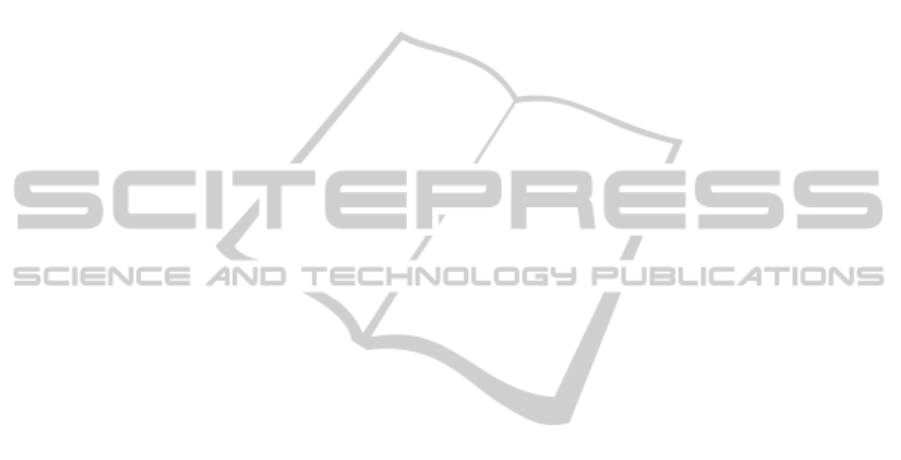
a conventional search, using an evolutionary algo-
rithm(Geiger et al., 2010).
4 CONCLUSIONS
The motivation of Thesis 1 reveals the relations be-
tween Computational Intelligence (CI) and Artificial
General Intelligence (AGI). The understanding pro-
vided by these scientific disciplines is indispensable
for every approach to solve imprecisely posed prob-
lems.
In the same way as problem solving is the objec-
tive of Artificial Intelligence (AI), imprecisely posed
problem solving can be understood as the objective
of AGI. Two well known experts of AGI, Richard
Loosemore and Ben Goertzel wrote in a recent paper
(Loosemore and Goertzel, 2012):
“There are two possible types of intelligence
speedup: one due to faster operation of an intelli-
gent system (clock speed increase) and one due to
an improvement in the type of mechanismus that im-
plement the thought process (’depth of thought’ in-
crease).”
But as speedup may be limited by quantum me-
chanical limitations for the hardware and by the in-
tractability of many problems, the second type of in-
telligence amplification seems to be more promis-
ing. Really intelligent systems must be “self-
understanding”. To solve a problem means first, to
understand this problem, but secondly to understand
also the search process and context for its solution.
Many industrial systems like plants are not only tech-
nical systems but also social systems, and as such sys-
tems they enable emergent events. The complexity
residing in those systems requires a parallel design of
it’s parts. For that reason, plant engineering always
confronts imprecisely posed problems. The “depth of
thought” in engineering reasoning is therefor strongly
related to the ability for solving imprecisely posed
problems. The methods of CI and AGI are suitable to
create strategies for that task. The presented strategies
correspond to the strongest confinements of common
designs.
REFERENCES
Aztiria, A., Izaguirre, A., and Augusto, J. C. (2010). Learn-
ing patterns in ambient intelligence environments: a
survey. Artificial Intelligence Review, 34:35–51.
Benferhat, R. (2010). Interventions and belief change in
possibilistic graphical models. Artificial Intelligence,
174(2):177–189.
D
¨
urrbaum, A., Kroll, A., and Sommer, H. (2012).
Ci-methoden zur koordination und entkopplung
komplexer systeme. at-Automatisierungstechnik,
60(10):600–610.
D
¨
urrbaum, A., Scherm, W., and Sommer, H. (2008). Sys-
temidentifikation mittels glaubenskalk
¨
ul. Automa-
tisierungstechnik, 7:350–356.
Geiger, A., Sommer, H., and Kroll, A. (2010). Koevolu-
tionaerer algorithmus zur analyse struktureller steuer-
barkeit - eine methode der ’komplexen netzwerke’
zur systemanalyse. In F. Hoffmann, E. H., edi-
tor, 20. Workshop Computational Intelligence, vol-
ume 33, pages 235– 249, Karlsruhe. Institut fuer
Angewandte Informatik/Automatisierungstechnik, In-
stitut fuer Technology.
Goertzel, B., Ikle, M., and Wigmore, J. (2012). Foundat-
tions ’AGI’ Mind Architecture. Atlantis Press, Ams-
terdam.
Goertzel, B. and Pennachin, C. (2007). Artificial General
Intelligence. Springer, New York.
Gross, B. (1999). Gesamtoptimierung verfahrenstech-
nischer systeme mit evolution
¨
aren algorithmen. In
Fortschritt-Berichte VDI, volume 3, page 131. VDI-
Verlag, D
¨
usseldorf.
Jager, R. R. (1994). Aggregation operators and fuzzy sys-
tems modeling. Fuzzy Sets and Systems, 67(2):129–
145.
K
¨
opcke, H. (2004). Optimierung von aufstel-
lungsentw
¨
urfen von chemieanlagen durch constraint
programming. In 14 Workshop Fuzzy-Systeme und
Computational Intelligence, page 139, Dortmund.
Kragh, H. (2013). Testability and epistemic shifts in mod-
ern cosmologiy. Studies in History and Philosopy of
Modern Physics. to appear.
Kroll, A. (2013). Computational Intelligence, Eine
Einf
¨
uhrung in Probleme, Methoden und technische
Anwendungen. Oldenbourg Verlag, M
¨
unchen.
Kroll, A. and Sommer, H. J. (2013). Coordination of dis-
tributed control systems. to appear in International
Journal of Computing Anticipatory Systems.
Ljung, L. (1987). System Identification, Theory for the
User. PTR Prentice-Hall, Inc., London.
Loosemore, R. and Goertzel, B. (2012). Why
an intelligence explosion is probable.
http://www.fhi.ox.ac.uk/archived-events/winter-
conference.
Sommer, H. (1995). The logic in knowledge processing
formalisms. In H.J., Z., editor, EUFIT’95, volume 31,
pages 274–278, Aachen.
Sommer, H. and Schreiber, L. (2012). Is logic in the mind or
in the world? why a philosophical question can affect
the understanding of intelligence. Journal of Artificial
General Intelligence, 3(1):25–47.
Sun, R. (2009). Theoretical status of computational cogni-
tive modeling. Cognitive Systems research, 10:124–
140.
van Beek, T. J. and Tomiyama, T. (2012). Structured work-
flow approach to support evolvability. Advanced En-
gineering Informatics, 26:487–501.
InNeedofMethodstoSolveImpreciselyPosedProblems
299