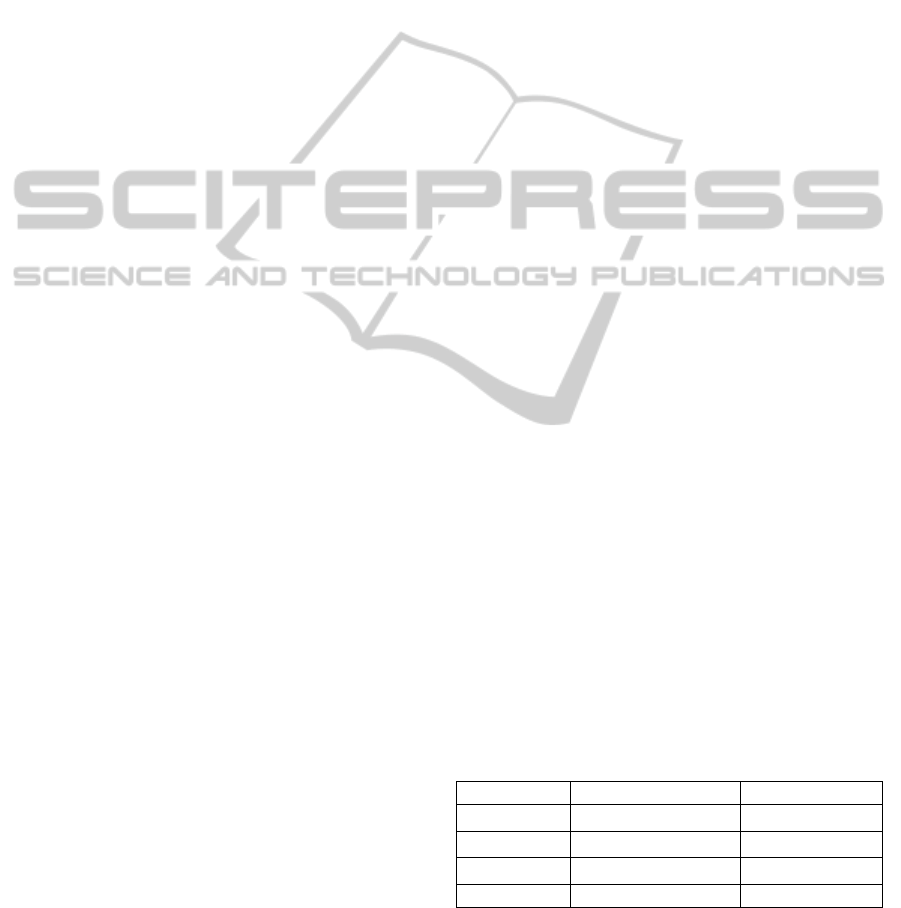
bpm/s, the algorithm detects a type III artefact
(Figure 2, fourth plot).
The thresholds that are used for the detection of
type II and III artefacts are selected by trial and
error.
2.2.3 Performance Measures
The percentage of time that one training session
contains artefacts is computed for all trainings. This
is done for each type of artefact separately, as well
as for all types combined. These measures are
weighted with the duration of the training: the
largest weight is 1 and corresponds with a training
duration of 2 h.
2.3 Pre-processing Algorithm
In the project, for which this data is acquired, a high
quality and reliable heart rate signal of 1 Hz is
required. The first step in the pre-processing
algorithm therefore would be to low-pass filter the 5
Hz heart rate signal to remove high-frequent
information. However, this filtering introduces large
errors in periods where artefacts are present.
Therefore, artefacts are first removed from the heart
rate by linear interpolation (Figure 3, second plot).
During our measurements the median duration of
type I, II and III artefacts were 4.1, 1.7 and 14.8
seconds respectively. Therefore linear interpolation
produced adequate results.
Next a low-pass Butterworth filter with cut-off
frequency of 1 Hz is applied on this signal (Figure 3,
third plot).
Finally heart rate during artefacts is replaced
with cubic interpolation, to take into account the
non-linear characteristics of heart rate (
Figure 3,
fourth plot).
2.4 Statistical Analysis
The performance measures are calculated before and
after pre-processing. The effect of pre-processing on
the different types of artefacts can thus be
investigated.
Normality of each group is tested with a
Lilliefors test. The null-hypothesis (data is
distributed normally) was rejected for each group
(p<0.001). Since comparisons are made between two
paired, non-parametric groups, the Wilcoxon test is
used.
3 RESULTS AND DISCUSSION
Table 1 reveals that most of the artefacts in this
dataset are of type III; the median of type I and II
artefacts are only 0.1 % and 0.9 % respectively,
while this is 6.7 % for type III. The median of the
total percentage of artefacts is 9.0 %, which
corresponds with 11 minutes of unreliable data per
training. In total 37 hours of artefacts are present in
the data.
Another interesting result is that the total
percentage of artefacts is reduced significantly
(p<0.01) after pre-processing. The total period of
data containing artefacts is reduced with more than
10 hours. Even more, type I and II artefacts are
almost completely removed from the raw data. The
pre-processing algorithm presented in this paper is
thus able to reduce type I and II artefacts
significantly (p<0.01).
Type III artefacts on the other hand are still
present after pre-processing, although they are
decreased from 6.7 to 4.2 %. In this case more
advanced methods are required.
4 CONCLUSIONS
In this paper an artefact detection algorithm for heart
rate is presented, which is able to automatically
detect three types of artefacts; physiologically not
meaningful heart rates (type I), heart rate changes
that are too fast (type II) and changes that show too
little variation (type III).
The pre-processing algorithm could not reduce
type III artefacts. More advanced techniques are
needed here to recover this data. Nevertheless
periods in heart rate still containing errors can be
detected automatically. In further analysis this
information can be taken into account.
Table 1: Median and interquartile range,
a
shows statistical
significance (p < 0.01) between group before and group
after pre-processing.
Artefacts Before Pre-processing After Pre-processing
Total (%)
9.0
+9.8
-6.0
a
4.4
+8.1
-4.1
Type I (%)
0.1
+1.4
-0.1
a
0
+0.02
-0
Type II (%)
0.9
+1.0
-0.5
a
0.04
+0.13
-0.03
Type III (%)
6.7
+10.4
-6.4
4.2
+8.2
-4.0
It is also shown that with pre-processing the total
percentage of artefacts can be significantly reduced.
Typical artefacts (type I and II) in heart rate can
even be almost completely removed from raw data.
CARDIOTECHNIX2013-InternationalCongressonCardiovascularTechnologies
60