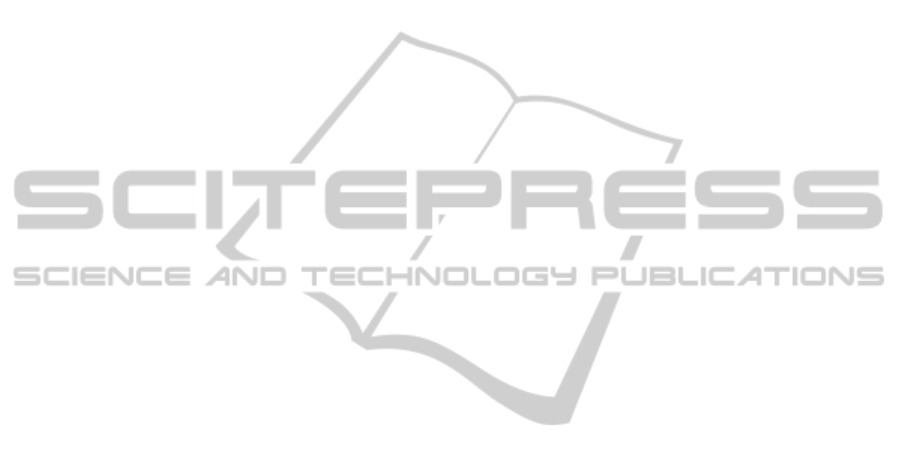
REFERENCES
Bianchi, L., Sami, S., Hillebrand, A., Fawcett, I.,
Quitadamo, L., and Seri, S. (2010). Which phys-
iological components are more suitable for visual
ERP based brain-computer interface? A preliminary
MEG/EEG study. Brain Topography, 23(2):180–185.
Birbaumer, N. (2006). Breaking the silence: Brain-
computer interfaces (BCI) for communication and
motor control. Psychophysiology, 43(6):517–532.
Donchin, E., Spencer, K., and Wijesinghe, R. (2000). The
mental prosthesis: Assessing the speed of a P300-
based brain-computer interface. IEEE Transactions
on Rehabilitation Engineering, 8(2):174–179.
Dunlop, M. D. and Crossan, A. (2000). Predictive text entry
methods for mobile phones. Personal Technologies,
4(2-3):134–143.
Farwell, L. and Donchin, E. (1988). Talking off the top of
your head: toward a mental prosthesis utilizing event-
related brain potentials. Electroencephalography and
Clinical Neurophysiology, 70(6):510 – 523.
Grover, D. L., King, M. T., and Kushler, C. A. (1998). Re-
duced keyboard disambiguating computer. U.S. Patent
No. 5,818,437. Washington, DC: U.S. Patent and
Trademark Office.
Kececi, H., Degirmenci, Y., and Atakay, S. (2006). Ha-
bituation and dishabituation of P300. Cognitive and
Behavioral Neurology, 19(3):130–134.
Kleih, S., Nijboer, F., Halder, S., and K
¨
ubler, A. (2010).
Motivation modulates the P300 amplitude during
brain-computer interface use. Clinical Neurophysiol-
ogy, 121(7):1023 – 1031.
Kleih, S. C., Kaufmann, T., Hammer, E., Pisotta, I., Pi-
chiorri, F., Riccio, A., Mattia, D., and K
¨
ubler, A. (in
press). Motivation and SMR-BCI: Fear of failure af-
fects BCI performance. In Proceedings of the Fifth
International BCI Meeting.
Krusienski, D., Sellers, E., McFarland, D., Vaughan, T.,
and Wolpaw, J. (2008). Toward enhanced P300
speller performance. Journal of Neuroscience Meth-
ods, 167(1):15 – 21.
Lu, J., Speier, W., Hu, X., and Pouratian, N. (2013). The
effects of stimulus timing features on P300 speller
performance. Clinical Neurophysiology, 124(2):306
– 314.
Mak, J. and Wolpaw, J. (2009). Clinical applications of
brain-computer interfaces: Current state and future
prospects. IEEE Reviews in Biomedical Engineering,
2:187–199.
Mangun, G. R. and Buck, L. A. (1998). Sustained visual-
spatial attention produces costs and benefits in re-
sponse time and evoked neural activity. Neuropsy-
chologia, 36(3):189 – 200.
McFarland, D. J., Sarnacki, W. A., Townsend, G., Vaughan,
T., and Wolpaw, J. R. (2011). The P300-based brain-
computer interface (BCI): Effects of stimulus rate.
Clinical Neurophysiology, 122(4):731 – 737.
Murata, A. and Uetake, A. (2001). Evaluation of mental fa-
tigue in human-computer interaction - Analysis using
feature parameters extracted from event-related poten-
tial. In 10th IEEE International Workshop on Robot
and Human Interactive Communication, pages 630–
635.
Polich, J. and Kok, A. (1995). Cognitive and biological de-
terminants of P300: An integrative review. Biological
Psychology, 41(2):103 – 146.
Schalk, G., McFarland, D., Hinterberger, T., Birbaumer, N.,
and Wolpaw, J. (2004). Bci2000: A general-purpose
brain-computer interface (BCI) system. IEEE Trans-
actions on Biomedical Engineering, 51(6):1034–
1043.
Sellers, E. W. and Donchin, E. (2006). A P300-based
brain–computer interface: Initial tests by ALS pa-
tients. Clinical Neurophysiology, 117(3):538 – 548.
Sellers, E. W., Krusienski, D. J., McFarland, D. J., Vaughan,
T. M., and Wolpaw, J. R. (2006). A P300 event-related
potential brain–computer interface (BCI): The effects
of matrix size and inter stimulus interval on perfor-
mance. Biological Psychology, 73(3):242 – 252.
Shih, J. J., Townsend, G., Krusienski, D. J., Shih,
K. D., Shih, R. M., Heggeli, K., Paris, T., and
Meschia, J. F. (2013). Comparison of checker-
board P300 speller vs. row-column speller in
normal elderly and aphasic stroke population.
Paper presented at the Fifth International Brain-
Computer Interface Meeting, Asilomar Confer-
ence Grounds, Pacific Grove, CA. Retrieved from
http://castor.tugraz.at/doku/BCIMeeting2013/020.pdf.
Wang, C., Guan, C., and Zhang, H. (2005). P300 brain-
computer interface design for communication and
control applications. In 27th Annual International
Conference of the Engineering in Medicine and Bi-
ology Society IEEE-EMBS 2005, pages 5400–5403.
Wolpaw, J. R., Birbaumer, N., McFarland, D. J.,
Pfurtscheller, G., and Vaughan, T. M. (2002). Brain-
computer interfaces for communication and control.
Clinical Neurophysiology, 113(6):767–791.
NEUROTECHNIX2013-InternationalCongressonNeurotechnology,ElectronicsandInformatics
40