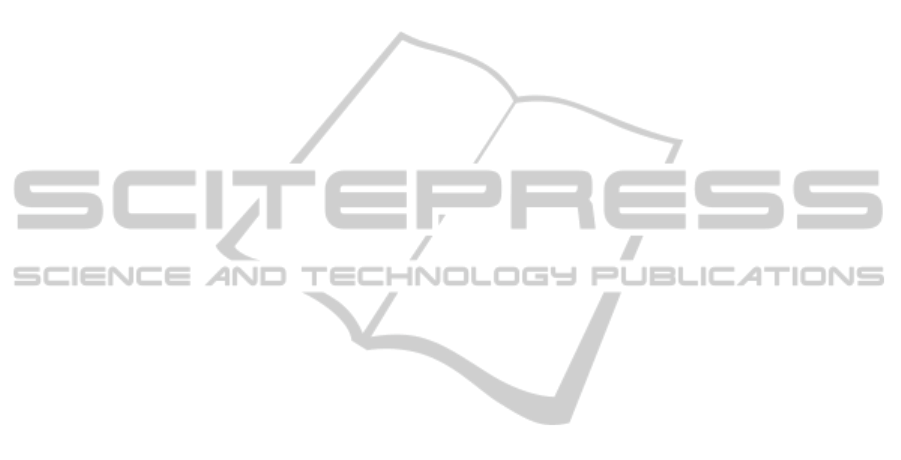
the Human-Robot interaction during the experiment:
Human [pointing the unseen white teddy-bear]:
“Describe this!”
Robot:: “It is white!”
Human [pointing the already seen, but reversed, yellow
box]: “Describe this!”
Robot: “It is yellow!”
Human [pointing the unseen apple]: “Describe this!”
Robot: “It is red!”
7 CONCLUSIONS
This paper has presented, discussed and validated a
cognitive system for high-level knowledge
acquisition based on the notion of artificial curiosity.
Driving as well the lower as the higher levels of the
presented cognitive system, the emergent artificial
curiosity allow such a system to learn in an
autonomous manner new knowledge about unknown
surrounding world and to complete (enrich or
correct) its knowledge by interacting with a human.
Experimental results, performed as well on a
simulation platform as using the NAO robot show
the pertinence of the investigated concepts as well as
the effectiveness of the designed system. Although it
is difficult to make a precise comparison due to
different experimental protocols, the results we
obtained show that our system is able to learn faster
and from significantly fewer examples, than the
most of more-or-less similar implementations.
Based on obtained results, it is thus justified to
say, that a robot endowed with such artificial
curiosity based intelligence will necessarily include
autonomous cognitive capabilities. With respect to
this, the further perspectives will focus integration of
the investigated concepts in other kinds of machines,
such as mobile robots. There, it will play the role of
an underlying system for machine cognition and
knowledge acquisition.
REFERENCES
Achanta, Estrada, F., Wils, P., Susstrunk, S., 2008. Salient
Region Detection and Segmentation, Proc. of
International Conference on Computer Vision Systems
(ICVS '08), vol. 5008, LNCS, Springer Berlin /
Heidelberg, 66_75.
Achanta, R., Hemami, S., Estrada, F., Susstrunk, S., 2009
Frequency-tuned Salient Region Detection, Proc. of
IEEE International Conference on Computer Vision
and Pattern Recognition (CVPR).
Araki, T., Nakamura, T., Nagai, T., Funakoshi, K.,
Nakano, M. and Iwahashi, N., 2011 'Autonomous
acquisition of multimodal information for online
object concept formation by robots', IEEE/RSJ
International Conference on Intelligent Robots and
Systems, San Francisco, 1540-1547.
Berlyne, D. E., 1954, 'A theory of human curiosity',
British Journal of Psychology, vol. 45, no. 3, August,
pp. 180-191.
Bowerman, M. , 1983, 'How Do Children Avoid
Constructing an Overly General Grammar in the
Absence of Feedback about What is Not a Sentence?',
Papers and Reports on Child Language Development.
Brand, R. J., Baldwin, D. A. and Ashburn, L. A., 2002
'Evidence for 'motionese': modifications in mothers
infant-directed action', Developmental Science, 72-83.
Coradeschi, S. and Saffiotti, A., 2003, 'An introduction to
the anchoring problem', Robotics and Autonomous
Systems, vol. 43, pp. 85-96.
Goodrich, M. A. and Schultz, A. C., 2007, 'Human-robot
interaction: a survey', Foundations and trends in
human computer interaction, vol. 1, jan, pp. 203-275.
Greeff, J. D., Delaunay, F. and Belpaeme, T., 2009,
'Human-Robot Interaction in Concept Acquisition: a
computational model', Proceedings of the 2009 IEEE
8th International Conference on Development and
Learning, Washington, 1-6.
Harel, J., Koch, C., Perona, P., 2007, Graph-based visual
saliency, Advances in Neural Information Processing
Systems 19, 545_552.
Itti, L., Koch, C. , Niebur, E., 1998, A Model of Saliency-
Based Visual Attention for Rapid Scene Analysis,
IEEE Transactions on Pattern Analysis and Machine
Intelligence, 20, 1254_1259.
Kang, M. J. J., Hsu, M., Krajbich, I. M., Loewenstein, G.,
McClure, S. M., Wang, J. T. T. and Camerer, C. F.,
2009, 'The wick in the candle of learning: epistemic
curiosity activates reward circuitry and enhances
memory', Psychological science, vol. 20, no. 8,
August, pp. 963-973, Available: 1467-9280.
Kuhn, D., Garcia-Mila, M., Zohar, A. and Andersen, C.,
1995, 'Strategies of knowledge acquisition', Society for
Research in Child Development Monographs, vol. 60
(4), no. 245.
Liang, Z., Chi, Z., Fu, H., Feng, D., 2012, Salient object
detection using content-sensitive hypergraph
representation and partitioning, Pattern Recogn. 45
(11), 3886_3901.
Litman, J. A., 2008, 'Interest and deprivation factors of
epistemic curiosity', Personality and Individual
Differences, vol. 44, no. 7, pp. 1585-1595.
Liu, T., Yuan, Z., Sun, J., Wang, J., Zheng, N. , Tang X.,
Shum, H.-Y., 2011, Learning to Detect a Salient
Object, IEEE Trans. Pattern Anal. Mach. Intell. 33 (2),
353_367.
Madani, K. and Sabourin, C., 2011, 'Multi-level cognitive
machine-learning based concept for human-like
"artificial" walking: Application to autonomous stroll
of humanoid robots. Neurocomputing, Vol. 74, 1213-
1228.
Madani, K., Ramik, D. M. and Sabourin, C., 2012, Multi-
level cognitive machine-learning based concept for
IJCCI2013-InternationalJointConferenceonComputationalIntelligence
418