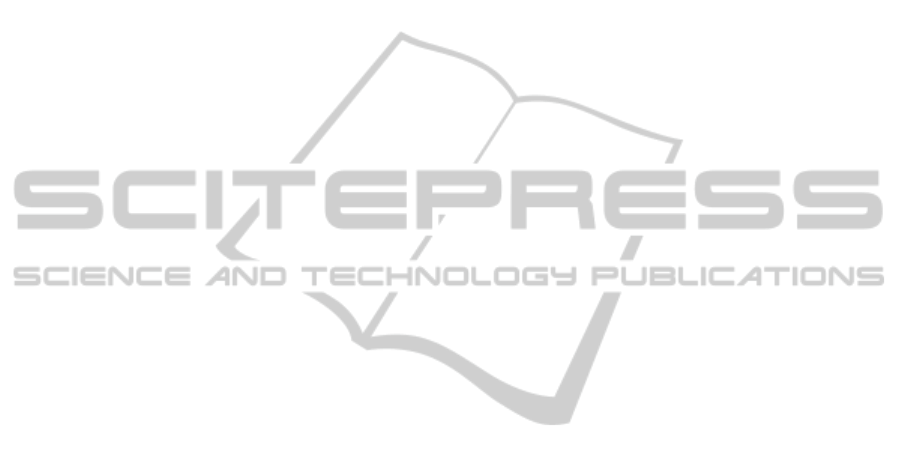
tempt to study the techniques from this point of view, i.e.; usability. We identified
three main characterizing criteria that permit to distinguish between aggregation tech-
niques reported in this paper: The information need (the query), the user profile (lim-
ited here to the user task) and information sources content.
The study of the aggregation techniques shows that each of the techniques can be
suitable for a specific situation. This study confirms previously mentioned assertion,
i.e.; aggregated search has never been treated as a whole [18]. Each aggregation tech-
nique was developed for a specific situation. Some techniques were developed for
some kind of queries, some others were developed for a very specific user profile and
others are most general and developed according to sources content types. Thus, it is
important to select a context or an application case before thinking about the devel-
opment or the use of an aggregation technique.
Undeniably, this study needs empirical experiments in order to confirm findings
and conclusions. Other characterizing criteria such as those related to visualization
constraints, semantic aspects and context might also be considered. We are interesting
in future work to confirm the finding by empirical experiments and to develop dy-
namic aggregation technique.
References
1. V. Murdock and M. Lalmas, editors. SIGIR 2008 Workshop on Aggregated Search, New
York, NY, USA, ACM. 1, 3.7. 2008.
2. S. Sushmita, M. Lalmas, A. Tombros . Using digest pages to increase user result space:
Preliminary designs. Proceedings of the 2008 ACM SIGIR Workshop on Aggregated
Search. Singapore, July 2008.
A. Kopliku, K., M. Boughanem. Aggregated search: potential, issues and évaluation. Tech-
nical report: IRIT/RT–2009-4–FR, IRIT, septembre 2009.
3. J. Callan, Distributed information retrieval. In W. Croft (Ed.), Advances in Information.
Retrieval (pp. 127–150). Hingham, MA, USA: Kluwer Academic.2000.
4. J. Callan and M. Connell. Query-based sampling of text databases. ACM Transactions on
Information Systems, 19:97–130, 2001.
5. R. Caruana. Multitask learning. Machine Learning, 28:41–75, July 1997.
6. J. Caverlee, L. Liu, and J. Bae. Distributed query sampling: A quality conscious approach.
In Proceedings of the 29th annual international ACM SIGIR conference on Research and
development in information retrieval, SIGIR ’06, pages 340–347, New York, NY, USA,
2006.
7. K. Chen, R. Lu, C. K. Wong, G. Sun, L. Heck, and B. Tseng. Trada: Tree based ranking
function adaptation. In Proceeding of the 17th ACM conference on Information and
knowledge management, CIKM ’08, pages 1143–1152, New York, NY, USA, 2008..
8. L. Gravano, Chen-Chuan K. Chang, H. García-Molina, and A. Paepcke. Starts: Stanford
proposal for internet meta-searching. In Proceedings of the 1997 ACM SIGMOD interna-
tional conference on Management of data, SIGMOD ’97, pages 207–218, New York, NY,
USA, 1997.
9. M. Shokouhi. Central-rank-based collection selection in uncooperative distributed infor-
mation retrieval. In Proceedings of the 29th European conference on IR research, ECIR’07,
pages 160–172, Berlin, Heidelberg, Springer-Verlag, 2007.
10. W. Rivadeneira and B. B. Bederson. A study of search result clustering interfaces: Compar-
ing textual and zoomable user interfaces. Technical report, University of Maryland HCIL
Technical Report HCIL-2003-36, 2003.
72