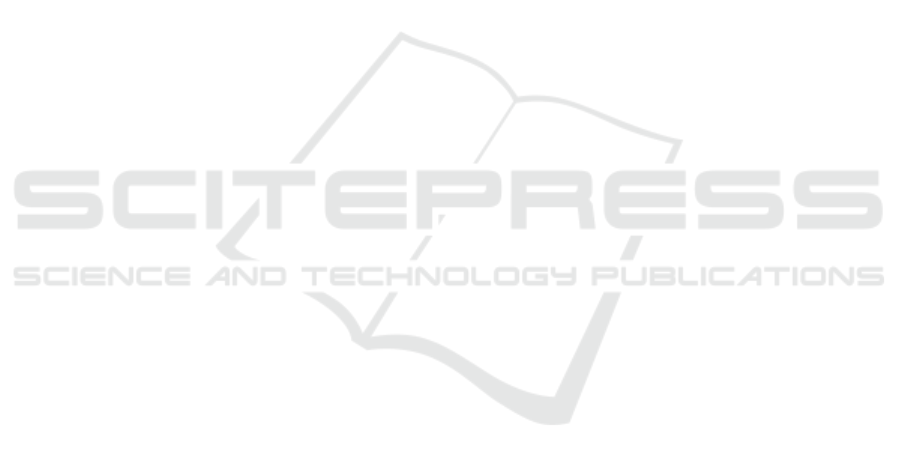
Computational Models of Populations of Motor Neurons
Jakob L. Dideriksen and Dario Farina
Department of Neurorehabiliation Engineering, Bernstein Focus Neurotechnology Göttingen,
Bernstein Center for Computational Neuroscience, Universitätsmedizin Göttingen, Göttingen, Germany
1 OBJECTIVES
In this paper, we will provide examples of how
computational models of motor neuron and muscle
activity can support basic and applied research on
human movement. Both examples focus on
pathological tremor. Tremor is a rhythmic,
involuntary oscillation of a limb and it is the most
prevalent movement disorder, symptomatic to e.g.
Parkinson’s Disease (Wenning et al., 2005). Tremor
implies a serious worsening of the quality of life,
also because the effect of the current treatments is
variable(Rascol et al., 2000).
First, we show how models can be applied in the
development of a rehabilitation device for
suppressing pathological tremor (Objective 1). The
proposed tremor rehabilitation system relied on
modulation of spinal neuron excitability of the
tremorogenic motor neurons using homonymous
excitation and reciprocal inhibition evoked by
electrical stimulation of peripheral type Ia nerves.
Successful implementation of this strategy implied
robust and precise on-line analysis of tremor
(Objective 1A) and stimulation parameter selection
(Objective 1B). Here, the Iterated Hilbert Transform
(IHT) applied to the surface EMG signals were
selected for tremor analysis (Dideriksen et al.,
2011).
Next, we demonstrate how models can enhance
the understanding of the underlying physiological
mechanisms of tremor (Objective 2), especially
aspects that cannotbe easily assessed experimentally.
Specifically, here we will address the contribution of
afferent feedback in tremor, which has been debated
in the literature (Rack and Ross 1986).
2 METHODS
The neuromechanical models applied to address the
two objectives consisted of a number of sub-models
and shared the same basic structure; however, the
level of model complexity required for addressing
each of the objectives determined how the various
sub-models were implemented.
2.1 Neuromechanical Models
The model was designed to reflect the characteristics
of an antagonist muscle pair acting on one limb in
one degree of freedom and consisted of a number of
sub-models interacting via one or more variables.
First, the activity of the motor neuron population
(spike trains) was determined based on the synaptic
input it received. Each motor neuron innervated a set
of muscle fibers (the motor unit). Each motor unit
was assigned a set of parameters describing its
contractile properties. Along with these properties,
the discharge rate determined the motor unit force.
The force of the muscles (the sum of the force
generated by all motor units) evoked the movement
of the limb. Proprioceptive activity was determined
by limb dynamics (muscle spindle; type Ia) and
muscle forces (Golgi tendon organs; type Ib) and
provided afferent feedback to the motor neurons.
Tremor was simulated by imposing a sine wave to
the motor neuron input.
For Objective 1A, a model of the motor unit
population and the force it generates was adopted
(Fuglevand et al., 1993), while the afferent feedback
was simulated as compound signals (Prochazka and
Gorassini, 1998). A model of the surface motor unit
action potentials(Farina et al., 2004) was used to
simulate the surfaceEMG signal (based on the motor
unit spike trains), that was used for tremor
estimation. For Objective 1B and 2 a more detailed
description of the single neuron behaviour (Cisi and
Kohn, 2008), a more advanced mechanical model
(Oguztoreli and Stein, 1982), as well as models
allowing the afferent feedback to be described as
spike trains (Mileusnic and Loeb, 2006) were
applied. In this way, simulation of axon action
potentials generated by surface stimulation was
made possible.
L. Dideriksen J. and Farina D..
Computational Models of Populations of Motor Neurons.
Copyright
c
2013 SCITEPRESS (Science and Technology Publications, Lda.)