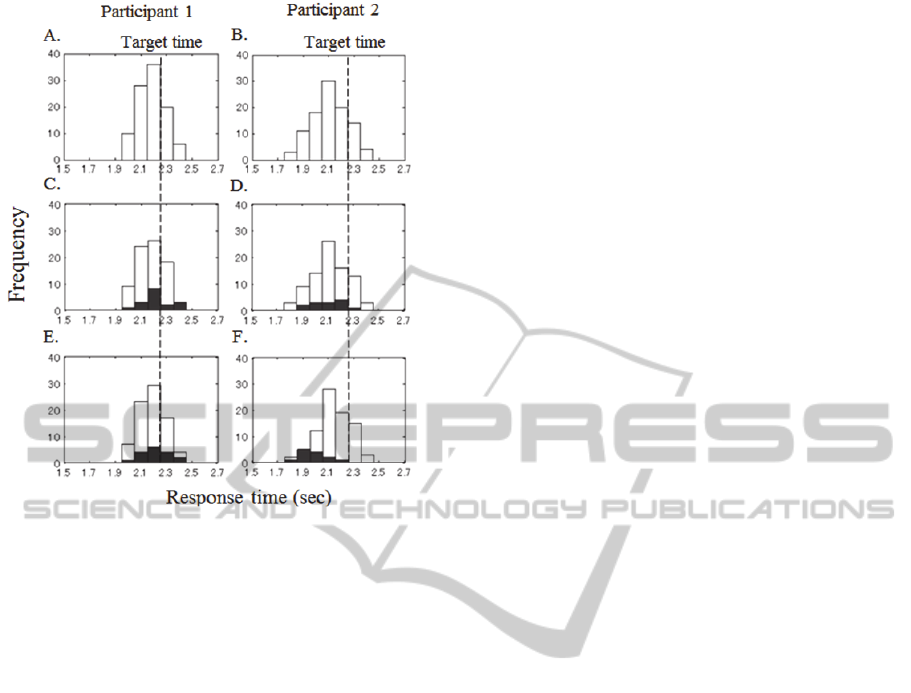
Figure 5: Inter-individual differences in trial by trial
compensation. Examples of two participants are shown.
(A, B) Histograms of response time on the whole trials in
Risk condition. Both histograms are normally distributed.
(C, D) Histograms of response time from the trials before
miss (filled bars) and success (open bars). Histograms
have a similar shape between them and between the
participants. (E, F) Histogram of response time from the
trials following to miss (filled bars) and success (open
bars). In participants 2, earlier responses to the target time
are shown after miss trials more than after success trials.
In participants 1, consecutive misses were shown.
4 DISCUSSION
This is the first study to assess the relationship
between risk-sensitivity and task performance. We
showed that the participants tended to be risk-
seeking under a risk situation where high gain and
zero gain are joining to each other. This risk-seeking
strategy, as well as response variability, had a
significant effect on degrading the task performance.
In Risk condition, the observed response time
was closer to the target time than the theoretically
calculated optimal response time, that is, all the
participants tended to be risk-seeking. Cumulative
prospect theory (Tversky and Kahneman, 1992)
would explain that this tendency might be due to
underestimation of their own response variance (Wu
et al., 2009); (O’Brien and Ahmed, 2013);
(Nagengast, et al 2011). The participants might
believe themselves to have smaller response
variability than they actually have, which would
likely influence them to respond closer to the target
time.
The asymmetric score function was applied in
Risk condition. Wu et al., (2006) found that
participants performed suboptimally when pointing
in the asymmetric expected gain landscape. On the
other hand, Trommershäuser et al., (2003a; 2003b)
showed risk-neutral and optimal movement planning
in the symmetric expected gain landscape. Our
participants might not be able to perform a
theoretically optimal strategy under the asymmetric
score function. This was confirmed by calculating
the difference between the observed total score and
the theoretically calculated total score. From the
typical example in Figure 2, this participant could
improve 924 points that was calculated by
subtracting the observed total score (i.e., 7582
points) from the optimal total score (i.e., 8596
points).
Risk-seeking behaviours are sometimes observed
in real sports fields. For example, professional NBA
basketball players attempt consecutive three point
shots after they successfully scored three points even
though the probability of taking points is decreased
(Neiman and Lowenstein, 2011). They may believe
they will succeed again. Therefore, suboptimal
decision-making would have the effect on degrading
a performance of beginners as well as experts in a
variety of sports.
We also investigated inter-individual differences
in responses following miss trials. In participants 2
in Figure 5F, earlier responses to the target time
were shown after miss trials more often than after
success trials. This indicated that he made a large
compensation with a different strategy. This
tendency was not shown in participants 1 (Figure
5E). The histogram of the response time from the
trials following to miss had similar shape to that
from the trials following to success. In contrast to
Figure 5F, some responses were plotted over the
target time, which indicated that consecutive misses
were shown. For this participant, the total score will
surely increase by responding within the target time
with surely strategy to avoid consecutive miss. The
behaviour following to miss would be an important
factor to explain the individual response pattern.
Thus, we need to investigate how these differences
are produced in future studies.
As implication for real sports field, our results
suggest that it is important to evaluate and improve
optimal strategy depending on each player’s skill
level. Coaches and trainers often instruct how to
move a body focusing on a form itself. In addition to
SuboptimalStrategyinPerformingCoincidentTimingTaskunderRisk
17