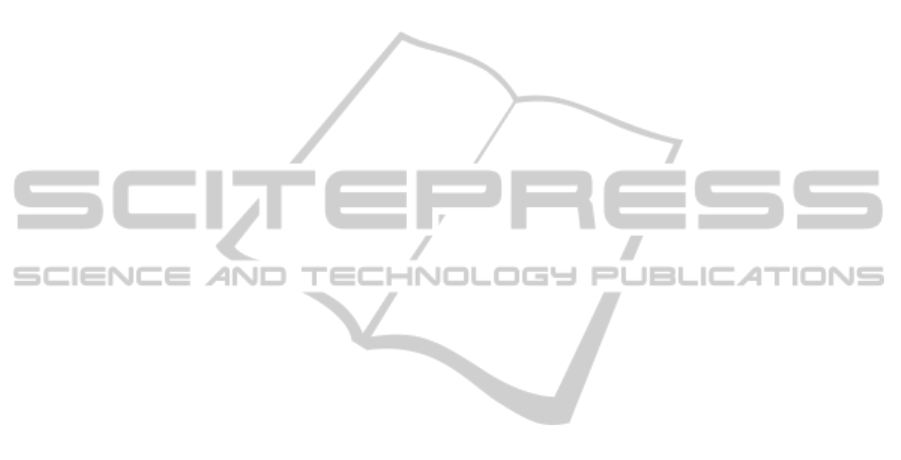
Correlation between tremor, voluntary contraction,
and firing pattern of motor units in Parkinson's
disease. In J Neurol Neurosurg Psychiatry. vol. 37(8).
pp. 927–937.
Farina, D., Holobar, A., Merletti, R., Enoka R.M., 2010
Decoding the neural drive to muscles from the surface
electromyogram. In Clin Neurophysiol. vol. 121. pp.
1616–1623.
Farina, D., Negro, F., Gazzoni, M., Enoka, R.M., 2008
Detecting the unique representation of motor-unit
action potentials in the surface electromyogram. In J.
Neurophysiol. vol. 100. pp. 1223–1233.
Gross, J., Kujala, J., Hamalainen, M., Timmermann, L.,
Schnitzler, A., Salmelin, R., 2001. Dynamic imaging
of coherent sources: Studying neural interactions in
the human brain. In Proc. Natl. Acad. Sci. USA 98. pp.
694-699.
Groznik, V. , Guid, M., Sadikov, A., Možina, M.,
Georgiev, D., Kragelj, V., Ribarič, S., Pirtošek, Z.,
Bratko, I., 2013. Elicitation of neurological knowledge
with argument-based machine learning. In Artificial
Intelligence in Medicine. vol. 57(2). pp. 133-44.
Halliday, D. M., Rosenberg, J. R., Amjad, A. M., Breeze,
P., Conway, B. A., Farmer, S. F., 1995. A framework
for the analysis of mixed time series/point process data
- theory and application to the study of physiological
tremor, single motor unit discharges and
electromyograms. In Prog Biophys Mol Biol. vol.
64, no. 2/3, pp. 237-278.
Holobar, A., Glaser, V., Gallego, J.A., Dideriksen, J.L.,
Farina, D., 2012. Non-invasive characterization of
motor unit behaviour in pathological tremor. In J
Neural Eng. vol. 9.
Holobar, A., Minetto, M. A., Botter, A., Negro, F., Farina
D., 2010. Experimental analysis of accuracy in the
identification of motor unit spike trains from high-
density surface EMG. In IEEE trans. neural syst.
rehabil. eng.vol. 18. no. 3. pp. 221-229.
Holobar, A., Minetto, M. A., Farina, D., 2013. A signal-
based approach for assessing the accuracy of high-
density surface EMG decomposition. Submitted to 6th
International IEEE/EMBS Conference on Neural
Engineering 2013.
Muthuraman, M., Heute, U., Arning, K., Anwar, A.R.,
Elble, R., Deuschl, G., Raethjen, J., 2012. Oscillating
central motor networks in pathological tremors and
voluntary movements. What makes the difference?. In
NeuroImage. vol. 60. pp. 1331–1339.
Muthuraman, M., Hossen, A., Heute, U., Deuschl, G.,
Raethjen, J., 2011. A New Diagnostic Test to
Distinguish Tremulous Parkinson’s Disease from
Advanced Essential Tremor. In Movement Disorders.
vol. 26. no. 8. pp. 1548-1552.
Raethjen, J., Govindan, R.B., Muthuraman, M., Kopper,
F., Volkmann, J., Deuschl, G., 2009. Cortical
correlates of the basic and first harmonic frequency of
Parkinsonian tremor. In Clin Neurophysiol. vol. 120.
pp.1866–1872.
Rocon, E., Andrade, A.O., Pons, J. L., Kyberd, P., Nasuto,
S. J., 2006. Empirical mode decomposition: a novel
technique for the study of tremor time series. In Med.
Biol. Engineering and Computing. vol. 44(7). pp. 569-
582 .
Saunders-Pullman, R., Derby, C., Stanley, K., Floyd, A.,
Bressman, S., Lipton, R.B., Deligtisch, A., Severt, L.,
Yu, Q., Kurtis, M., Pullman, S.L., 2008. Validity of
spiral analysis in early Parkinson's disease, In Mov
Disord. vol. (4). pp. 531-537.
Scheltera, B., Timmer, J., Eichler, M., 2009. Assessing the
strength of directed influences among neural signals
using renormalized partial directed coherence. In
Journal of Neuroscience Methods. vol. 179 (1). pp.
121–130.
Wu, Z., Huang, N.E., 2009. Ensemble Empirical Mode
Decomposition: a Noise-assisted Data Analysis
Method. In Adv Adapt Data Anal. vol. 1. pp. 1–41.
NEUROTECHNIX2013-InternationalCongressonNeurotechnology,ElectronicsandInformatics
132