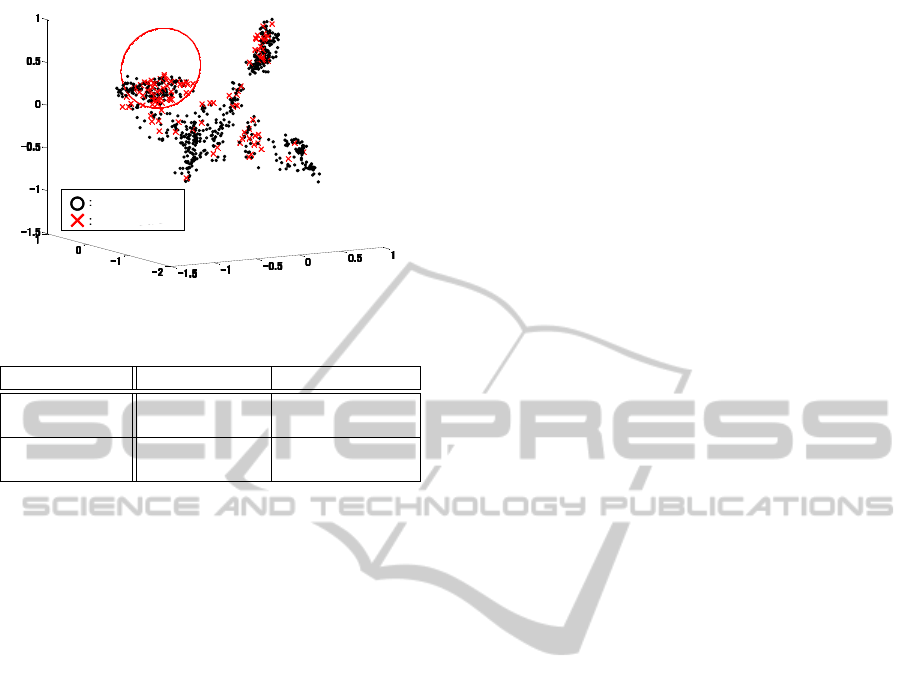
Figure 10: Mapping result with PCA.
Table 2: Discrimination of collision with PCA.
Collision [%] No collision [%]
Recognized as
collision 63 / 115 [54.8] 132 / 617 [21.4]
Recognized as
no collision 52 / 115 [45.2] 485 / 617 [78.6]
keypoints stably detected on the hand of NAO. Not
only improving reliability of image features (e.g., us-
ing PCA-SIFT (Ke and Sukthankar, 2004)) but also
applying multiple kinds of features will be important
to generate good data matrix.
Mismatching of keypoints is substantially in-
evitable when a part of the robot changes its posture.
Therefore, it will be important to expand the frame-
work to a more flexible one, which can continuously
map a vector whose elements are partly lost.
6 CONCLUSIONS
In this paper, a manifold learning method was tested
for bottom-up acquisition of a space which is useful
for motion generation of a robot. This approach does
not require any specific knowledge on the robot and
its environment, which will contribute to development
of truly flexible intelligence of autonomous robots.
In the evaluation of simulated image vectors, it
was verified that the distance between the robot hand
and the object was reflected in the map. In the evalu-
ation of experiment with real images, the robot could
classify images whether the robot is colliding with
the object based on the obtained mapping. Moreover,
manifold learning turned out to be superior to linear
dimensionality reduction, PCA. As a next step, it will
be required to extend the idea of bottom-up construc-
tion of a low-dimensional space to the case where fea-
tures frequently disappears.
ACKNOWLEDGEMENTS
This work was partly supported by Kayamori Foun-
dation of Informational Science Advancement.
REFERENCES
Aldebaran Robotics (2009). Nao. http://www.aldebaran-
robotics.com/. Technical Specifications Document.
Argall, B. D., Chernova, S., Veloso, M., and Browning, B.
(2009). A survey of robot learning from demonstra-
tion. Robot. Auton. Syst., 57(5):469–483.
Ke, Y. and Sukthankar, R. (2004). Pca-sift: A more distinc-
tive representation for local image descriptors. Com-
puter Vision and Pattern Recognition.
Kobayashi, Y., Okamoto, T., and Onishi, M. (2012). Gen-
eration of obstacle avoidance based on image features
and embodiment. International Journal of Robotics
and Automation, 24(4):364–376.
Kohonen, T. (1995). Self-Organizing Maps. Springer Press.
Lowe, D. G. (1999). Object recognition from local scale-
invariant features. In Proc. of IEEE International Con-
ference on Computer Vision, volume 2, pages 1150–
1157.
Lungarella, M., Metta, G., Pfeifer, R., and Sandini, G.
(2003). Developmental robotics: a survey. Connec-
tion Science, 15:151–190.
Morimoto, J., Nakanishi, J., Endo, G., Cheng, G., Atke-
son, C. G., and Zeglin, G. (2005). Poincar
´
e-Map-
Based Reinforcement Learning For Biped Walking. In
Proc. of IEEE International Conference on Robotics
and Automation.
Oudeyer, P. Y., Kaplan, F., and Hafner, V. (2007). Intrinsic
motivation systems for autonomous mental develop-
ment. IEEE Transactions on Evolutionary Computa-
tion, 11(2):265–286.
Prankl, J., Zillich, M., and Vincze, M. (2011). 3d piece-
wise planar object model for robotics manipulation.
In Robotics and Automation (ICRA), 2011 IEEE In-
ternational Conference on, pages 1784 –1790.
Saul, L. K. and Roweis, S. T. (2003). Think globally,fit
locally : Unsupervised learning of low dimensional
manifolds. Journal of Machine Learning Research,
4:119–155.
Stoytchev, A. (2009). Some basic principles of develop-
mental robotics. IEEE Transactions on Autonomous
Mental Development, 1(2):122–130.
Sutton, R. S. and Barto, A. G. (1998). Reinforcement Learn-
ing: An Introduction (Adaptive Computation and Ma-
chine Learning). A Bradford Book.
Weng, J., McClelland, J., Pentland, A., Sporns, O., Stock-
man, I., Sur, M., and Thelen, E. (2001). Autonomous
mental development by robots and animals. Science,
291:599–600.
IJCCI2013-InternationalJointConferenceonComputationalIntelligence
534