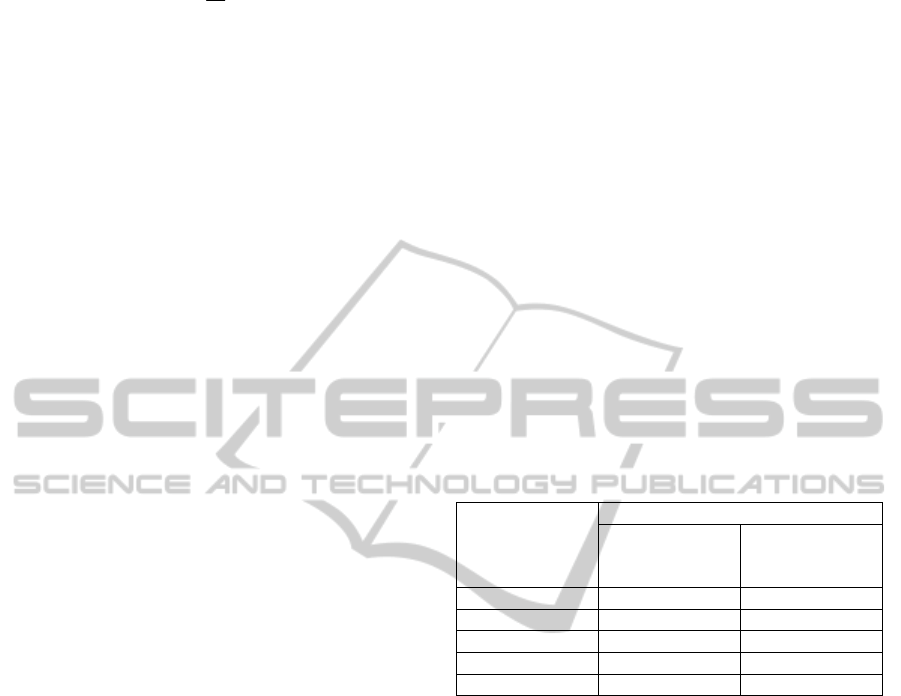
i
k
t
kj
kj
ewB
ˆ
1
ˆ
ˆ
ˆ
.
(19)
where
i
ˆ
is the number of interval currently being
analyzed;
kj
t
ˆ
is an enumerated spike,
kj
w
ˆ
is a
weight of synapse that spikes comes to soma
through. Now if one calculates
j
t according to (17)-
(19) on each time interval until a real value is
received, he can obtain spiking neuron firing time
for an arbitrary number of incoming spikes (Figure 3
illustrates case with three incoming spikes).
Thus, having analytical model of spiking neuron,
a researcher can easily implement a software
application of self-learning spiking neural network
(learning procedures of spiking neural networks are
out of scope of this paper). Under easy software
implementation, we understand the fact that a
researcher does not have to program spike
propagation form a receptive neuron or a spiking
neuron through multiple synapse to soma of the
spiking neuron whose firing time is being obtained.
In a sense, the proposed model of spiking neuron is
akin to conventional models of artificial neural
networks of the second generation as they are
constructed in terms of matrix algebra, thus allowing
developers and researcher to avoid biological aspect
of neurons operating.
An additional advantage of the proposed model
is that it can operate in a sequence mode when new
spikes constantly come to spiking neuron inputs.
However, we have to note here that spiking neuron
refractoriness and effect of spike-after potential on
further neuron firing are not considered in this work
as in any case they play no part in the most of
spiking neural networks used in actual practice.
4 SPIKING NEURON SOFTWARE
IMPLEMENTATIONS
PERFORMANCE
Nowadays software applications of the designed
models and systems are in most common use due to
their simplicity and low price as compared to
hardware implementations. This brings up an
important question on performance of different
spiking neuron software implementations.
Surprisingly, ways to improve spiking neural
network models for software implementation are
poorly researched. This section describes results of
performance testing of two spiking neuron software
implementations – straightforward model and the
model introduced in this paper based on the Lambert
W function.
The straightforward model of spiking neuron (an
example of it can be found in (De Berredo, 2005))
emulates spiking neuron membrane potential
dynamics and has to check whether its value crossed
firing threshold on each time step.
Software implementation of the spiking neuron
model introduced on the Lambert W function base
rests on the procedure described in the previous
section: incoming spikes are put in order of their
firing time magnitude and
j
t is calculated with
(17)-(18) on each time interval formed; the first real
value of
j
t indicates firing time of spiking neuron.
As seen from Table 1, the introduced model is
always faster than the straightforward model though
its operating time raises as size of input spikes
vector increases.
Table 1: Results of performance testing of straightforward
model of spiking neuron and the model proposed in this
paper.
Size of input
spikes vector
Firing time calculation speed, s
Straightforward
model
Model based on
the Lambert W
function
10 0.0019 0.0012
50 0.254 0.148
100 0.706 0.308
300 2.129 1.524
500 4.018 1.757
We have to note here that in practice, a range of
various techniques are used to improve performance
of software implementations (e.g., methods of
matrix algebra). We used just ‘pure’ models for the
sake of reference models comparison.
5 CONCLUSIONS
The major conclusion of the research is that
analytical dependence of spiking neuron firing time
on input spikes can be expressed – but in an intricate
way. That fact complicates comprehensive analysis
of spiking neural networks behavior and features.
However, the proposed spiking neuron model allows
of improving spiking neural networks software
implementations performance. It also allows a
researcher to abstract away from biological specific
of spiking neural networks when implementing them
and to use them just as a regular tool for data
processing. Another advantage of the proposed
model is its precision level of the firing time
ASpikingNeuronModelbasedontheLambertWFunction
545