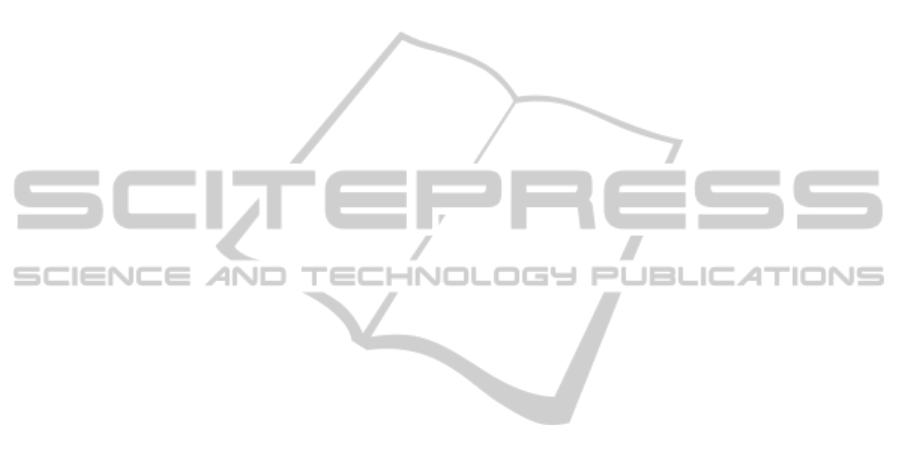
engineering phases, using a consistent information
model.
In order to cover a wide spectrum of different
domains, the following three goals were regarded as
equally important: 1. Applicability on factory
automation machinery and its operation.
2. Extensibility and upgradeability in subsequent
usage scenarios. 3. Portability and applicability to
different domains or proprietary software tools.
Consequently, OntoENERGY has been realized
as a universal lightweight upper ontology, allowing
for individual adaptations while providing all
necessary means for deploying it right out of the
box. It can be easily integrated into future software
tools and methodologies. The applicability of
OntoENERGY to a process has been demonstrated
on a real-life manufacturing domain test bed. Further
steps will include the linking to third-party software
tools and adaptations to specific domain
requirements.
REFERENCES
Bechhofer, S., Van Harmelen, F., Hendler, J., Horrocks, I.,
McGuiness, D. L., Patel-Schneider, P. F., Stein, L. A.,
2004: OWL Web Ontology Language Reference. URL
http://www.w3.org/TR/2004/REC-owl-ref-20040210/.
– accessed 19.04.2013.
Borst, P., Akkermans, J.M., Pos, A., Top, J., 1995. The
PhysSys ontology for physical systems. In Working
Papers of the Ninth International Workshop on
Qualitative Reasoning QR, pp. 11–21.
Carlisle, D., Ion, P., Miner, R., 2010. Mathematical
Markup Language (MathML) Version 3.0. URL
http://www.w3.org/TR/MathML3/. - accessed
23.04.2013.
Chryssolouris, G., Mavrikios, D., Papakostas, N.,
Mourtzis, D., Michalos, G., Georgoulias, K., 2009.
Digital manufacturing: history, perspectives, and
outlook. In Proceedings of the Institution of
Mechanical Engineers, Part B: Journal of
Engineering Manufacture. Vol. 223, pp. 451–462.
DECHEMA e.V., 2009. Chemieanlagen: ‚Operational
Excellence‘ ist das Ziel: Trendbericht Nr. 3:
Chemieanlagen-Konzepte.
Dietmair, A., Verl, A., 2008. Energy Consumption
Modeling and Optimization for Production Machines.
In Proceedings “International Conference on
Sustainable Energy Technologies (ICSET)”, pp. 574-
579.
European Parliament, 2006. Directive 2006/32/EC on
energy end-use efficiency and energy services and
repealing Council Directive 93/76/EEC.
ISO, 2006. ISO 18629: Industrial automation systems and
integration - Process specification language - Part 1:
Overview and basic principles.
NASA, 2012. Semantic Web for Earth and Environmental
Terminology (SWEET). URL http://sweet.jpl.nasa.gov.
- accessed 21.04.2013.
NASA, 2006. Semantic Web for Earth and Environmental
Terminology (SWEET) V1.1: units.owl. URL
http://sweet.jpl.nasa.gov/1.1/units.owl. - accessed
21.04.2013.
Niles, I., and Pease, A., 2001. Towards a Standard Upper
Ontology. In Proceedings of the 2nd International
Conference on Formal Ontology in Information
Systems (FOIS-2001). Ogunquit, Maine, October 17-
19, 2001.
Rudolph, M. and Wagner, U., 2008.
Energieanwendungstechnik. Wege und Möglichkeiten
zur Optimierung der Energietechnik. Springer. Berlin.
Berlin 2008
Sauer, O., 2010. Trends in manufacturing execution
systems. In Proceedings of the 6th CIRP-Sponsored
International Conference on Digital Enterprise
Technology, pp. 685–693.
VDI, 2003. VDI-Directive 4661: Energetic characteristics
Definitions – terms – methodology.
Wicaksono, H., Rogalski, S., Ovtcharova, J., 2012.
Ontology Driven Approach for Intelligent Energy
Management in Discrete Manufacturing. In
Proceedings of 4th International Conference on
Knowledge Engineering and Ontology Development.
Barcelona, Spain, pp. 108-114.
World Wide Web Consortium (W3C), 2006. Defining N-
ary Relations on the Semantic Web. URL
http://www.w3.org/TR/swbp-n-aryRelations/. -
accessed 05.03.2013.
Verl, A., Westkämper, E., Abele, E., Dietmair, A.,
Schlechtendahl, J. F.: Architecture for Multilevel
Monitoring and Control of Energy Consumption. In
Hesselbach, J., Hermann, C. (ed.): Glocalized
Solutions for Sustainability in Manufacturing.
Proceedings of the 18th CIRP International
Conference on Life Cycle Engineering, Technische
Universität Braunschweig, Braunschweig, Germany,
May 2nd - 4th, 2011, pp. 347-352.
Zeiler, W., van Houten, R., Boxem, G., Savanovic, P., van
der Velden, J., Wortel, W., Haan, J.-F., Kamphuis, R.,
Hommelberg, M., Broekhuizen, H., 2009. Flexergy.
An ontology to couple decentralised sustainable
comfort systems with centralized energy
infrastructure. In Proceedings of 3rd International
Conference on Smart and Sustainable Built
Environments (SASBE).
KEOD2013-InternationalConferenceonKnowledgeEngineeringandOntologyDevelopment
344