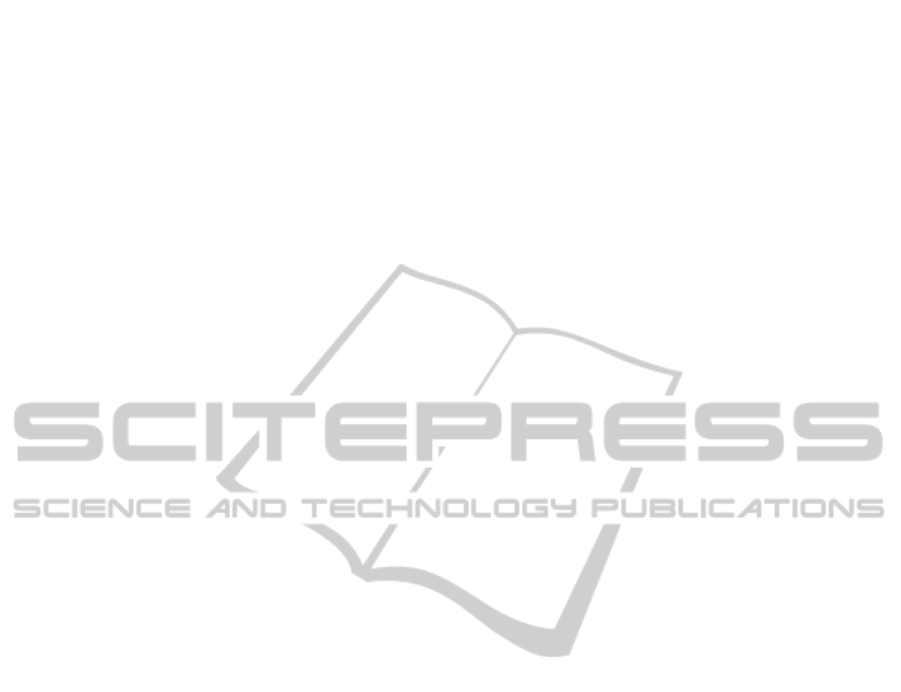
Ignacik, J., 2008. Informacyjność wybranych kryteriów
doboru kandydatów do szkolenia w piłce ręcznej
młodych szczypiornistów. [Informativeness for the
selection criteria of young handball players]. Zeszyty
Metodyczno – Naukowe. Studia Doktoranckie AWF
Katowice, 25, 319-333.
Keogh, J. W. L., Hume, P. A., Mellow, P., & Pearson, S.
(2005). The use of anthropometric variables to predict
bench press and squat in well–trained strength athletes.
In Q. Wang (Chair), 23
rd
International Symposium on
Biomechanics in Sports. Symposium conducted at the
meeting of the International Society of Biomechanics
in Sports, Beijing, China. Retrieved from
http://w4.ub.unikonstanz.de/cpa/article/view/ 841.
Klocek, T., Spieszny, M., Szczepanik, M., 2002.
Komputerowe testy zdolności koordynacyjnych
[Computer tests of coordination abilities]. Warsaw,
Poland: Centralny Ośrodek Sportu.
Magiera, A., Ryguła I., 2007. Biometric model and
classification functions in sport climbing. Journal of
Human Kinetics, 18, 87-98.
Maszczyk, A., 2008. Określenie wartości diagnostycznej i
predyktywnej wybranych cech budowy ciała,
sprawności fizycznej ogólnej i specjalnej 14 – 15-
letnich oszczepników [The determination of diagnostic
and predictive value of selected morfological
parameters, general and specific finess among 14-15-
year old javelin throwers]. Zeszyty Metodyczno –
Naukowe. Studia Doktoranckie AWF Katowice, 25,
23-37.
Mayhew, J. L., Bird, M., Cole, M. L., Koch, A. J.,
Jacques, J. A., Ware, J. S., Fletcher, K. M., 2005.
Comparisons of the backward overhead medicine ball
throw to power production in college football players.
Journal of Strength and Conditioning Research, 19,
514-518.
Mayhew, J. L., McCormick, T. P., Piper, F. C., Kurth, A.
M., Arnold, M. D., 1993. Relationships of body
dimensions to strength performance in novice
adolescent male powerlifters. Pediatric Exercise
Science, 5, 347-356.
McArdle, W. D., Katch, F. I., Pechar, G. S., Jacobson L.,
Ruck, S., 1972. Reliability and interrelationships
between maximal oxygen intake, physical work
capacity and step-test scores in college women.
Medicine and Science in Sports, 4, 182-186.
Mirwald, R. L., Baxter-Jones, A. D. G., Bailey, D. A.,
Beunen, G. P., 2002. An assessment of maturity from
anthropometric measurements. Medicine and Science
in Sports Exercise, 34, 689-694.
Płóciennik, Ł., 2012. The diagnostic value of criteria for
selection among 19-23-year-old powerlifters
(Unpublished doctoral dissertation). Academy of
Physical Education and Sport, Gdansk, Poland.
Ryguła, I., 2003. Proces badawczy w naukach o sporcie
[Research process in science of sport]. Katowice,
Polska: Uniwersytet Śląski
, Katowice.
Saavedra, J. S., Escalante, Y., Rodriguez, F. A., 2010. A
Multivariate Analysis of Performance in Young
Swimmers. Pediatric Exercise Science, 22, 135-151.
Salonia, M. A., Chu, D. A., Cheifetz, P. M., Freidhoff, G.
C., 2004. Upper-body power as measured by medicine
ball throw distance and its relationship to class level
among 10- and 11-year-old female participants in club
gymnastics. Journal of Strength and Conditioning
Research, 18, 695-702.
Sampaio, J., Janeira, M., Ibáñez, S., Lorenzo, A., 2006.
Discriminant analysis of game – related statistics
between basketball guards, forwards and centres in
three professional leagues. European Journal of Sport
Science, 6, 173-178.
Shephard, R. J., 1991. Body composition in biological
anthropology. London: Cambridge University Press.
Watson, P. E., Watson, I. D., Batt, R. D., 1980. Total body
water volumes for adult males and females estimated
from simple anthropometric measurements. American
Journal of Clinical Nutrition, 33, 27-39.
Winwood, P. W., 2011. Strongman: Strength and
conditioning practices, and the inter – relationships
between strength, anthropometrics and performance
(Master’s thesis). Auckland University of Technology,
Auckland, USA.
Woźniak-Kozak, E., Laurentowska, M., & Tokłowicz B.
(1986). Wybrane próby czynnościowe określające
wydolność układu krążenia [Selected functional tests
determining cardiovascular capacity]. In W. Rożynek-
Łukanowska (Ed.), Wybrane ćwiczenia z fizjologii
wysiłku fizycznego i sportu [Selected exercises from
physiology of exercise and sport] (pp. 81-131).
Poznań, Poland: Wydawnictwo Akademii Wychowania
Fizycznego.
Zawadzki, B., Strelau, J., Szczepaniak, P., & Śliwińska M.
(1998). Inwentarz Osobowości NEO – FFI Costy i
McCrae [Costa`s and McCrae`s Personality Inventory
NEO - FFI]. Warsaw, Poland: Polskie Towarzystwo
Psychologiczne.
PowerliftingatJuniorLevel-SelectionParadigm
123