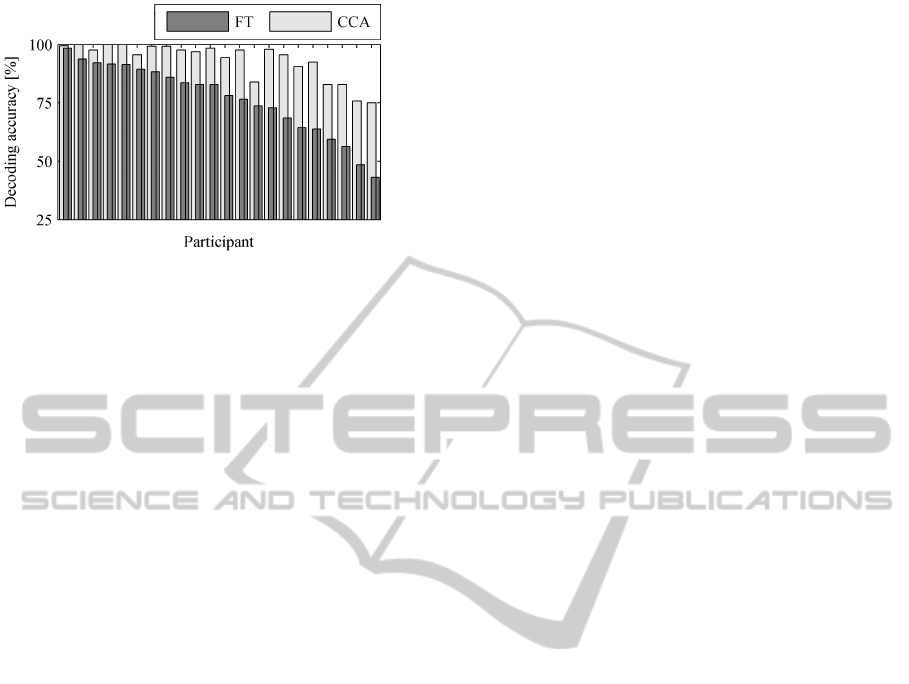
Figure 2: Single subject performance obtained in an SOV.
Each of the 22 subjects shows improvement of decoding
accuracy with the CCA method (light gray bars) compared
to classification of FT features (dark gray bars, sorted in
descending order).
extraction method combined with multivariate classi-
fication to CCA/MC regarding their performance in
MEG based BCIs. With the CCA approach, decoding
accuracy was considerably improved compared to FT.
This held already for analysis windows as short as one
second. The higher accuracy of CCA even with short
data windows considerably increased the information
transfer rates as compared to FT. This result suggests
that CCA/MC is an efficient method for high through-
put SSVEF-BCIs. A further advantage of CCA/MC
over FT/rLR in the context of BCI is that CCA/MC, as
opposed to FT/rLR does not require training blocks.
Thus, lengthy initial phases for acquisition of train-
ing data can be avoided. In BCI-practice, the model
estimation for each single trial in CCA can lead to im-
proved robustness against sensor replacement, sensor
malfunction and non-static brain patterns. This ren-
ders the CCA method as a flexible and reliable feature
extraction method for multi-channel BCIs controlled
by shifting attention to oscillating visual stimuli.
We confirmed in our study the finding that in-
clusion of harmonics significantly increases classifi-
cation accuracy in SSVEP BCIs (M¨uller-Putz et al.,
2005). The performance increase was small for CCA
but statistically significant. However, other authors
did not find such a benefit (Bin et al., 2009). Im-
portantly, the better performance of CCA/MC than
FT/rLR was independent of whether harmonics were
included or not.
In this study we demonstrate for the first time that
magnetic SSVEFs are suitable to control a BCI. In
particular, SSVEFs were decoded for selection of ob-
jects, presented in a VR scenario. Furthermore, we
showed that the CCA is a powerful method to rapidly
detect target frequencies in the MEG. The method in-
troduced in this work is capable of decomposing fre-
quency components of sources that are spatially dis-
tributed over dense sensor arrays. Importantly, the
proposed algorithm can be executed in several mil-
liseconds and, consequently, it is suited for BCI im-
plementation and can be applied online.
Even though MEG is not suited for home use,
this modality is suited for BCI development. Despite
reports of higher ITRs in some EEG based SSVEP
studies (Bin et al., 2009) we believe that the BCI
accuracy strongly depends on the visual stimulation.
Therefore, comparison of ITRs should be treated with
caution. Furthermore, an MEG system could serve
as a training device to familiarize patients with BCI
paradigms.
ACKNOWLEDGEMENTS
This work was supported by the EU project ECHORD
number 231143 from the 7th Framework Programme,
by Land-Sachsen-Anhalt Grant MK48-2009/003 and
by a Lower Saxony grant for the Center of Safety Crit-
ical Systems Engineering.
REFERENCES
Bin, G., Gao, X., Yan, Z., Hong, B., and Gao, S.
(2009). An online multi-channel SSVEP-based brain-
computer interface using a canonical correlation anal-
ysis method. J Neural Eng, 6(4):046002.
Friman, O., Volosyak, I., and Gr¨aser, A. (2007). Mul-
tiple channel detection of steady-state visual evoked
potentials for brain-computer interfaces. IEEE Trans
Biomed Eng, 54(4):742–750.
Horki, P., Solis-Escalante, T., Neuper, C., and M¨uller-Putz,
G. (2011). Combined motor imagery and SSVEP
based BCI control of a 2 DoF artificial upper limb.
Med Biol Eng Comput, 49(5):567–577.
Lin, Z., Zhang, C., Wu, W., and Gao, X. (2007). Frequency
recognition based on canonical correlation analysis
for SSVEP-based BCIs. IEEE Trans Biomed Eng,
54(6 Pt 2):1172–1176.
M¨uller, M. M., Teder, W., and Hillyard, S. A. (1997). Mag-
netoencephalographic recording of steady-state visual
evoked cortical activity. Brain Topogr, 9(3):163–168.
M¨uller-Putz, G. R., Scherer, R., Brauneis, C., and
Pfurtscheller, G. (2005). Steady-state visual evoked
potential (SSVEP)-based communication: impact of
harmonic frequency components. J Neural Eng,
2(4):123–130.
Quandt, F., Reichert, C., Hinrichs, H., Heinze, H. J., Knight,
R. T., and Rieger, J. W. (2012). Single trial dis-
crimination of individual finger movements on one
hand: A combined MEG and EEG study. Neuroim-
age, 59(4):3316–3324.
Reichert, C., Kennel, M., Kruse, R., Heinze, H. J.,
Schmucker, U., Hinrichs, H., and Rieger, J. W. (2013).
NEUROTECHNIX2013-InternationalCongressonNeurotechnology,ElectronicsandInformatics
236