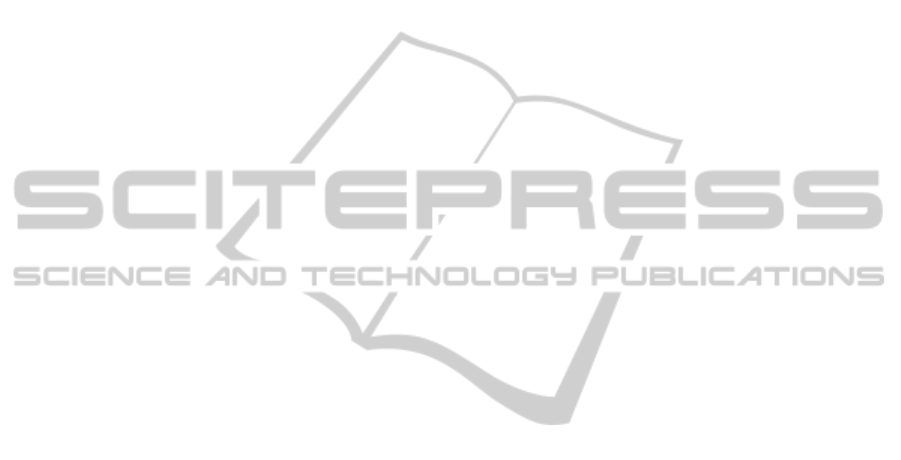
isterium fur Wirtschaft und Technologie (BMWi,
grant FKZ 50 RA 1012 and grant FKZ 50 RA 1011).
The authors like to thank Marc Tabie for providing us
with the evaluation data.
REFERENCES
Ahmadian, P., Cagnoni, S., and Ascari, L. (2013). How ca-
pable is non-invasive EEG data of predicting the next
movement? A mini review. Frontiers in Human Neu-
roscience, 7:124.
Bai, O., Rathi, V., Lin, P., Huang, D., Battapady, H., Fei,
D.-Y., Schneider, L., Houdayer, E., Chen, X., and
Hallett, M. (2011). Prediction of human voluntary
movement before it occurs. Clinical Neurophysiology,
122(2):364–372.
Blankertz, B., Dornhege, G., Lemm, S., Krauledat, M., Cu-
rio, G., and M¨uller, K. (2006). The Berlin Brain-
Computer Interface: machine learning based detec-
tion of user specific brain states. Journal of Universal
Computer Science, 12(6):581–607.
Chang, C.-C. and Lin, C.-J. (2011). LIBSVM:
A library for support vector machines. ACM
Transactions on Intelligent Systems and Tech-
nology, 2:27:1–27:27. Software available at
http://www.csie.ntu.edu.tw/ cjlin/libsvm.
Fabiani, M., Gratton, G., and Federmeier, K. D. (2007).
Event-related brain potentials: Methods, theory, and
applications. In Cacioppo, J., Tassinary, L. G., and
Berntson, G. G., editors, Handbook of Psychophys-
iology, pages 85–119. Cambridege University Press,
Cambridge [u.a], 3rd edition.
Folgheraiter, M., Jordan, M., Straube, S., Seeland, A., Kim,
S. K., and Kirchner, E. A. (2012). Measuring the im-
provement of the interaction comfort of a wearable ex-
oskeleton. International Journal of Social Robotics,
4(3):285–302.
Folgheraiter, M., Kirchner, E. A., Seeland, A., Kim, S. K.,
Jordan, M., W¨ohrle, H., Bongardt, B., Schmidt, S.,
Albiez, J., and Kirchner, F. (2011). A multimodal
brain-arm interface for operation of complex robotic
systems and upper limb motor recovery. In Vieira,
P., Fred, A., Filipe, J., and Gamboa, H., editors, In
Proceedings of the 4th International Conference on
Biomedical Electronics and Devices (BIODEVICES-
11), pages 150–162, Rome. SciTePress.
Kirchner, E. A., Albiez, J., Seeland, A., Jordan, M., and
Kirchner, F. (2013). Towards assistive robotics for
home rehabilitation. In Chimeno, M. F., Sol´e-Casals,
J., Fred, A., and Gamboa, H., editors, In Proceedings
of the 6th International Conference on Biomedical
Electronics and Devices (BIODEVICES-13), pages
168–177, Barcelona. SciTePress.
Kirchner, E. A. and Tabie, M. (2013). Closing the gap:
combined EEG and EMG analysis for early movement
prediction in exoskeleton based rehabilitation. In Pro-
ceedings of the 4th European Conference on Techni-
cally Assisted Rehabilitation - TAR 2013.
Kornhuber, H. H. and Deecke, L. (1965). Hirnpoten-
tial¨anderungen bei Willk¨urbewegungen und passiven
Bewegungen des Menschen: Bereitschaftspotential
und reafferente Potentiale. Pfl¨uger’s Archiv f¨ur die
gesamte Physiologie des Menschen und der Tiere,
284(1):1–17.
Kubat, M., Holte, R. C., and Matwin, S. (1998). Machine
learning for the detection of oil spills in satellite radar
images. Machine Learning, 30(2-3):195–215.
Lemm, S., Sch¨afer, C., and Curio, G. (2004). BCI competi-
tion 2003–data set III: probabilistic modeling of sen-
sorimotor mu rhythms for classification of imaginary
hand movements. IEEE Transactions on Biomedical
Engineering, 51(6):1077–80.
Libet, B., Gleason, C. A., Wright, E. W., and Pearl, D. K.
(1983). Time of conscious intention to act in relation
to onset of cerebral activity (readiness-potential) the
unconscious initiation of a freely voluntary act. Brain,
106(3):623–642.
Mohammadi, R., Mahloojifar, A., and Coyle, D. (2012).
A combination of pre- and postprocessing techniques
to enhance self-paced BCIs. Advances in Human-
Computer Interaction, 2012:3:1–3:10.
Rivet, B., Souloumiac, A., Attina, V., and Gibert, G. (2009).
xDAWN algorithm to enhance evoked potentials: ap-
plication to brain-computer interface. IEEE Transac-
tions on Biomedical Engineering, 56(8):2035–2043.
Solis-Escalante, T., M¨uller-Putz, G., and Pfurtscheller, G.
(2008). Overt foot movement detection in one single
laplacian EEG derivation. Journal of Neuroscience
Methods, 175(1):148–153.
Tabie, M. and Kirchner, E. A. (2013). EMG onset detec-
tion – comparison of different methods for a move-
ment prediction task based on EMG. In Alvarez,
S., Sol´e-Casals, J., Fred, A., and Gamboa, H., ed-
itors, In Proceedings of the 6th International Con-
ference on Bio-inspired Systems and Signal Process-
ing (BIOSIGNALS-13), pages 242–247, Barcelona.
SciTePress.
Vapnik, V. N. (1995). The nature of statistical learning the-
ory. Springer New York, Inc., New York, NY, USA.
Zhu, X., Wu, J., Cheng, Y., and Wang, Y. (2006). GMM-
based classification method for continuous prediction
in brain-computer interface. In Proceedings of the
18th International Conference on Pattern Recognition
- Volume 01, ICPR ’06, pages 1171–1174, Washing-
ton, DC, USA. IEEE Computer Society.
NEUROTECHNIX2013-InternationalCongressonNeurotechnology,ElectronicsandInformatics
20