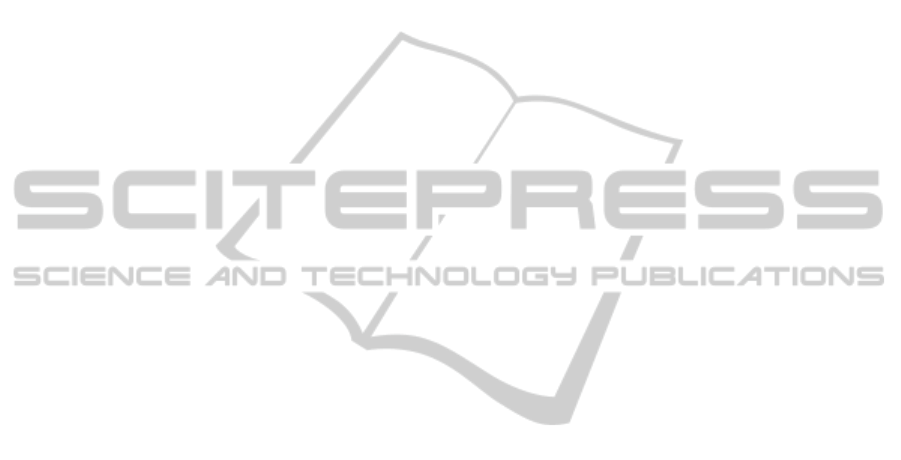
kind of terms of units are discovered (e.g. a tempera-
ture unit, a relative humidity unit, a permeability unit)
and allow an automatic integration of variant or new
units directly in the OTR.
7 CONCLUSIONS AND FUTURE
WORK
The paper presents our overall two-step approach in
order to identify variant terms of units or new units in
unstructured scientific documents. We have success-
fully demonstrated how supervised learning methods
can help reduce the search space of terms of units
drowned in textual information. We have achieved
almost 86% of reducing. For this purpose, we have
used many algorithms and weight-based measures to
prove the relevancy of our approach. We have also
presented our first results to detect and extract vari-
ant terms and new units, using a new string distance
measure adapted to our unit identification issue. As
presented in the paper, this second step needs to be
deepened. Our approach, presented in this paper, ad-
dresses the entire issue: location, extraction and iden-
tification of units. Further work on semantical identi-
fication of variant terms and new units should be ad-
dressed in order to automatically populate the OTR.
REFERENCES
Cohen, W. W., Ravikumar, P., and Fienberg, S. E. (2003).
A comparison of string distance metrics for name-
matching tasks. In Proc. of the Workshop on Informa-
tion Integration on the Web (IIWeb-03), volume 47.
Cunningham, H., Maynard, D., Bontcheva, K., and Tablan,
V. (2002). GATE: A framework and graphical de-
velopment environment for robust NLP tools and ap-
plications. In Proceedings of the 40th Annual Meet-
ing of the Association for Computational Linguistics,
Philadelphia, PA, USA.
Damerau, F. J. (1964). A technique for computer detec-
tion and correction of spelling errors. Commun. ACM,
7(3):171–176.
Hawizy, L., Jessop, D., Adams, N., and Murray-Rust, P.
(2011). ChemicalTagger: a tool for semantic text-
mining in chemistry. Journal of cheminformatics,
3(1):17.
Hiemstra, D. (2000). A probabilistic justification for using
tf x idf term weighting in information retrieval. Int. J.
on Digital Libraries, 3(2):131–139.
Hignette, G., Buche, P., Couvert, O., Dibie-Barth
´
elemy, J.,
Doussot, D., Haemmerl
´
e, O., Mettler, E., and Soler,
L. (2008). Semantic annotation of Web data applied
to risk in food. International Journal of Food Micro-
biology, 128(1):174–180.
Jessop, D. M., Adams, S. E., and Murray-Rust, P. (2011a).
Mining chemical information from open patents.
Journal of cheminformatics, 3(1):40.
Jessop, D. M., Adams, S. E., Willighagen, E. L., Hawizy,
L., and Murray-Rust, P. (2011b). OSCAR4: a flexi-
ble architecture for chemical text-mining. Journal of
cheminformatics, 3(1):1–12.
John, G. H. and Langley, P. (1995). Estimating continuous
distributions in bayesian classifiers. In Proc. of the
conf. on Uncertainty in artificial intelligence, pages
338–345.
Jones, K. S., Walker, S., and Robertson, S. E. (2000). A
probabilistic model of information retrieval: develop-
ment and comparative experiments - part 1. Inf. Pro-
cess. Manage., 36(6):779–808.
Kohavi, R. and Quinlan, J. R. (2002). Data mining tasks and
methods: Classification: decision-tree discovery. In
Handbook of data mining and knowledge discovery,
pages 267–276. Oxford University Press, Inc.
Maedche, A. and Staab, S. (2002). Measuring similar-
ity between ontologies. In Knowledge Engineering
and Knowledge Management: Ontologies and the Se-
mantic Web, volume 2473 of LNCS, pages 251–263.
Springer.
Maynard, D., Li, Y., and Peters, W. (2008). Nlp techniques
for term extraction and ontology population. In Pro-
ceeding of the 2008 conference on Ontology Learning
and Population: Bridging the Gap between Text and
Knowledge, page 107127.
Platt, J. C. (1999). Fast training of support vector machines
using sequential minimal optimization. In Advances
in kernel methods, pages 185–208. MIT Press.
Rijgersberg, H., van Assem, M., and Top, J. (2013). Ontol-
ogy of units of measure and related concepts. Seman-
tic Web.
Rijgersberg, H., Wigham, M., and Top, J. (2011). How se-
mantics can improve engineering processes: A case of
units of measure and quantities. Advanced Engineer-
ing Informatics, 25(2):276–287.
Su, J., Zhang, H., Ling, C. X., and Matwin, S. (2008). Dis-
criminative parameter learning for bayesian networks.
In Proc. of the int. conf. on Machine learning, pages
1016–1023.
Thompson, A. and Taylor, B. N. (2008). Guide for the use
of the international system of units (SI).
Touhami, R., Buche, P., Dibie-Barth
´
elemy, J., and Ibanescu,
L. (2011). An ontological and terminological resource
for n-ary relation annotation in web data tables. On
the Move to Meaningful Internet Systems: OTM 2011,
pages 662–679.
Van Assem, M., Rijgersberg, H., Wigham, M., and Top, J.
(2010). Converting and annotating quantitative data
tables. The Semantic Web–ISWC 2010, pages 16–31.
Willems, D. J., Rijgersberg, H., and Top, J. (2012). Identify-
ing and extracting quantitative data in annotated text.
SWAIE.
Wimalasuriya, D. C. and Dou, D. (2010). Ontology-based
information extraction: An introduction and a survey
of current approaches. Journal of Information Sci-
ence, 36(3):306323.
KDIR2013-InternationalConferenceonKnowledgeDiscoveryandInformationRetrieval
256