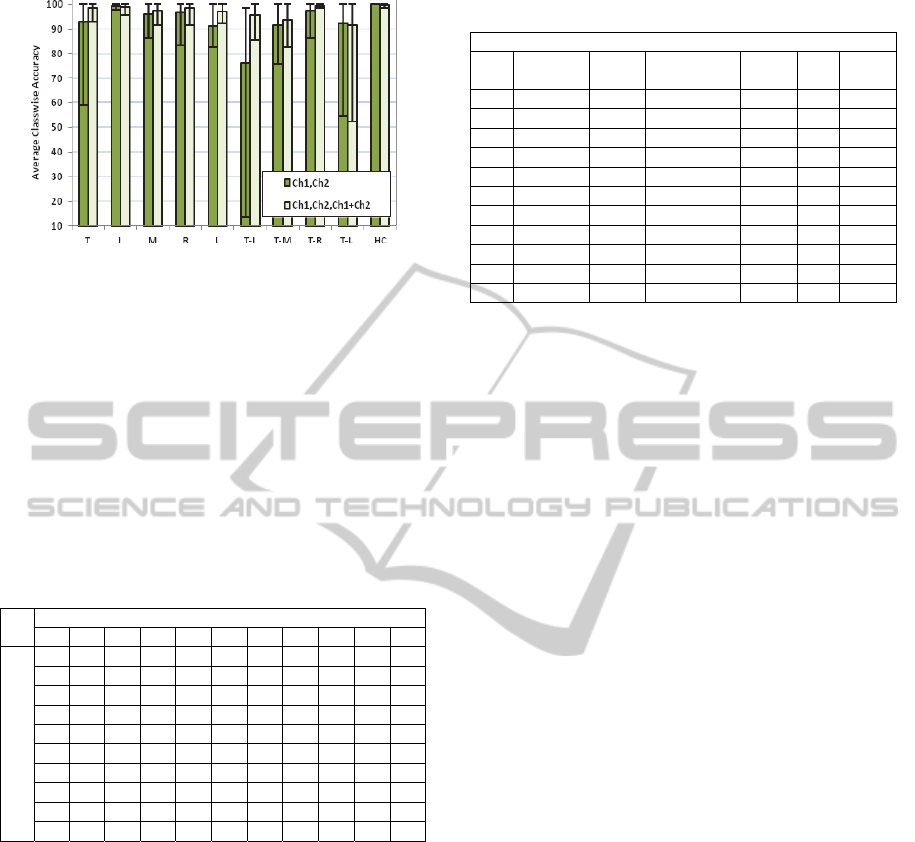
Figure 4: The online classification accuracy.
The online classification is the second
experiments performed. The individual and
combined finger movements were recognized in
real-time based on the matrix projection of SRDA
and the trained ELM kernel from offline stage. In
this experiments, the configuration of (ch1, ch2)
achieve 93.36 % accuracy while the (ch1,ch2,
ch1+ch2) configuration attained better accuracy
which is 97.07 %. The performance of finger
recognition is depicted in the fig.4 and the table 2.
Table 2: The confusion matrix of the classification results
averaged for SIX subjects.
Intended task (%)
T I M R L T-I T-M T-R T-L HC
Classified task (%)
T 98.7 0.1 0.0 0.0 0.0 0.9 0.0 0.0 0.0 0.3
I 0.0 99.3 0.0 0.0 0.0 0.3 0.0 0.0 0.4 0.0
M 0.0 0.0 98.0 0.2 0.0 0.0 1.3 0.0 0.0 0.5
R 0.0 0.0 0.0 99.9 0.0 0.0 0.0 0.1 0.0 0.0
L 1.1 0.0 0.0 0.0 97.2 0.6 0.0 0.0 0.1 1.0
T-I 0.5 0.0 0.0 0.0 1.5 95.1 1.0 0.0 1.9 0.0
T-M 0.0 0.0 0.9 0.0 0.7 1.3 96.1 0.0 0.1 1.0
T-R 0.0 0.0 0.0 0.0 0.0 0.3 0.5 99.1 0.0 0.0
T-L 0.0 0.1 0.0 0.0 6.0 0.3 0.7 0.5 92.3 0.0
HC 0.0 0.0 0.0 0.0 0.0 0.0 0.0 0.2 0.0 99.8
Figure 4 shows that the T-L movement is the most
difficult one to recognize. It was misclassified to the L
movements as seen in the confusion matrix table 2. It was
probably caused by the facts that the T-L was composed of
Thumb(T) and Little(L) finger movement therefore there
is possibility each movement affects the combined
movements.
Besides the classification performance, the
processing time of the real-time application has been
also tested which the result is presented in table 3.
The acquisition, filtering, feature extraction and
reduction, ELM and majority vote processing time
were record during the experiment. This recognition
system took 112.13 ms in average. It is verified that
processing time of this system is in between the
optimal processing time for real-time myoelectric
control, 100-125 ms, as suggested in (Farrell and
Weir, 2007).
Table 3: The processing time of the online experiment.
Processing time (ms)
Class Acquiring Filter
Extraction
/reduction
ELM Vote Total
T 100 3.9 7.6 0.5 0.1 112.1
I 100 3.5 7.2 0.5 0.1 111.3
M 100 3.5 7.3 0.5 0.1 111.4
R 100 3.6 7.4 0.5 0.1 111.6
L 100 3.7 7.6 0.6 0.1 111.9
T-I 100 3.5 7.3 0.5 0.1 111.4
T-M 100 3.6 7.5 0.5 0.1 111.7
T-R 100 3.6 7.6 0.6 0.1 111.8
T-L 100 3.5 7.3 0.5 0.1 111.4
HC 100 3.5 7.3 0.5 0.1 111.4
Avg 100 3.6 7.4 0.5 0.1 112.1
This promising result could be implemented to
the hand exoskeleton to recover the motor function
of the patients post stroke. It could move all
individual fingers and some combined movements.
However, it is aimed for finger extension only. In
addition, it would not work properly if the EMG
signal of the subject is very weak. Therefore, it
could be only applied to the partially paralyzed
subject.
Furthermore the proposed system could be
implemented to the prosthetic hand device. It is
promising because it used few electrodes which
enhance the user's comfort. However, it needs more
validation for amputee subjects.
4 CONCLUSIONS
The two channel sEMG signals were used in this
paper to recognize the ten individual and combined
finger movements. The extracting more feature from
summation of the signals from the two channels
improves the classification accuracy in both offline
and online classification system. By using this
combination, the recognition system was able to
achieve in average 97.96 % in offline and 97.07% in
online one. These results show the feasibility of the
proposed system in classifying ten different finger
movements.
REFERENCES
Al-Timemy, A. H., Bugmann, G., Escudero, J. & Outram,
N. 2013. Classification of Finger Movements for the
Dexterous Hand Prosthesis Control with Surface
Electromyography. Biomedical and Health
Informatics, IEEE Journal of, 17, 608-618.
Anam, K., Khushaba, R. & Al-Jumaily, A. 2013. Two-
Channel Surface Electromyography for Individual and
NEUROTECHNIX2013-InternationalCongressonNeurotechnology,ElectronicsandInformatics
222