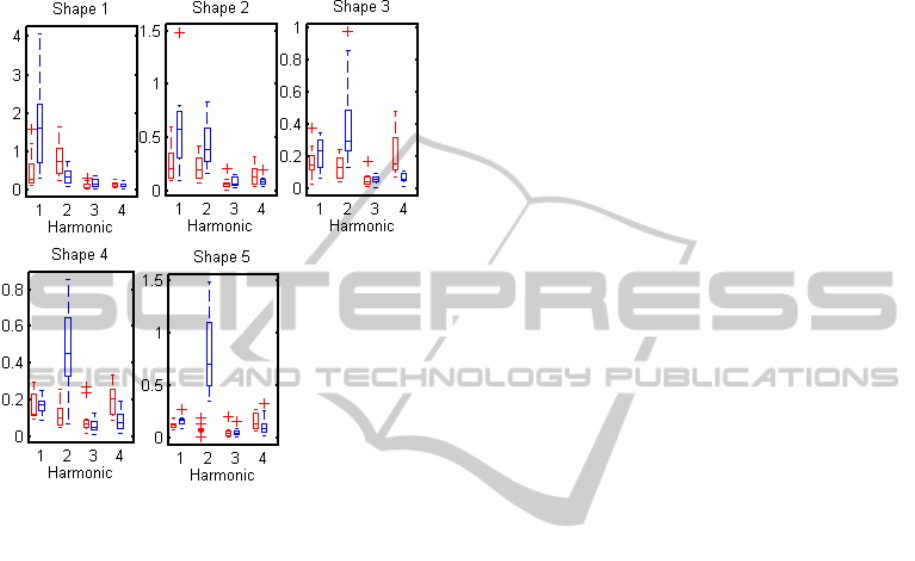
juxtaposition of commands represented by
rectangles together with commands represented by
checkerboards, but flickering at the same frequency
(thereby potentially doubling the number of possible
commands).
Figure 6: Fourier Power of the SSVEP responses to 30Hz
and 15Hz stimuli. 1/2/3/4 denote correspondingly the
frequencies 15Hz/30Hz/45Hz/60Hz (harmonics and
subharmonics of 30Hz stimulation may be observed at 15,
30 and 60Hz; and for 15Hz stimulation at 15, 30, 45 and
60Hz). Red boxes denote the Fourier powers in response
to a 30Hz stimulus; blue boxes denote the Fourier powers
in response to a 15Hz stimulus.
4 CONCLUSIONS
In this study, we observed that different size and
different type of shape of the stimuli change the
properties of SSVEP responses. The main
observation is the fact that a flickering checkerboard
elicits responses whose 2
nd
harmonic contain more
power than the first. This effect could be useful for
increasing the number of possible commands in
SSVEP brain-computer interfaces.
5 REFERENCES
Dawson, G. D., 1954. A summation technique for the
detection of small evoked potentials. Electroenceph.
Clin. Neurophysiol.1954; 6:65-84.
Foxe, J. J., Strugstad, E. C., Sehatpour, P., Molholm, S.,
Pasieka, W., Schroeder, C.E., McCourt, M.E. 2008.
Parvocellular and magnocellular contributions to the
initial generators of the visual evoked potential: high-
density electrical map-ping of the ‘‘C1’’ component.
Brain Topogr. 21 (1), 11–21.
Herrmann, C. S., 2001. Human EEG responses to 1–100
Hz flicker: resonance phenomena in visual cortex and
their potential correlation to cognitive phenomena.
Exp. Brain Res. 137 (3–4), 346–353.
McKeefry, D. J., Russell, M. H., Murray, I. J.,
Kulikowsky, J.J. 1996. Amplitude and phase
variations of harmonic components in human
achromatic and chromatic visual evoked
potentials.Vis. Neurosci. 13, 639–653.
Nicolelis, M. A-L., Wessberg, J., Stambaugh, C. R.,
Kralik, J. D., Beck, P. D., Laubach, M., Chapin, J. K.,
Kim, J., Biggs, S. J., Srinivasan, M. A. 2000. Real-
time prediction of hand trajectory by ensembles of
cortical neurons in primates. Nature 408 (6810): 361–
5.
Regan, D., 1989. Human Brain Electrophysiology: Evoked
Potentials and Evoked Magnetic Fields in Science and
Medicine. Elsevier, New York.
Silberstein, R. B., 1995. Steady-state visually evoked
potentials, brain resonances, and cognitive
processes.Nunez, P. L. (Ed.), Neocortical Dynamics
andHuman EEG Rhythms. Oxford University Press,
Oxford, pp. 272–303.
Tanaka, K., 1996. Inferotemporal cortex and object
vision.Annu. Rev. Neurosci. 19, 109–139.
Tanaka, K., 1997. Mechanisms of visual object
recognition: monkey and human studies. Curr. Opin.
Neurobiol. 7 (4), 523–529.
Thorey, J., Adibpour, P., Tomita, Y., Gaume, A.,
Bakardjian, H., Dreyfus, G., Vialatte, F.B. 2012. Fast
BCI calibration – Comparing methods to adapt BCI
systems for new subjects. Proc. NCTA 2012,
Barcelona, Spain. IJCCI (NCTA) 2012:663-669.
Vialatte, F. B., Maurice, M., Dauwels, J., Cichocki, A.
2010. Steady-State Visually Evoked Potentials: Focus
on Essential Paradigms and Future Perspectives.
Progress in Neurobiology, 90(4):418-438.
Vialatte, F. B., Maurice, M., Dauwels, J., Cichocki, A.
Steady State Visual Evoked Potentials in the Delta
Range (0.5-5 Hz). 15th International Conference on
Neural Information Processing, ICONIP, Auckland,
New Zealand, November 25-28 2008. ICONIP 2008,
LNCS, Part I, 5506:399–406, published in 2009.
Wang, Y., Wang, R., Gao, X., Hong, B., Gao, S., 2006. A
practical VEP-based brain–computer interface. IEEE
Trans. Neural Syst. Rehabil. Eng. 14 (2), 234–239.
EffectofStimulusSizeandShapeonSteady-StateVisuallyEvokedPotentialsforBrain-ComputerInterfaceOptimization
577