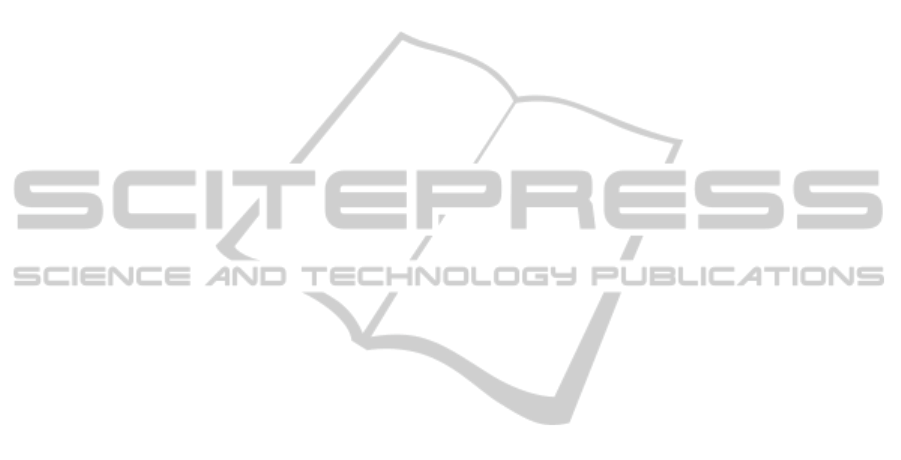
• The state of the model – including the information
that is sent at a particular time – has an explicit
representation. This facilitates the creation and
application of measures that describe global, dy-
namic properties of the system enabling the com-
parison of systems that would otherwise be too
different to be compared in a more direct way.
• The model focuses on the interactions between
its information processing entities. The building
blocks of the model are designed such that the
modeling of complex interactions is facilitated.
One aspect of this is the ability of messages to ex-
ist over arbitrary long time intervals which, e.g.,
enables modeling of the spatial propagation of a
message.
In our future work we will use the presented model
to investigate phenomena observed in neuronal infor-
mation processing systems as they are a prime exam-
ple of complex systems. Their operation involves a
substantial variety of mechanisms, e.g., fast signal-
ing through action potentials, complex integration of
signals due to dendrite morphology and electrotonic
properties, change of processing characteristics via
neuromodulators, or the constant adjustment of net-
work topology by means of synaptic plasticity (Koch,
2004; Shepherd and Grillner, 2010). Furthermore,
nervous systems develop by evolutionary processes
over millions of years and are able to “bootstrap”
themselves by a process of self-organized growth
within a timescale of weeks to months (Butler and
Hodos, 2005; Squire et al., 2008).
Among the first phenomena that we want to anal-
yse are the mechanisms of self-organization utilized
during nervous system development such as biochem-
ical guidance cues which are used in the context of
neuron migration and axonal path finding.
We think that modeling and simulation of these
neuronal systems will not only result in a better un-
derstanding of the particular processes themselves but
may provide also insight into more general principles
of self-organization and emergence.
REFERENCES
Allan, R. (2010). Survey of agent based modelling and sim-
ulation tools. Technical report, STFC Daresbury Lab-
oratory, Computational Science and Engineering De-
partment, Daresbury, Warrington WA4 4AD.
Bankes, S. (1993). Exploratory modeling for policy analy-
sis. Operations Research, 41(3):435–449.
Bonabeau, E. (2002). Agent-based modeling: Methods and
techniques for simulating human systems. Proceed-
ings of the National Academy of Sciences of the United
States of America, 99(Suppl 3):7280–7287.
Brun, R. and Rademakers, F. (1996). Root - an object ori-
ented data analysis framework. In AIHENP’96 Work-
shop, Lausane, volume 389, pages 81–86.
Butler, A. and Hodos, W. (2005). Comparative Vertebrate
Neuroanatomy: Evolution and Adaptation. Wiley.
Caron-Lormier, G., Humphry, R., Bohan, D., Hawes, C.,
and Thorbek, P. (2008). Asynchronous and syn-
chronous updating in individual-based models. Eco-
logical Modelling, 212(3-4):522–527.
DeAngelis, D. L. and Mooij, W. M. (2005). Individual-
based modeling of eecological and evolutionary pro-
cesses. Annual Review of Ecology, Evolution, and Sys-
tematics, 36(1):147–168.
Dennett, D. (1998). Brainchildren: Essays on Designing
Minds, 1984-1996. Representation and Mind Series.
Mit Press.
Evans, K. M. (2001). Larger than life: Digital creatures
in a family of two-dimensional cellular automata. In
Cori, R., Mazoyer, J., Morvan, M., and Mosseri, R.,
editors, Discrete Models: Combinatorics, Computa-
tion, and Geometry, DM-CCG 2001, volume AA of
DMTCS Proceedings, pages 177–192. Discrete Math-
ematics and Theoretical Computer Science.
Gardner, M. (1970). Mathematical games: The fantastic
combinations of John Conway’s new solitaire game
‘Life’. j-SCI-AMER, 223(4):120–123.
Holland, J. (1998). Emergence. Oxford University Press,
New York.
Koch, C. (2004). Biophysics of Computation: Information
Processing in Single Neurons. Computational Neuro-
science Series. Oxford University Press, USA.
Langton, C. G. (1986). Studying artificial life with cel-
lular automata. Physica D: Nonlinear Phenomena,
22(13):120 – 149. Proceedings of the Fifth Annual
International Conference.
Lempert, R. J., Schlesinger, M. E., and Bankes, S. C.
(1996). When we dont know the costs or the bene-
fits: Adaptive strategies for abating climate change.
Change, Climactic Change, 33:235–274.
Maslow, A. and Wirth, A. (1966). The psychology of sci-
ence: a reconnaissance, volume 8 of The John Dewey
Society lectureship series. Harper & Row.
Minar, N., Burkhart, R., Langton, C., and Askenazi, M.
(1996). The swarm simulation system: A toolkit for
building multi-agent simulations. Santa Fe Institute.
Newman, M. (2003). The structure and function of complex
networks. SIAM Review, 45(2):167–256.
Newman, M. E. J. (2011). Resource letter cs-1: Complex
systems. American Journal of Physics, 79:800–810.
North, M., Collier, N., and Vos, J. (2006). Experiences cre-
ating three implementations of the repast agent mod-
eling toolkit. ACM Trans. Model. Comput. Simul.,
16(1):1–25.
Railsback, S., Lytinen, S., and Jackson, S. (2006). Agent-
based simulation platforms: Review and development
recommendations. Simulation, 82(9):609–623.
Reynolds, C. (1987). Flocks, herds and schools: A dis-
tributed behavioral model. In Proceedings of the 14th
ICINCO2013-10thInternationalConferenceonInformaticsinControl,AutomationandRobotics
520