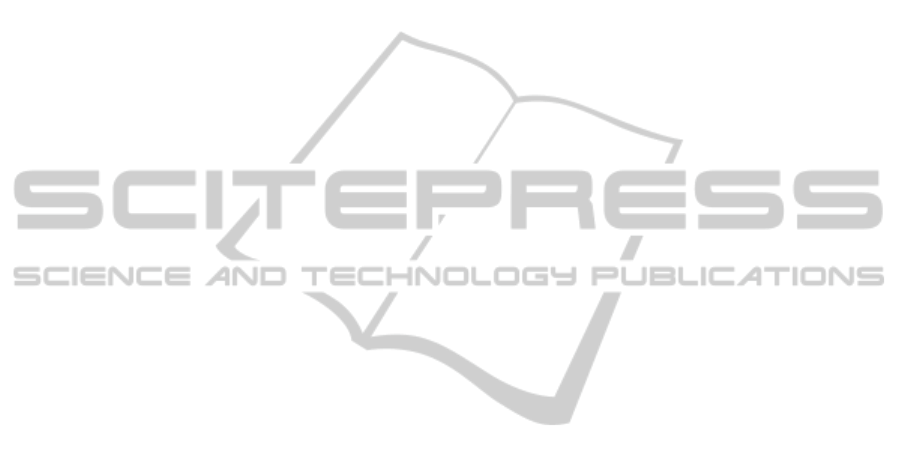
types of trends, for example rising, falling and cyclic
patterns. SQL-queries and data visualization will help
achieve the following:
• Trace the observed quality of evidence sources
based on the history of source impact values.
• Monitor the quality of the ontology learning sys-
tem itself via the ratio of relevant to irrelevant con-
cept candidates.
• Investigate which sources suggest which con-
cepts, and shifts between sources.
• Examine aggregated (eg. all text or all social ev-
idence sources) patterns, or comparisons across
domains.
6 CONCLUSIONS
This position paper presents the enhancements to an
existing ontology learning system – adding novel fea-
tures to automate the ontology learning cycle as far as
possible. These features allow for a wide range of on-
tology evolution experiments which reflect and detect
data-driven change in the domain.
The main contributions of the paper are (i) provid-
ing a model which supplies a high level of automation
for learning and evolving lightweight ontologies, (ii)
describing a prototype which implements this model
as a Web service, including the administration inter-
face and parameters, (iii) presenting trend and pattern
detection experiments facilitated by the automated ar-
chitecture and the database that collects fine-grained
data about ontological elements over time.
Future work includes the completion of a more
powerful evaluation framework which performs eval-
uation tasks either with (refined) GWAPs or delegates
them to CrowdFlower. The new evaluation frame-
work is under development. Furthermore, after col-
lecting longitudinal data, we will conduct and extend
the ontology evolution experiments described in Sec-
tion 5.
ACKNOWLEDGEMENTS
The presented work was developed within DIVINE
(www.weblyzard.com/divine), a project funded by the
Austrian Ministry of Transport, Innovation & Tech-
nology (BMVIT) and the Austrian Research Pro-
motion Agency (FFG) within FIT-IT (www.ffg.at/fit-
it). The work has also been supported by uComp
(www.ucomp.eu), a project in EU’s ERA-NET
CHIST-ERA programme.
REFERENCES
Cimiano, P., Maedche, A., Staab, S., and Voelker, J. (2009).
Ontology learning. In Staab, S. and Rudi Studer,
D., editors, Handbook on Ontologies, International
Handbooks on Information Systems, pages 245–267.
Springer Berlin Heidelberg.
Cimiano, P., Pivk, A., Schmidt-Thieme, L., and Staab,
S. (2005). Ontology Learning from Text, chapter
Learning Taxonomic Relations from Heterogeneous
Sources of Evidence, pages 59–76. IOS Press, Am-
sterdam.
Fellbaum, C. (1998). Wordnet an electronic lexical
database. Computational Linguistics, 25(2):292–296.
Haase, P. and Stojanovic, L. (2005). Consistent evolution
of owl ontologies. In Proceedings of the Second Eu-
ropean Semantic Web Conference, Heraklion, Greece,
pages 182–197.
Harris, Z. S. (1968). Mathematical Structures of Language.
Wiley, New York, NY, USA.
Havasi, C., Borovoy, R., Kizelshteyn, B., Ypodimatopou-
los, P., Ferguson, J., Holtzman, H., Lippman, A.,
Schultz, D., Blackshaw, M., and Elliott, G. T. (2012).
The glass infrastructure: Using common sense to cre-
ate a dynamic, place-based social information system.
AI Magazine, 33(2):91–102.
Liu, W., Weichselbraun, A., Scharl, A., and Chang, E.
(2005). Semi-automatic ontology extension using
spreading activation. Journal of Universal Knowledge
Management, 0(1):50–58.
M
¨
adche, A. and Staab, S. (2001). Ontology learning for the
semantic web. IEEE Intelligent Systems, 16(2):72–79.
Maynard, D. and Aswani, N. (2010). Bottom-up Evolution
of Networked Ontologies from Metadata (NeOn De-
liverable D1.5.4).
Natasha F. Noy, Jonathan Mortensen, P. A. and Musen, M.
(2013). Mechanical turk as an ontology engineer?
In Proceedings of the ACM Web Science 2013 (Web-
Sci’13), Paris, Forthcoming.
Scharl, A., Sabou, M., and F
¨
ols, M. (2012). Climate quiz:
a web application for eliciting and validating knowl-
edge from social networks. In Bressan, G., Silveira,
R. M., Munson, E. V., Santanch
`
a, A., and da Grac¸a
Campos Pimentel, M., editors, WebMedia, pages 189–
192. ACM.
Siorpaes, K. and Hepp, M. (2008). OntoGame: Weaving
the semantic web by online games. In Bechhofer,
S., Hauswirth, M., Hoffmann, J., and Koubarakis,
M., editors, 5th European Semantic Web Conference
(ESWC), volume 5021, pages 751–766. Springer.
Weichselbraun, A., Wohlgenannt, G., and Scharl, A. (2010).
Refining non-taxonomic relation labels with external
structured data to support ontology learning. Data &
Knowledge Engineering, 69(8):763–778.
Wohlgenannt, G., Weichselbraun, A., Scharl, A., and
Sabou, M. (2012). Dynamic integration of multiple
evidence sources for ontology learning. Journal of In-
formation and Data Management (JIDM), 3(3):243–
254.
KEOD2013-InternationalConferenceonKnowledgeEngineeringandOntologyDevelopment
412