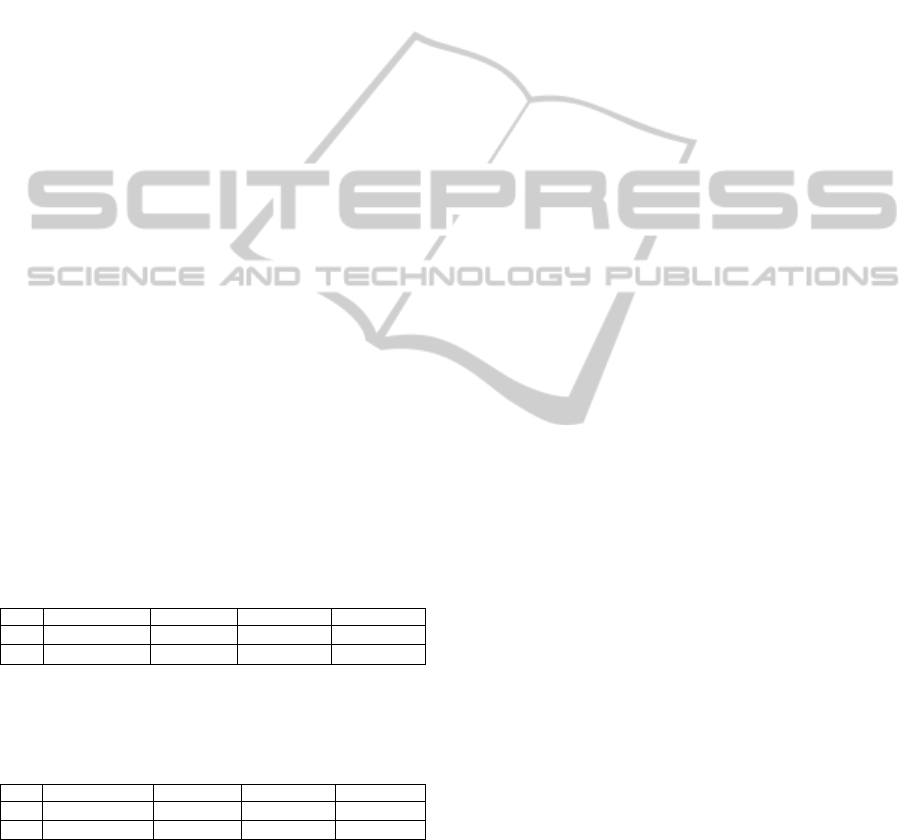
4 RESULTS AND DISCUSSION
The MSE and MAE obtained by ANFIS and FIR
models, for both HL and CL output variables are
summarized in tables 1 and 2, respectively. In both
tables, the prediction results reported in (Tsanas and
Xifara, 2012) for the Iteratively Reweighted Least
Squares (IRLS) and Random Forest (RF) algorithms
are also included in order to study their performance
when compared with fuzzy approaches. IRLS is a
linear regression algorithm that adjusts weights in
the coefficients of the classical regression scheme in
order to diminish the effect of the outliers when
obtaining the fitting curve (Bishop, 2007). RF is a
non-linear method which was first put forward by
Breiman (2001). RF is a set of classification and
regression trees, where the training sample set for a
base classifier is constructed by using the Bagging
algorithm (Breiman, 1996). When building a base
classifier, inner nodes are spitted with a random
candidate attribute set. The final classification rule
or regression function is the simple majority voting
method or the simple average method.
In tables 1 and 2 the errors of ANFIS and FIR
models over the 10 cross validation realisations were
averaged. Tsanas and Xifara performed 100 cross
validations for both, IRLS and RF models. Tables 1
and 2 show the average errors of these 100 CV. We
found out that the models errors for each realisation
were very similar and, therefore, we think that 10
CV are enough to ensure a fair comparison.
Table 1: Mean square prediction errors obtained by the
methodologies: IRLS, RF, ANFIS and FIR, for the HL
models and the CL models. The results are given in the
form of mean ± standard deviation.
MSE IRLS RF ANFIS FIR
HL
9.87±2.41 1.03±0.54 0.49±0.1 0.24±0.07
CL
11.46±3.63 6.59±1.56 3.04±0.62 2.96±0.73
Table 2: Mean absolute prediction errors obtained by the
methodologies: IRLS, RF, ANFIS and FIR, for the HL
models and the CL models. The results are given in the
form of mean ± standard deviation.
MAE IRLS RF ANFIS FIR
HL
2.14±0.24 0.51±0.11 0.52±0.05 0.35±0.04
CL
2.21±0.28 1.42±0.25 1.06±0.11 1.09±0.16
From tables 1 and 2 it can be seen that the linear
regression approach, IRLS, has the lowest
performance. All the non-linear approaches have
good results and FIR is the one that performs much
better for both outputs. It is interesting to notice that
FIR mean square errors are a 75% and 50% lower
than the errors obtained by the RF, for HL and CL
models, respectively. The ANFIS errors are also
significantly lower (50%) than the MSE of the RF
models. Therefore, both fuzzy approaches
outperform the RF in the application at hand. It is
relevant to mention that the standard deviations
obtained by ANFIS and FIR models are really much
lower than the ones obtained by RF models. A low
standard deviation indicates that all the predictions
errors (100 as described in the previous section) tend
to be very close to the mean.
An important issue is that FIR, which is the
methodology that has a better performance, is the
only one that performs a feature selection process.
FIR finds that two of the eight input variables, i.e.
relative compactness (RC) and glazing area (GA),
are highly causally related to the outputs, and
therefore, FIR models only use these two building
characteristics to predict the heating and cooling
loads. This is a very interesting result because, in the
one hand, is consistent with Tsanas and Xifara
outcomes that claim that the GA is the most
important predictor for both HL and CL.
On the other hand, it allows concluding that the
rest of the six variables, i.e. surface area (SA), wall
area (WA), roof area (RA), overall height (OH),
orientation (O), and glazing area distribution (GAD),
are redundant or irrelevant. Again, this is consistent
with the previous work that infer that variables RC,
SA, WA, RA and OH appear reasonably strongly
associated with the output variables, and at the same
time founds that some input variables are highly
correlated. Based on the FIR feature selection
process, it becomes reasonably to think that the
relative compactness variable, RC, includes the
information of other relevant variables involved in
the study, as SA or RA. In fact, this is true because
there is an analytic formula linking the RC the SA
and the volume (Tsanas and Xifara, 2012). The WA
variable is clearly directly related to the GA, so it is
redundant. Therefore, the five variables that appear
reasonably strongly associated with the output
variables contain redundant information if SA and
GA are already selected.
Figure 3 shows real versus predicted ANFIS and
FIR results for HL and CL models. In both cases we
present the fold that gives larger MSE, in order to
show that even for the worse prediction results the
difference with the real data is almost
indistinguishable, especially in the case of the
heating load model.
FuzzyApproachesImprovePredictionsofEnergyPerformanceofBuildings
509