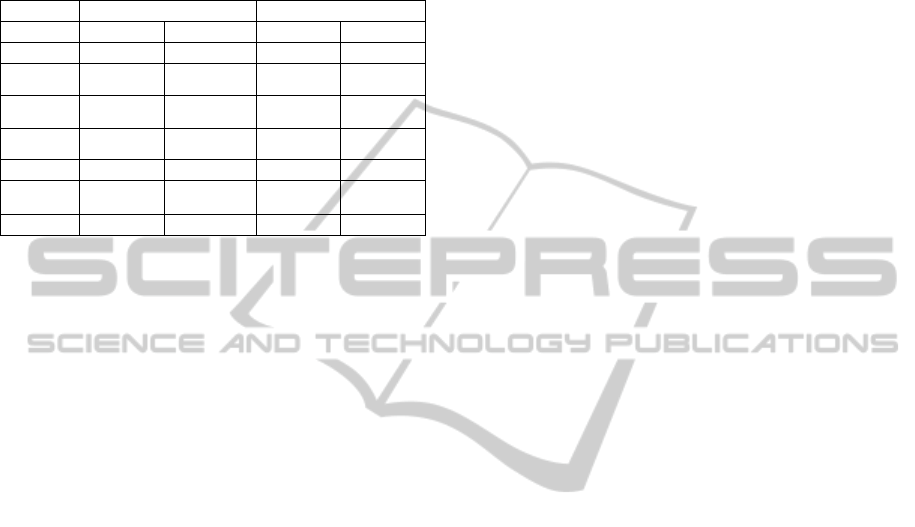
with assistance, the conditions changed as the
patient has now an extra support that helps in
alleviating pain. By this SW values do not differ
significantly from the healthy subjects.
Table 2: Spatiotemporal parameters of walker-assisted
gait. Average±Standard Deviation values.
Subj.
PT HI
Direction Forward Curve Forward Curve
G (s) 1,52±0,160 1,51±0,256 1,51±0,028 1,63±0,057
ST (%) 58,06±6,535 56,72±7,395 55,33±2,350 58,89±1,922
SW (%) 41,92±6,531 43,24±7,388 44,67±2,354 41,11±1,922
SL (m) 0,22±0,046 0,21±0,032 0,37±0,062 0,31±0,0455
vh (m/s) 0,32±0,158 0,3±0,147 0,44±0,061 0,39±0,047
CAD
(step/min)
79,54±7,895 81,01±13,146 79,13±0,01 73,15±2,547
d (m) 0,44±0,052 0,46±0,053 0,55±0,067 0,53±0,051
3.2 Human-walker Interaction
Parameters
In the ‘ψ Angle’ graph of Figure 7, one can see that
the IMU’s signals provide information about the PT
movement. He is going in straight line and then at
t=1s, he begins to make a curve. Then, at t=3s, he
goes again straight and makes a curve, at t=5s, for
the other side until t=8s. From t=8s to t=10s, he
continues to walk forward and straight.
The ‘Angular Velocity’ graph (Figure 7)
indicates that he increases (in absolute) its angular
velocity (wh) when he starts to curve, by analyzing
the same instants of time as previously.
Therefore, these two parameters can be used to
correctly detect the path that the user is following. In
‘Legs Distance’ graph (Figure 7), one can see that is
hard to distinguish between going forward and
making a curve. However, it can be noticed that
maximum values of right leg are reduced when PT
makes the first curve (t=1s to t=3s). However, this
change is not perceptible or significant in the second
curve.
After observing ‘Legs Distance’ signals from all
the patients, it was concluded that there is a great
variability on this signal. Which means that PTs can
perform a curve in different manners: some hide one
leg; others fend off the legs, or bring them together.
‘Legs Orientation’ (Figure 7) also presents small
changes during the time PT is performing a curve
(t=[3 4]s and t=[5 8] s). Once again, this signal
presents a great variability through PTs.
A possible solution to increase the effects of
making a curve on the LRF signal would be to put
the LRF up to the foot’s height, to detect their
direction. However, this is not possible to detect
with LRF sensor, because the signal becomes
distorted and poor of information. So, the utilization
of a camera, for example, could be a good solution
to detect the feet’s direction.
Thus, LRF sensor is good to detect
spatiotemporal parameters, as it was analyzed
before, but not too good to detect intention of
changing direction.
Moreover, LRF sensor is essential to detect when
legs are crossing with each other (identified by
circles on the graphs). This is an important event to
detect BCP position, since in these instants it is the
midpoint between the legs.
So, Human-Walker Interaction parameters can be
calculated every time the legs cross and are
represented in Figure 7.Distance between the user
and the walker (d) is acquired by the ‘Legs
Distance’ signal and it is marked with circles. Angle
of BCP orientation in relation to the walker (θ) is
acquired by the ‘Legs Orientation’ signal, being the
midpoint between each leg orientation, and is
represented in ‘θ and ϕ’ graph. Angle between linear
velocity vector and human-walker interaction line
(ϕ) is calculated by the sum of ϕ angle of walker and
ψ angle of human, both represented in ‘ψ Angle’
graph and θ. This angle is represented in ‘θ and ϕ’
graph by the designated signal ‘ϕ’. Angular velocity
of the user (wh) are the points marked with a circle
in the ‘Angular Velocity’ graph . Linear velocity of
the user (vh) depends on the time that the user takes
to complete a stride (two steps) and is shown in
‘Human Linear Velocity’ graph.
Looking at ‘Human Linear Velocity’ graph
(Figure 7), one can see that vh decreases when
making a curve, which is in accordance with
previous discussion.
Through ‘Human and Walker Orientation (ψ)’
(Figure 7), one can see that the walker turns first
than the human. This could indicate that the
intention of command is transmitted by the upper
limbs. This needs to be further studied by placing a
rotating handlebar with integrated IMU or force
sensors.
In ‘θ and ϕ’ graph (Figure 7), one can see that ϕ
is better to identify, with significant variability, the
orientation of the subject when compared with θ.
In conclusion, the Human-Walker Interaction
parameters, in the overall are correctly detected and
can describe the interaction between the PT and the
walker.
AssessmentofWalker-assistedHumanInteractionfromLRFandWearableWirelessInertialSensors
149