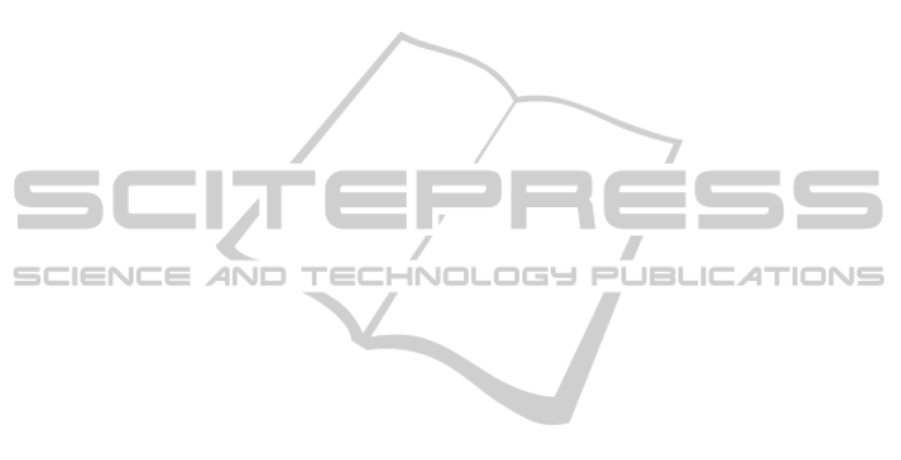
of games related to a drop in physical performance
or is it simply due to the team frequently chasing the
game and therefore taking more risks? Contextual
issues such as current form, changes in coaching and
playing staff, playing home or away as well as
opposition quality, playing style and team formation
and environmental conditions must also be
accounted for (Lago, 2009).
A practical question that frequently drives the
match analysis service is what are the key trends
‘statistically’ or the ‘key performance indicators’
that stand out (or not!) when winning, drawing or
losing games. While use of frequencies of key
performance indicators is common, analyses of
‘efficiency’ have more relevance from a practical
viewpoint (Hughes and Bartlett, 2002). For example,
a team might regularly create more scoring
occasions compared to other teams in the League but
the latter might have a better ratio of chances to
goals scored. Another question is based on the
relationship between key performance indicators and
the ability of teams to recover from a losing position
or maintain a winning position. For example, are
more points recovered by top compared to lower
ranked teams across the season after conceding a
goal first and which key match events can be
identified as having influenced the result (Carling et
al, in press)? Data comparing performance in home
versus away games or when playing against Top 6
teams or peers lower down in the table respectively
are pertinent. The former point can provide useful
indicators on how teams adapt according to game
location especially when a different playing style or
formation is employed. The latter can give an idea of
a team’s ability to ‘raise their game’ against the best
teams or ‘grind out’ results against peers fighting
relegation. It is also important not to simply
concentrate statistical analyses on attacking play and
neglect defensive performance. Distinguishing
physical performance in relation to team possession
(in other words during defensive or attacking play)
in particular can be helpful in understanding
differences in running distance (Bradley, Lago-
Peñas, Rey et al. in press). For example, is a wide-
midfielder but not a centre-forward working hard
‘physically’ off the ball to both create space for team
mates and to close down opposition players who are
in possession? Match analyses of technical
performance of a potential recruit can provide clues
as to how the player performs in relation to the
benchmark data of the player they might replace.
Data can also be exploited to identify potential
trends in performance across match periods (e.g.,
between halves or towards the end of games) in
order to judge a team’s or an individual player’s
ability to be ‘consistent’ over the course of play.
Similarly, the benchmarking of progress at different
milestones during the playing season is relevant (e.g.
first- versus second-half of season). This work can
be extended to investigate performance specifically
during periods of match congestion and determine a
team’s ability for example, to maintain standards in
domestic League matches directly after European
competition. The analyst might also track intra-
seasonal variations, for example, passing and
crossing completion rates over the first 5 games in
the present compared to the previous season.
Finally, information obtained from large-scale
match and motion analysis investigations can be
used to impact on the way teams generally train and
prepare for games. For instance, general exercise-to-
rest ratios or low- to high-intensity exercise ratios
calculated using time motion analysis data are used
to represent the physical demands of the game and
provide objective guidelines for optimising the
conditioning elements of training programmes
(Reilly, 2007). In addition, when statistical analyses
have enabled identification of a problematic issue,
the coaching staff can subsequently prescribe
adapted training drills and then reanalyse
performance to determine if there is improvement in
the frequency or efficiency of actions over following
games (Carling and Court, 2013). For example, the
effect of a modification in team formation on the
number of opportunities created for wide players to
cross the ball or a change in warm-up strategy to
improve high-intensity work rate in substitute
players immediately on entering play. Future work
will no doubt move towards the development of
intelligent systems to aid in the development of
optimum training prescriptions and predictive
modelling of ensuing performance using the
intelligence gathered from analyses of performance.
REFERENCES
Bradley, P. S., Lago-Peñas, C., Rey E., & Diaz, A. G.
(2013). The effect of high and low percentage ball
possession on physical and technical profiles in
English FA Premier League soccer matches. Journal
of Sports Sciences, in press.
Carling, C., & Court, M. (2012). Match & Motion
Analysis of Soccer. In M. Williams (Ed.), Science and
Soccer: Developing Elite Performers (pp 173-198).
London: Routledge.
Carling, C., Wright, C., Nelson, L., & Bradley, P.S.
(2013). Comment on ‘Performance analysis in
football: A critical review and implications for future