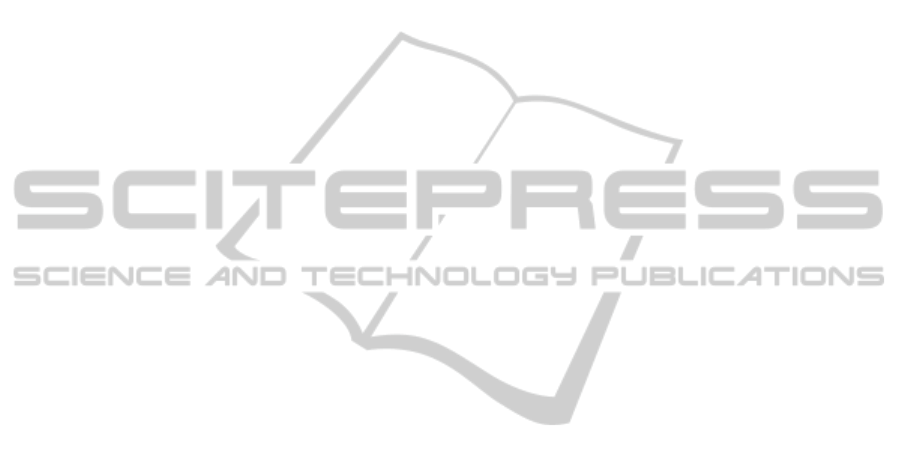
risk mitigation and failure avoidance.
The considerations presented in this paper are a
good basis, but requesting further research. First of
all it is inevitable to develop a conversion tool for
preservation. This tool is supposed to take sensor
and contextual information as input and convert them
into a preservable format. Of course, it is necessary
to check whether the preserved data contains all rel-
evant information that was given by the input data.
The preserved data will be replayed some time later
on. To perform this action a replaying algorithm is
required. This algorithm has to be examined regard-
ing its replay accuracy and usefulness. It is important
to check whether the replayed data behaves like the
original data did. As mentioned before in Subsection
4 there are additional possibilities to work with pre-
served data in the future than just replay it. There is
also the option to use the preserved, historic data to
predict future behaviour, e.g. by performing stream
mining algorithms. Especially in the framework of
civil engineering, where the focus is often on enhanc-
ing the safety and reducing a risk by avoiding failures,
the early detection errors or uncommon behaviour is
important. This can be achieved for example by learn-
ing a model by applying machine learning techniques
on the data. It is considerable to simulate emergency
situations, e.g. the bursting or the failure of a water
dam, by altering some parameters. The task for the
developed system is to detect this anomaly as soon as
possible to raise an alarm. The question is whether
the system is able to detect such a failure in time.
ACKNOWLEDGEMENTS
The authors would like to acknowledge the funding
by the European Commission under the ICT project
“TIMBUS” (Project No. 269940, FP7-ICT-2009-6)
within the 7th Framework Programme. This work
was also supported by national funds through FCT
Fundac¸
˜
ao para a Ci
ˆ
encia e a Tecnologia, under project
PEst-OE/EEI/LA0021/2013.
REFERENCES
American Society of Civil Engineers (2000). Guidelines
for instrumentation and measurements for monitoring
dam performance.
Becker, C., Kolar, G., Kueng, J., and Rauber, A. (2007).
Preserving Interactive Multimedia Art: A Case Study
in Preservation Planning. In Proceedings of the
10th International Conference on Asian Digital Li-
braries, ICADL’07, pages 257–266, Berlin, Heidel-
berg. Springer-Verlag.
Bloechle, J. L., Rigamonti, M., Hadjar, K., Lalanne, D.,
and Ingolf, R. (2006). XCDF: A Canonical and Struc-
tured Data Format. Document Analysis Systems VII,
3972:141–152.
Borghoff, U. M., R
¨
odig, P., and Scheffczyk, J. (2006).
Long-Term Preservation of Digital Documents, Prin-
ciples and Practices. Springer Berlin Heidelberg.
Chou, C., Dappert, A., Delve, J., and Peyrard, S. (2012).
Describing Digital Object Environments in PREMIS.
In Proceedings of the 9th International Conference on
Preservation of Digital Objects, iPRES 2012.
Cohen, N., Ourakayashta, A., Turek, J., Wong, L., and Yeh,
D. (2001). Challenges in Flexible Aggregation of Per-
vasive Data.
Conway, P. (1996). Archival Preservation in a Nationwide
Context. 2:204–222.
Day, M. (2001). Metadata for Digital Preservation: A Re-
view of Recent Developments. In Proceedings of the
5th European Conference on Research and Advanced
Technology for Digital Libraries, ECDL ’01, pages
161–172, London, UK, UK. Springer-Verlag.
Faber, M. H. (2007). Risk and safety in civil engineering.
Galushka, M., Taylor, P., Gilani, W., Thomson, J., Strodl,
S., and Neumann, M. A. (2012). Digital Preservation
Of Business Processes with TIMBUS Architecture. In
Proceedings of the 9th International Conference on
Preservation of Digital Objects, iPRES 2012.
Giaretta, D. (2011). Preservation of intelligibilityintelligi-
bility of digital objects. pages 39–166.
Guttenbrunner, M., Becker, C., and Rauber, A. (2010).
Keeping the Game Alive: Evaluating Strategies for the
Preservation of Console Video Games. International
Journal of Digital Curation, 5(1).
International Commission on Large Dams (1999). Guide-
lines for automated dam monitoring systems.
Kutter, B., Wilson, D., and Bardet, J. (2002). Metadata
Structure for Geotechnical Physical Models (and Sim-
ulations?). Technical report.
Lee, K.-H., Slattery, O., Lu, R., Tang, X., and McCrary,
V. (2002). The state of the art and practice in digital
preservation. Journal of Research-National Institute
of Standards and Technology, 107(1):93–106.
McHugh, A., Konstantelos, L., and Barr, M. (2010). Re-
flections on Preserving the State of New Media Art.
In Proceedings of the Archiving Conference.
Neumann, M. A., Miri, H., Thomson, J., Antunes, G.,
Mayer, R., and Beigl, M. (2012). Towards a Deci-
sion Support Architecture for Digital Preservation of
Business Processes. In Proceedings of the 9th Interna-
tional Conference on Preservation of Digital Objects,
iPRES 2012.
Roure, D. D., Belhajjame, K., Missier, P., Gmez-Prez, J. M.,
Palma, R., Ruiz, J. E., Hettne, K., Roos, M., Klyne,
G., and Goble, C. (2011). Towards the Preservation
of Scientific Workflows. In Proceedings of the 8th
International Conference on Preservation of Digital
Objects, iPRES 2011.
van der Hoeven, J., Lohman, B., and Verdegem, R. (2007).
Emulation for Digital Preservation in Practice: The
Results. 2:123–132.
Wieland, M. and Mueller, R. (2009). Dam safety, emer-
gency action plans and water alarm systems.
PreservationandRedeploymentofSensorAcquisitionProcessesfromaDamSafetyInformationSystem
495