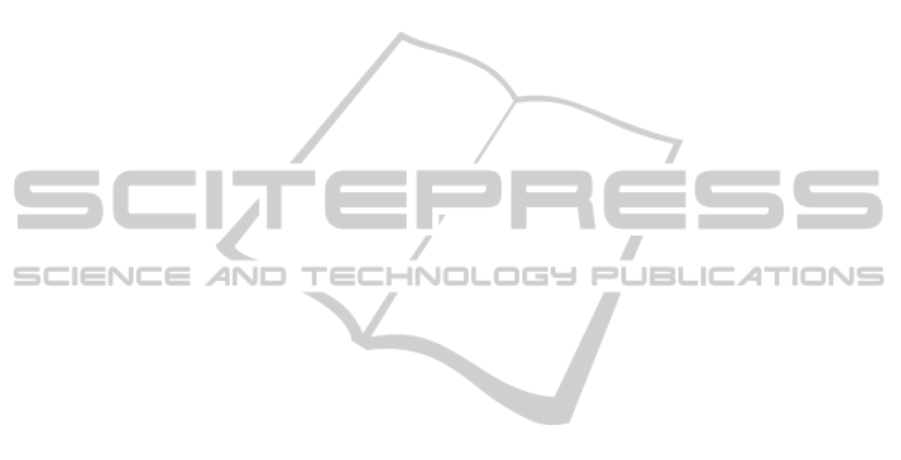
Sensitivity analysis can be applied to gain more
insight in the effects of (combinations of) parameters
on the outcome.
The ABM approach seems suitable to investigate
interdependency between social and technical
networks. It allows to observe unforeseen (possibly
unwanted) effects arising from this interdependency
and certain policy interventions. It also allows to
investigate impact on society. The java-based toolkit
Repast Simphony is flexible for this purpose, and
allows for connections with other programming
languages, which is useful for embedding a specific
technical model into a social/behavioral ABM.
Our case highlights the need for a multi-
disciplinary approach to using ABM for socio-
technical networks. Each research domain has its
own ontology which typically does not readily
combine with that from other domains (van Dam,
Nikolic, and Lukszo, 2012). For example, the
concepts and entities generally included in system
models of technical electricity grids are
incompatible with social psychological models of
human behavior. And yet we attempt to combine
both ontologies in a single ABM.
An essential next step to take is validation of a
socio-technical network. Because we need to make
many simplifications (compared to reality) in both
the social and the technical network model, the
question is whether the combined model actually fits
reality reasonably well. If unforeseen events arise
from the socio-technical model, one would like to
know if these events are plausible in reality or come
from an oversimplification or wrong specification of
the model. It should be stressed that our aim is not to
create perfect accurate predictive models at this
phase; instead we would like to use our models to
generate the types of network behaviors arising from
the interdependency between the social and
technical systems based on the characteristics of the
different networks and on potential policy
interventions. In the smart grid case, the setting is
futuristic, therefore we had to use fictional data and
could not directly validate the complete system,
though we need to take further steps in this
direction.
Another relevant research direction is balancing
the level of required detail or complexity in both the
social and the technical network models, in order to
make them fit together best.
For both themes our future research focuses on
obtaining guidelines that are as generic as possible,
i.e. that should be applicable also to other socio-
technical networks. We aim to obtain these goals
through the study of different use cases, like
residential choice models and financial networks.
REFERENCES
Ajzen, I., 1991. The theory of planned behaviour,
Organizational Behavior and Human Decision
Processes, 50(2): 179–211.
Ajzen, I. and Fishbein, M., 1980. Understanding attitudes
and predicting social behavior. Englewood Cliffs, NJ:
Prentice-Hall.
Baarsma, B., Berkhout, P., Hop, P., 2004. Op prijs gesteld,
maar ook op kwaliteit. Technisch rapport, SEO,
Amsterdam.
Bagozzi, R. P., 1992. The Self-Regulation of Attitudes,
Intentions, and Behavior, Social Psychology
Quarterly, 55(2): 178–204.
Benenson, I., 2004. Agent-based modeling: From
individual residential choice to urban residential
dynamics, in Spatially Integrated Social Science:
Examples in Best Practice, Oxford Univ. Press,
Oxford, U.K.
Boin, A., McConnell, A., 2007. Preparing for Critical
Infrastructure Breakdowns: The Limits of Crisis
Management and the Need for Resilience. Journal of
Contingencies and Crisis Management, 15(1): 50–59.
Bruch, E., Mare D., 2012. Residential Mobility, and
Neighborhood Change. Sociological Methodology, 42:
103-154.
Clastres, C., 2011. Smart Grids: Another step towards
competition, energy security and climate change
objectives. Energy Policy 39(9), 5399–5408.
Dam, K. van, Nikolic, I., Lukszo, Z., 2012. Agent-based
modelling of socio-technical systems. Springer:
Dordrecht, Netherlands.
Devisch O., Timmermans H., Arentze T., Borgers A.,
2009. An agent-based model of residential choice
dynamics in nonstationary housing markets.
Environment and Planning A, 41(8): 1997 – 2013.
Erlingsson, E., Raberto, M., Stefánsson, H., Sturluson, J.,
2013. Integrating the housing market into an agent-
based economic model. Managing Market Complexity,
Lecture Notes in Economics and Mathematical
Systems 662: 65-76.
Fishbein, M., Ajzen, I., 1975. Belief, attitude, intention,
and behavior: An introduction to theory and research.
Reading, MA: Addison-Wesley.
Gottwalt, S., Ketter, W., Block, C., Collins, J., Weinhardt,
C., 2011. Demand side management - A simulation of
household behavior under variable prices. Energy
Policy, 39: 8163-8174.
Guo, Y., Li, R., Poulton, G., Zeman, A., 2008. A
Simulator for Self-Adaptive Energy Demand
Management. In IEEE Conf. on Self-Adaptive and
Self-Organizing Systems.
Holling, C., 1973. Resilience and stability of ecological
systems. Annual Review of Ecological Systems 4:1–23.
Jackson, M., 2008. Social and Economic Networks
(Economics, Physics, Sociology), Princeton Univ.
Press.
Johnsen, S., Veen, M., 2013. Risk assessment and
resilience of critical communication infrastructure in
SIMULTECH2013-3rdInternationalConferenceonSimulationandModelingMethodologies,Technologiesand
Applications
316