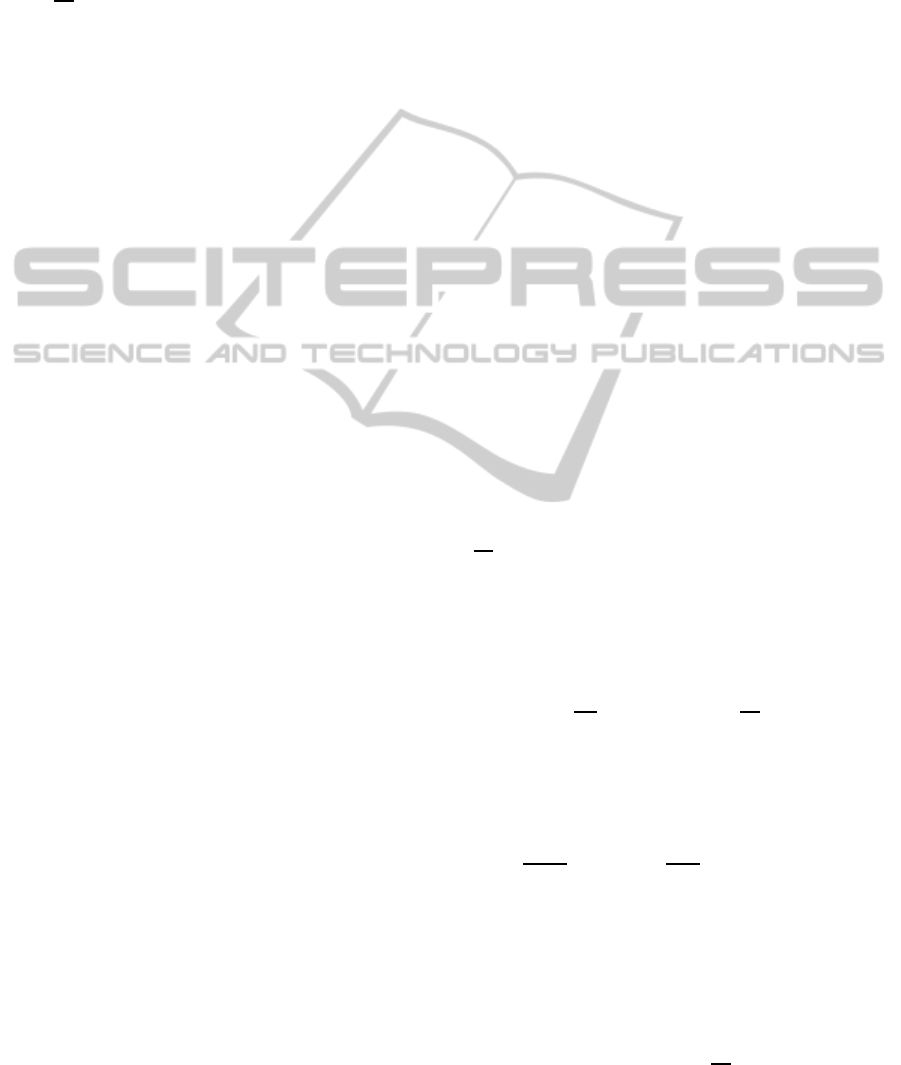
der the assumption of the classical Fick’s law for the
mass flux J
F
J
F
= −D∇c, (2)
where D is the diffusion tensor, the model can be writ-
ten as
∂c
∂t
= ∇.(D∇c) + f(c) in Ω ×(0, ∞). (3)
The mathematical model is complemented by bound-
ary conditions which impose no migration of cells be-
yond the brain boundary, that is,
J
F
.η = 0,
on the boundary, where η denotes the exterior unit
normal to the brain region, and by initial conditions
c(x,0) = c
0
(x),x ∈ Ω, where c
0
defines the initial
spatial distribution of malignant cells.
Tumor growth is generally assumed to be ex-
ponential, so that the cell growth term is given by
f(c) = ρc, where the net proliferation rate ρ is con-
stant. However, logistic and gompertzian growths
have been considered but found to be unnecessary
in the time frames considered for gliomas ((Harpold
et al., 2007)). To apply the modeling approach to spe-
cific patients, a more realistic look at the brain geome-
try and structure was necessary. Swanson et al. intro-
duced in (Swanson et al., 2000) the complex geome-
try of the brain and allowed diffusion to be a function
of the spatial variable to reflect the observation that
glioma cells exhibit higher motility in the white mat-
ter than in grey matter.
Finally we observe that the most popular treat-
ments used to combat gliomas are chemotherapy and
radiation. Mathematical models to describe the effect
of the previous treatments were proposed in the liter-
ature. Without being exhaustive we mention (Rockne
et al., 2009) and (Tracqui et al., 1995).
The partial differential equation (3), of parabolic
type,was established combing the mass conservation
law (1) with Fick’s law (2) for mass flux. It is
well known that, in this case, if a sudden change
on the cell concentration takes place somewhere in
the space, it will be felt instantaneously everywhere
this means that Fickian approach gives rise to infi-
nite speed of propagation which is not physically ob-
servable. To avoid the limitation of Fickian mod-
els an hyperbolic correction has been proposed in
different contexts (see (Edwards and Cohen, 1995),
(Joseph and Preziosi, 1989), (Fedotov, 1998), (Fedo-
tov, 1999), (Hassanizadeh, 1996), (Neuman and Tar-
takovsky, 2009) and the references cited in those pa-
pers).
The aim of the present paper is the establishment
of a class of non Fickian models that take into ac-
count the viscoelastic behavior of the brain tissue.
The paper is organized as follows. Since the brain tis-
sue presents a viscoelastic behaviour that can be de-
scribed by the Voigt-Kelvin model (see for instance
(G.Franceschini, 2006), (Humphrey, 2003), (Mehra-
bian and Abousleiman, 2011)), we present in Sec-
tion 2 a class of non Fickian models to describe the
space and time evolution of glioma cancer cells con-
structed by combining the diffusion process with the
viscoelastic properties of the brain tissue. In Section
3 we study the behaviour of the glioma mass. In Sec-
tion 4 we introduce the numerical method that will be
used to obtain numerical approximations for the den-
sity of proliferation and migratory glioma cells. Plots
illustrating the evolution of gliomas are included in
Section 5. Finally, in Section 6 we present some con-
clusions.
2 A VISCOELASTIC MODEL
The class of non Fickian models that we present in
what follows is established taking into account the
viscoelastic nature of the brain tissue. Following (Ed-
wards and Cohen, 1995), (Edward and Cohen, 1995),
(Edwards, 1996), (Edwards, 2001) and (Shaw and
Whiteman, 1998), if a diffusion process occurs in a
medium that has a viscoelastic behaviour, then this
behaviour should be included in the diffusion equa-
tion which leads to a modified diffusion equation
∂c
∂t
= ∇.(D∇c) + ∇.(D
v
∇σ) + f(c) in Ω×(0,∞),
(4)
where σ represents the stress exerted by the brain tis-
sue on the tumor cells.
We assume that the viscoelastic behaviour of the
brain tissue is described by
∂σ
∂t
+ βσ = α
1
ε+ α
2
∂ε
∂t
, (5)
where ε stands for the strain. Equation (5) is based on
a mechanistic model which is represented by a spring
(restorativeforce component) and a dashpot (damping
component) in parallel connected with a free spring.
In (5) the viscoelastic characteristic time β is given
by β =
E
0
+E
1
µ
1
, and α
1
=
E
0
E
1
µ
1
, α
2
= E
0
where E
1
is
the Young modulus of the spring element, µ
1
repre-
sents the viscosity and E
0
stands for the Young mod-
ulus of the free spring (see (G.Franceschini, 2006),
(Humphrey, 2003), (Mehrabian and Abousleiman,
2011)).
Equation (5) leads to the following expression for
σ
σ(t) =
Z
t
0
e
−β(t−s)
α
1
ε(s) + α
2
∂ε
∂t
(s)
ds+ e
−βt
σ(0).
(6)
SIMULTECH2013-3rdInternationalConferenceonSimulationandModelingMethodologies,Technologiesand
Applications
690