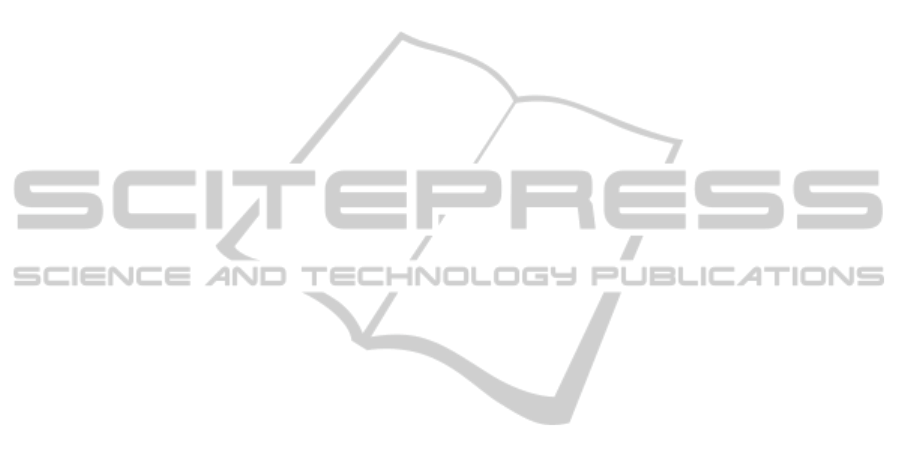
activity and thus increase of DCN activity). On the
other hand, the need of decreasing VOR and the
presence of overcompensation enable
simultaneously the reduction of agonist DCN
activity (PF-PC LTP) and the increase of antagonist
DCN activity (PF-PC LTD). Thus, during the VOR
gain-down, the stiffness of the muscle is temporally
increased and, after some time, it is reduced to the
minimal level.
In summary, a simple model with parallel,
sparsely coded channels, and with a single plasticity
mechanism that alters a subset of these channels, can
go a long way in explaining the general capacity of
motor learning in the VOR to exhibit specificity for
the particular stimuli present during training.
Learning, modulation and extinction proprieties
emerge.
According to the plasticity distribution at
multiple synaptic sites (Gao et al., 2012), the next
steps will be focused on the activation of the other
plasticity rules of the cerebellar model, expecting a
more stable and more accurate learning. The
modulation of these connectivities (MF→DCN and
PC→DCN) should lead to a learning generalization,
which will be tested through multiple tasks, such as
force-field paradigm in multi-joint reaching and
associative protocols such as eye blinking classical
conditioning task (Hwang and Shadmehr, 2005;
Yamamoto et al., 2007; Hoffland et al., 2012).
Moreover, for a more realistic computational
scenario, the sensorimotor platform will embed the
spiking version of this developed multiple-plasticity
cerebellar model (Luque et al., 2011).
5 CONCLUSIONS
The developed platform, a real neuro-robot able to
interact with the environment in multiple forms, is a
flexible and versatile test bed to concretely interpret
specific features of functional biological models, in
terms of neural connectivity, plasticity mechanisms
and functional roles into different closed-loop
sensorimotor tasks. In particular, the focus is on the
most CNS plastic structure, the cerebellum.
ACKNOWLEDGEMENTS
This work has been supported by the EU grant
REALNET (FP7-ICT-270434).
REFERENCES
Albus, J.S. (1971). A Theory of Cerebellar Function. Math
Biosci., 10, 25–61.
Boyden, E.S., Katoh, A., Raymond, J.L. (2004).
Cerebellum-dependant Learning: The Role of Multiple
Plasticity Mechanisms. Annu Rev Neurosci., 27, 581–
609.
Burdess, C. (1996). The Vestibulo-Ocular Reflex:
Computation in The Cerebellar Flocculus. Retrieved
(n.d.) from hxxp://bluezoo.org/vor/vor.pdf.
Casellato, C., Pedrocchi, A., Garrido, J.A., Luque, N.R.,
Ferrigno, G., D’Angelo, E., Ros, A. (2012). An
Integrated Motor Control Loop of a Human-like
Robotic Arm: Feedforward, Feedback and
Cerebellum-based Learning. Biomedical Robotics and
Biomechatronics (BioRob), 2012 June 24-27 4th IEEE
RAS & EMBS International Conference, no., 562-567.
doi: 10.1109/BioRob.2012.6290791.
Donchin, O., Rabe, K., Diedrichsen, J., Schoch, B.,
Gizewski, E.R., Timmann, D. (2012). Cerebellar
Regions Involved in Adaptation to Force Field and
Visuomotor Perturbation. J Neurophysiol., 107, 134–
147.
Gao, Z., van Beugen, B.J., De Zeeuw, C.I. (2012).
Distributed Synergistic Plasticity and Cerebellar
Learning. Nat Rev Neurosci., 13, 619-35.
Garrido, J.A., Luque, N.R., D’Angelo, E., Ros, E.
Distributed cerebellar plasticity implements adaptable
gain control in a manipulation task: a closed-loop
robotic simulation. Submitted to Front. Neural
Circuits.
Hwang, E.J., Shadmehr, R.J. (2005). Internal Models of
Limb Dynamics and The Encoding of Limb State.
Neural Eng., 2(3), 266-78.
Hoffland, B.S., Bologna, M., Kassavetis, P., Teo, J.T.H.,
Rothwell, J.C., Yeo, C.H., van de Warrenburg, B.P.,
Edwards, M.J. (2012). Cerebellar Theta Burst
Stimulation Impairs Eyeblink Classical Conditioning.
J Physiol., 590(4), 887-897.
Ito M. (2006). Cerebellar Circuitry as a Neuronal
Machine. Prog Neurobiol., 78, 272–303.
Luque, N.R., Garrido, J.A., Carrillo, R.R., Coenen, O.J.,
Ros, E. (2011). Cerebellar Input Configuration
Toward Object Model Abstraction in Manipulation
Tasks. Neural Networks, IEEE Transactions on, 22(8),
1321-1328.
Marr, D. (1969). A Theory of Cerebellar Cortex. J
Physiol., 202(2), 437–470.
van der Smagt, P. (2000). Benchmarking Cerebellar
Control. Robotics and Autonomous Systems, 32, 237-
251.
Yamamoto, K., Kawato, M., Kotosaka, S., Kitazawa, S.
(2007). Encoding of Movement Dynamics by Purkinje
Cell Simple Spike Activity during Fast Arm
Movements under Resistive and Assistive Force Field.
J Neurophysiol., 97(2), 1588-99.
Yamazaki, T., Tanaka, S. (2007). The cerebellum as a
liquid state machine. Neural Networks, 20(3), 290-
297.
Brain-inspiredSensorimotorRoboticPlatform-LearninginCerebellum-drivenMovementTasksthroughaCerebellar
RealisticModel
573