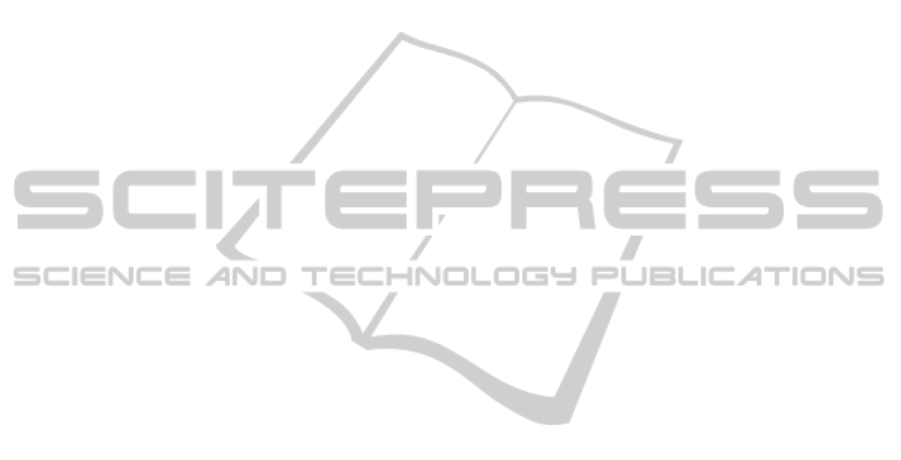
man National Research Center for Information Tech-
nology.
Jaeger, H., Luko
ˇ
sevi
ˇ
cius, M., Popovici, D., and Siewert, U.
(2007). Optimization and applications of echo state
networks with leaky-integrator neurons. Neural Net-
works, 20(3):335–352.
Kayser, K. (2011). Quantification of virtual slides: Ap-
proaches to analysis of content-based image informa-
tion. Journal of Pathology Informatics, 2:2.
Kayser, K., Borkenfeld, S., and Kayser, G. (2012). How
to introduce virtual microscopy (VM) in routine di-
agnostic pathology: constraints, ideas, and solutions.
Analytical Cellular Patholology, 35(1):3–10.
Kayser, K., G
¨
ortler, J., Bogovac, M., Bogovac, A., Gold-
mann, T., Vollmer, E., and Kayser, G. (2009). AI
(artificial intelligence) in histopathology - from image
analysis to automated diagnosis. Folia Histochemica
et Cytobiologica, 47(3):355–361.
Kayser, K., Gortler, J., Goldmann, T., Vollmer, E., Hufnagl,
P., and Kayser, G. (2008a). Image standards in tissue-
based diagnosis (diagnostic surgical pathology). Di-
agnostic Pathology, 3:17.
Kayser, K., Hoshang, S. A., Metze, K., Goldmann,
T., Vollmer, E., Radziszowski, D., Kosjerina, Z.,
Mireskandari, M., and Kayser, G. (2008b). Texture-
and object-related automated information analysis in
histological still images of various organs. Analytical
and Quantitative Cytology and Histology, 30(6):323–
335.
Kuntimad, G. and Ranganath, H. (1999). Perfect image seg-
mentation using pulse coupled neural networks. IEEE
Transactions on Neural Networks, 10(3):591–598.
Lin, W., Xiao, J., and Micheli-Tzanakou, E. (1998). A
computational intelligence system for cell classifica-
tion. In Proceedings of the 1998 IEEE international
conference on information technology applications in
biomedicine, pages 105–109.
Lu, K.-C. and Yang, D.-L. (2009). Image processing and
image mining using decision trees. Journal of Infor-
mation Science and Engineering, 25:989–1003.
Luko
ˇ
sevi
ˇ
cius, M. and Jaeger, H. (2009). Reservoir comput-
ing approaches to recurrent neural network training.
Computer Science Review, 3(3):127 – 149.
Luko
ˇ
sevi
ˇ
cius, M., Jaeger, H., and Schrauwen, B. (2012).
Reservoir computing trends. KI - K
¨
unstliche Intelli-
genz, pages 1–7.
Maass, W. (2010). Motivation, theory, and applications of
liquid state machines. In Cooper, B. and Sorbi, A.,
editors, Computability in Context: Computation and
Logic in the Real World, pages 275–296. Imperial Col-
lege Press, London.
Maass, W., Legenstein, R., and Markram, H. (2002a).
A new approach towards vision suggested by bio-
logically realistic neural microcircuit models. In
Buelthoff, H. H., Lee, S. W., Poggio, T. A., and Wall-
raven, C., editors, Biologically Motivated Computer
Vision. Proc. of the Second International Workshop,
BMCV 2002, Tuebingen, Germany, November 22–24,
2002, volume 2525 of Lecture Notes in Computer Sci-
ence, pages 282–293. Springer (Berlin).
Maass, W. and Markram, H. (2004). On the computational
power of circuits of spiking neurons. Journal of Com-
puter and System Sciences, 69:593–616.
Maass, W., Natschl
¨
ager, T., and Markram, H. (2002b).
Real-time computing without stable states: A new
framework for neural computation based on perturba-
tions. Neural Computation, 14(11):2531–2560.
Mitra, S. and Pal, S. K. (2005). Fuzzy sets in pattern recog-
nition and machine intelligence. Fuzzy Sets and Sys-
tems, 156(3):381–386.
Molnar, B., Berczi, L., Diczhazy, C., Tagscherer, A., Varga,
S. V., Szende, B., and Tulassay, Z. (2003). Dig-
ital slide and virtual microscopy based routine and
telepathology evaluation of routine gastrointestinal
biopsy specimens. Journal of Clinical Pathology,
56(6):433–438.
Mori, I., Nunobiki, O., Ozaki, T., Taniguchi, E., and
Kakudo, K. (2008). Issues for application of virtual
microscopy to cytoscreening, perspectives based on
questionnaire to japanese cytotechnologists. Diagnos-
tic Pathology, 3(Suppl 1):S15.
Norton, D. and Ventura, D. (2006). Preparing more effective
liquid state machines using Hebbian learning. In Pro-
ceedings of the IEEE International Joint Conference
on Neural Networks IJCNN’06, pages 8359–8364.
Norton, D. and Ventura, D. (2010). Improving liquid state
machines through iterative refinement of the reservoir.
Neurocomputing, 73(16-18):2893–2904.
Ranganath, H. and Kuntimad, G. (1999). Object detection
using pulse coupled neural networks. IEEE Transac-
tions on Neural Networks, 10(3):615 –620.
Revell, P. A. (1983). Histomorphometry of bone. Journal
of Clinical Pathology, 36:1323–1331.
Riber-Hansen, R., Vainer, B., and Steiniche, T. (2012). Dig-
ital image analysis: a review of reproducibility, stabil-
ity and basic requirements for optimal results. APMIS,
120(4):276–289.
Schaberg, E. S., Jordan, W. H., and Kuyatt, B. L. (1992).
Artificial intelligence in automated classification of rat
vaginal smear cells. Analytical and Quantitative Cy-
tology and Histology, 14(6):446–450.
Schrauwen, B. and Van Campenhout, J. (2003). BSA, a fast
and accurate spike train encoding scheme. In IEEE
International Joint Conference on Neural Networks
(IJCNN), pages 2825–2830. IEEE.
Schrauwen, B., Verstraeten, D., and Van Campenhout, J.
(2007). An overview of reservoir computing: theory,
applications and implementations. In Proceedings of
the 15th European Symposium on Artificial Neural
Networks, pages 471–482.
Shivhare, S. and Shrivastava, R. (2012). Automatic Bone
Marrow White Blood Cell Classfication using Mor-
phological Granulometric Feature of Nucleus. Inter-
national Journal of Scientific & Technology Research,
1(4):125–131.
Sj
¨
ostr
¨
om, P. J., Frydel, B. R., and Wahlberg, L. U. (1999).
Artificial neural network-aided image analysis system
for cell counting. Cytometry, 36(1):18–26.
Specht, D. F. (1990). Probabilistic neural networks. Neural
Networks, 3:109–118.
AutomatedClassificationofHaematopoieticCompartmentsintheHumanBoneMarrowusingReservoirComputing
17