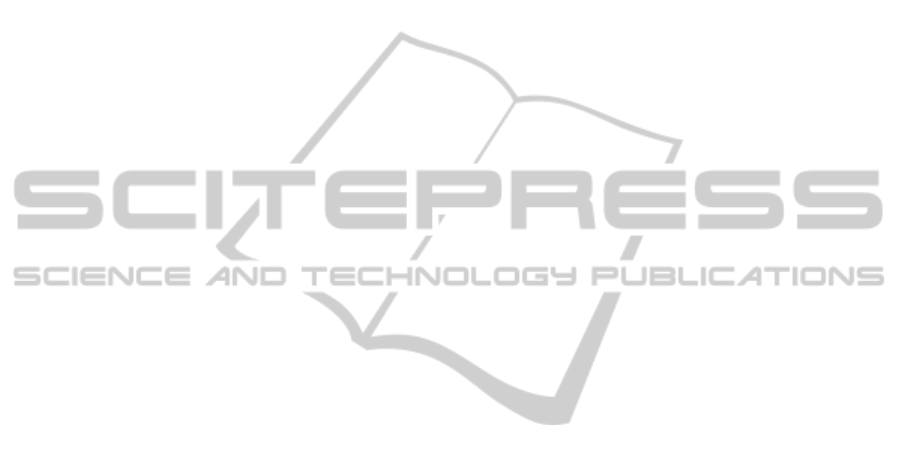
6 FUTURE DEVELOPMENTS
To face the problem discussed in this work, the
authors became aware of some problems affecting
the operational functioning of the Emergency
Department.
In particular it has come to light that the triage
phase is not carried out with an adequate accuracy.
In fact, a considerable percentage of hospital
admissions (37%) is ascribable to patients
previously identified with green code, while the
greatest part of such category is supposed to be
discharged, or in the worst cases, sent to OBI or SS.
On another hand, it has been pointed out that the
great pressure on ED physicians, caused by white
and green codes that usually represent the majority
of ED accesses, has no reasons to exist. It is a non
sense to wear out and divert such kind of emergency
specialists with high frequency visits forced by the
significant number of patients affected by low
critical diseases. This kind of patients could be
easily visited by young specializing doctors as
already happens in the US. For such a reason, the
authors are currently building a discrete event
simulator in order to evaluate a possible ED re-
organization. This re-organization will have to take
into consideration the creation of a different path for
white and green-coded patients among the hospital,
in order to allow emergency specialists to take care
of real urgent cases in a better way.
REFERENCES
Asplin, B. R., Magid, D. J., Rhodes, K. V., Solberg, L. I.,
Lurie, N., Camargo, C.A., 2003. A conceptual model
of emergency department crowding. In Annals of
Emergency Medicine, vol.42, Issue 2, pag. 173-180
Baer, R. B., Pasternack, J. S., et al. 2001. Recently
discharged inpatients as a source of emergency
department overcrowding. In Acad Emerg Med 8(11)
Briano, E., Caballini, C., Mosca, R., Revetria, R., Testa,
A., 2010. Proposing a system dynamic approach to
assess and improve Italian ports competitiveness.
Proceedings of the 12th WSEAS Conference on
Automatic Control, Modelling and Simulation
Cassettari L., Dagnino F., Mosca M., Revetria R., 2010.
Analysis of a urban route traffic flow exploiting the
system dynamic model. Proceedings of the 4th
WSEAS International Conference on Computer
Engineering and Applications (CEA’10), Cambridge,
USA, January 27-29, 2010.
Cassettari L., Mosca R., Revetria R. Rolando F., 2011.
Sizing of a 3,000,000t Bulk Cargo Port through
Discrete and Stochastic Simulation Integrated with
Response Surface Methodology Techniques.
Proceedings of 11th WSEAS International Conference
on Systems Theory And Scientific Computation
(ISTASC '11), Florence, Italy, August 23-25.
Cassettari, L., Mosca, R., Revetria, R., 2012. Monte Carlo
Simulation Models Evolving in Replicated Runs: A
Methodology to Choose the Optimal Experimental
Sample Size. In Mathematical Problems In
Engineering, 2012 Hindawi Publishing, Article
Number: 463873 DOI: 10.1155/2012/463873.
Frigato, A., Mosca, M., Schenone, M., 1999. Risk
management applied to project management and
project control. Proceedings of MIC 99- IASTED
Symposium on Modelling, Identification and Control,
Innsbruck (Austria).
Jack, B. W., Chetty, V. K., et al. 2009. A Reengineered
Hospital Discharge Program to Decrease
Rehospitalization: A Randomized Trial. In Ann Intern
Med. 150(3).
Homer, J. B. and Hirsch G. B., 2006. System Dynamics
Modelling for Public Health: Background and
Opportunities. In Am J Public Health 96(3).
Lattimer, V., Brailsford, S., Turnbull, J., Tarnaras, P.,
Smith, H., George, S., Gerard, K., Maslin-Prothero S.,
2004. Reviewing emergency care systems I: insights
from system dynamics modelling. In Emerg Med J
2004, n.21, 685-691.
Morrison, J. B., Rudolph, J. W., 2011. Learning from
Accident and Error: Avoiding the Hazards of
Workload, Stress, and Routine Interruptions in the
Emergency Department. Academic Emergency
Medicine, Vol.18, Issue 12, Dec. 2011.
Morrison, J. B., Wears, R. L., 2011. Emergency
Department Crowding: Vicious Cycles in the ED. 4
th
Mayo Clinic Conference on Systems Engineering &
Operations Research in Health Care Proceedings,
Rochester, MN.
Mosca, R., Giribone, P., 1982. Optimal lenght in O.R.
simulation experiments of large scale production
system. Proceedings of IASTED "Modelling,
Identification and Control", Davos (CH), 1982, pp.
78-82.
Mosca, R., 2002. Using HLA as a framework for supply
chain management: Project web-integrated logistics
designer. Simulation 78 (5 SPEC.) ,SCS, pp. 289-292.
Mosca, R., Bruzzone, A.G., Revetria R., 2002.
Cooperation in Maritime Training Process using
Virtual Reality Based and HLA Compliant Simulation.
Proceedings of XVII International Port Conference.
January 27-29, Alexandria Egypt.
Mosca, R., Cassettari, L., Revetria, R., Magro, L., 2005.
Simulation as Support for production planning in
Small & Medium Enterprise: A Case Study.
Proceedings of Winter Simulation Conference 2005,
Orlando (FL), 5-7 December 2005.
Mosca, R., Bruzzone, A. G., Cassettari, L., Mosca, M.
2009. Risk analysis for industrial plants projects: an
innovative approach based on simulation techniques
with experimental error control. Proceedings of the
European Modelling and Simulation Symposium
(EMSS’09).
ASystemDynamicsStudyofanEmergencyDepartmentImpactontheManagementofHospital'sSurgeryActivities
603