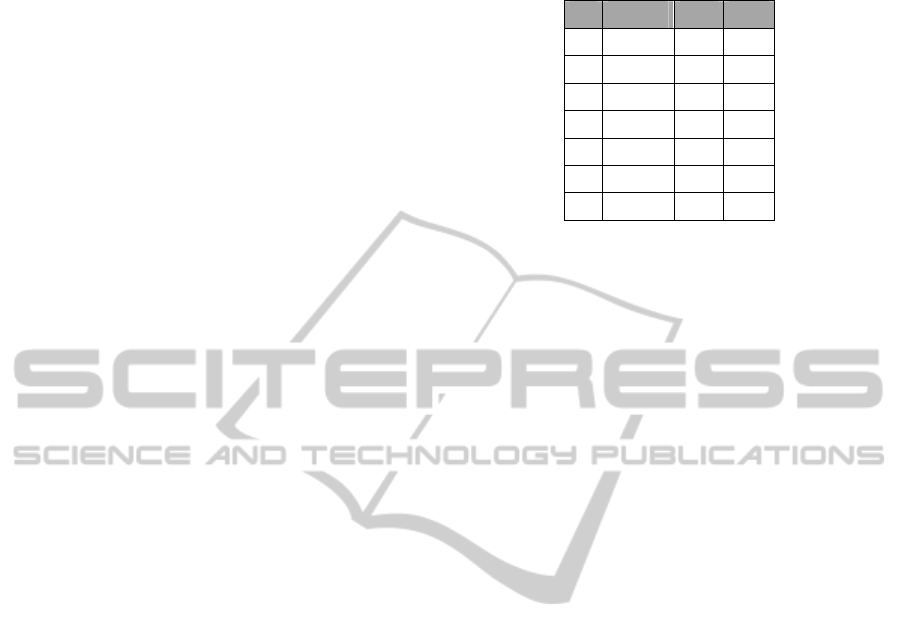
very precisely. Extensions of PerPot are now able to
determine the individual anaerobe threshold (IAT)
by simulation, enabling optimization of speed and
heart rate profiles in endurance sports (Perl and
Endler, 2006).
The meta-model PerPot describes physiological
adaptation on an abstract level as an antagonistic
process: A load input flow is identically feeding a
strain potential and a response potential. The
response potential increases the performance
potential by a positive flow, while the strain
potential reduces it by a negative flow. All flows
show specific delays, thus modeling the time the
components of the modeled system need to react. In
particular in endurance sports delays play an
important role for the process of fatigue and
recovering (Perl, 2005).
3.2 Experiences
In order to examine the system regarding the
optimization of the target time in running, an
experimental pre-test has been conducted in a first
step. For this purpose, a number of (hobby-) athletes
were asked to perform the following sequence of
runs:
1. Calibration run: In order to determine the
individual performance parameters (strain
potential, response potential) of an athlete
required for the PerPot module, a run similar to a
step-test had to be performed. Based on these
parameters, the system is able to calculate the
optimal goal time and speed for a given distance
thereby forming the basis for the generation of
feedback.
2. Free run of about 10 kilometres or at least 40
minutes (without feedback messages).
The athletes were instructed to perform this run
to the best of their ability.
3. Run over the same distance as the second run,
but this time with consideration of the automatic
feedback messages of the PerPot module.
In order to limit side effects, all runs were carried
out on a flat track. Moreover, the athletes had to
complete the three runs within 2 weeks and without
doing any other physical sport activities in between
in order to avoid training effects.
As shown in Table 1, the results between the
three runs and athletes are quite different and do not
always follow a clear trend. In consideration to
several factors like actual weather conditions, the
athletes’ condition and motivation – which all have
influence on the results – this may not be
unsuspected.
Table 1: Pre-test results.T1: Time of free run. T2: Time of
run with feedback messages.
Nr. Distance T1 T2
1 10.000m 47:46 50:36
2 8.000m 46:46 45:08
3 9.250m 44:40 43:35
4 7.950m 45:55 44:31
5 9.600m 54:45 54:11
6 9.400m 49:26 41:36
7 9.200m 50:19 47:02
However, six out of seven athletes reached a
better target time when using the MMA – with
improvements from one up to eight minutes.
Consequently, the MMA shows promising
tendencies for improving performance data of
runners.
Another observation is that some of the athletes,
who did not reach the predicted target time, did not
give the responsibility to the system, but rather to
other factors such as their actual condition on the
day. Overall, however, athletes seem to trust in the
developed system – even in the case when they did
not reach the predicted target time.
It appears that the athletes try to follow the
feedback messages as good as possible – although
the frequency of feedback messages can be quite
high (up to 15 seconds interval). It usually takes
about 15 seconds for the athlete to react to the
feedback. This may be an indication for the
acceptance of the MMA, as athletes who do not trust
in the system would not have enough motivation for
following the feedback messages.
REFERENCES
Baca, A., Kornfeind, P., Preuschl, E., Bichler, S., Tampier,
M. and Novatchkov, H., 2010, A Server-Based Mobile
Coaching System, Sensors, 10, 10640-10662.
Perl, J., 2005, Dynamic simulation of performance
development: Prediction and optimal scheduling. Int.
Journal of Computer Science in Sport, 4, 28-37.
Perl, J. and Endler, S., 2006, Training- and Contest-
scheduling in Endurance Sports by Means of Course
Profiles and PerPot-based Analysis. International
Journal of Computer Science in Sport, 5 (2), 42-46.
Tampier, M., Baca, A., and Novatchkov, H., 2012, E-
Coaching in Sports. In Proc. 2012 Pre-Olympic
Congress, Liverpool, England, UK.