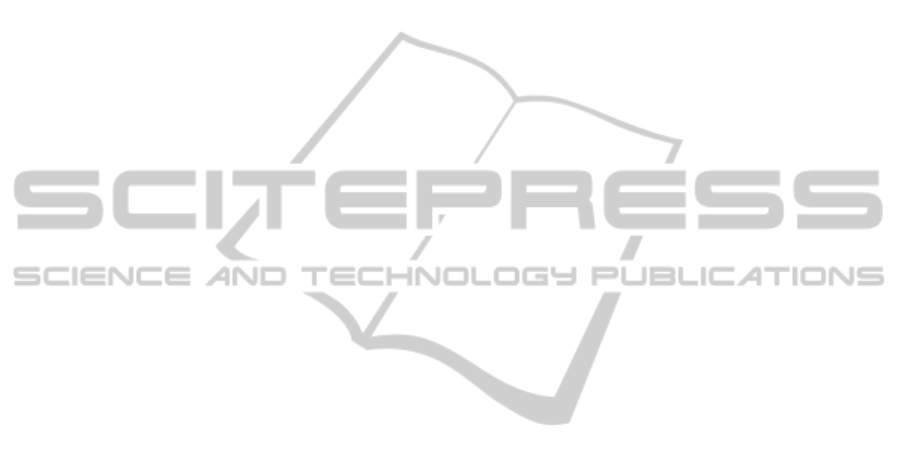
quire some adjustment before optical flow and in-
ternal self-motion signals produce a coherent sig-
nal again.
• Grid cells which are already present in a coarse
and rudimentary form in young rats even before
they start exploring their environment can be ex-
plained by random position inputs generated dur-
ing development.
Irrespective of these specific aspects regarding grid
cells the idea of a dendritic self-organizing map has
itself some intriguing consequences. Commonly, the
units of a self-organizing map (SOM) represent sin-
gle classes. In order to increase the “resolution” of a
SOM more units must be added. But the more units
are present in a SOM, the less inputs will be directed
towards each individual unit. As fewer and fewer in-
puts reach individual units, learning of new inputs be-
comes increasingly difficult. Additionally, if the in-
put is high-dimensional, the “curse of dimensional-
ity” will lead to a weak discrimination between the
individual units of the SOM. This contrasts the way
how grid cells encode information. Instead of repre-
senting a single region of the input space they sample
the whole input space at regular intervals, thus repre-
senting many regions, i.e., many classes, at once. In
order to identify a specific region of the input space,
grid cells of different groups with different orienta-
tion and spacing form a population code that uniquely
identifies the particular region. As each cell has only
a few units, i.e., dendritic subsections, in their local
SOM they suffer less from the curse of dimensional-
ity. Furthermore, a grid cell encoding of information
may be metabolically more efficient than the encod-
ing represented by a common SOM. Grid cells cover
the whole input space and are to that effect more fre-
quently active than cells of a SOM which may be only
rarely used but have to be maintained anyway.
8 CONCLUSIONS AND
OUTLOOK
We have presented a plausible computational model
of grid cells using a dendritic self-organizing map as
one of its core components. The model is not only
able to generate the peculiar, triangular firing pattern
of grid cells, but is also able to provide plausible ar-
guments that can account for several other phenomena
observed in the context of grid cells.
We argue that the way how grid cells encode spa-
tial information may represent a more general con-
cept of how high-level information is processed in the
brain. It appears promising to investigate how other
input modalities can be represented by a population
code of dendritic SOMs. These modalities include
high level feature descriptions of images, sound or
more abstract signals like the posture of the body.
REFERENCES
Barry, C., Ginzberg, L. L., OKeefe, J., and Burgess, N.
(2012). Grid cell firing patterns signal environmen-
tal novelty by expansion. Proceedings of the National
Academy of Sciences, 109(43):17687–17692.
Burgess, N., Barry, C., and O’Keefe, J. (2007). An oscilla-
tory interference model of grid cell firing. Hippocam-
pus, 17(9):801–812.
Derdikman, D., Whitlock, J. R., Tsao, A., Fyhn, M., Haft-
ing, T., Moser, M.-B., and Moser, E. I. (2009). Frag-
mentation of grid cell maps in a multicompartment en-
vironment. Nat Neurosci, 12(10):1325–1332.
Fiete, I. R., Burak, Y., and Brookings, T. (2008). What
grid cells convey about rat location. The Journal of
Neuroscience, 28(27):6858–6871.
Fritzke, B. (1995). A growing neural gas network learns
topologies. In Advances in Neural Information Pro-
cessing Systems 7, pages 625–632. MIT Press.
Fuhs, M. C. and Touretzky, D. S. (2006). A spin glass model
of path integration in rat medial entorhinal cortex. The
Journal of Neuroscience, 26(16):4266–4276.
Fyhn, M., Hafting, T., Treves, A., Moser, M.-B., and
Moser, E. I. (2007). Hippocampal remapping and
grid realignment in entorhinal cortex. Nature,
446(7132):190–194.
Fyhn, M., Molden, S., Witter, M. P., Moser, E. I., and
Moser, M.-B. (2004). Spatial representation in the en-
torhinal cortex. Science, 305(5688):1258–1264.
Giocomo, L., Moser, M.-B., and Moser, E. (2011). Com-
putational models of grid cells. Neuron, 71(4):589 –
603.
Hafting, T., Fyhn, M., Molden, S., Moser, M.-B., and
Moser, E. I. (2005). Microstructure of a spatial map
in the entorhinal cortex. Nature, 436(7052):801–806.
Kropff, E. and Treves, A. (2008). The emergence of grid
cells: Intelligent design or just adaptation? Hip-
pocampus, 18(12):1256–1269.
Langston, R. F., Ainge, J. A., Couey, J. J., Canto, C. B.,
Bjerknes, T. L., Witter, M. P., Moser, E. I., and Moser,
M.-B. (2010). Development of the spatial representa-
tion system in the rat. Science, 328(5985):1576–1580.
McNaughton, B. L., Battaglia, F. P., Jensen, O., Moser, E. I.,
and Moser, M.-B. (2006). Path integration and the
neural basis of the ’cognitive map’. Nat Rev Neurosci,
7(8):663–678.
Mhatre, H., Gorchetchnikov, A., and Grossberg, S. (2012).
Grid cell hexagonal patterns formed by fast self-
organized learning within entorhinal cortex. Hip-
pocampus, 22(2):320–334.
Monaco, J. D. and Abbott, L. F. (2011). Modular realign-
ment of entorhinal grid cell activity as a basis for hip-
pocampal remapping. The Journal of Neuroscience,
31(25):9414–9425.
IJCCI2013-InternationalJointConferenceonComputationalIntelligence
428