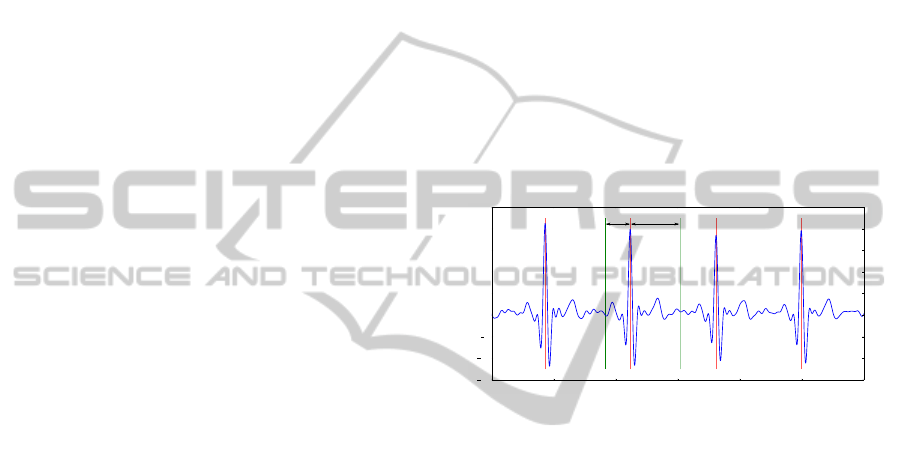
the subjects only partook in one of the sessions (sub-
jects S1, S3 and S6).
The Philips equipment was used in the standard
12-lead configuration (I-III, V1-V6, aVF, aVL, aVR),
as shown in Figure 1, with conductive gel applied to
each of the electrodes, which were placed on the sub-
jects by an experienced technician. It acquires sig-
nal data at 500 Hz, with 16 bit resolution, and storage
is made in a proprietary file format. Our sensor was
used in the virtual ground setting with dry Ag/AgCl
electrodes, and the subjects were told to hold the elec-
trodes at the hands. The BITalino device acquires sig-
nal data at 1000 Hz, with 10 bit resolution, and stor-
age is made in the open StorageBIT file format (Car-
reiras et al., 2013a). Given the fact that the Philips
system records only 10 seconds of data for each run,
we acquired three sequential runs, thus amounting to
30 seconds of data per subject per session.
The main application of our sensor has been to
ECG biometrics (Lourenc¸o et al., 2011; Silva et al.,
2013; Carreiras et al., 2013b), reason for which both
signals were filtered with a Finite Impulse Response
(FIR) bandpass filter with a Hamming window of
300ms, and cutoff frequencies of 5 − 20Hz. These
parameters were determined to be appropriate for this
specific application (Lourenc¸o et al., 2012).
Additionally, for the comparison measures we em-
ploy in this paper, it is necessary to have both signals
with the same sample rate. Therefore, and given the
characteristics of the filter just described, we down-
sampled the BITalino signal by a factor of 2, making
it match the 500 Hz sampling rate of the Philips de-
vice.
3 COMPARATIVE STUDY
We base our comparative study of the BITalino sen-
sor and the Philips system on two major approaches:
morphological and synchronized analyses. While the
first approach focuses on the shape similarity between
individual heartbeat waveforms, the second focuses
on analyzing the correlation between the signals after
a synchronization step, i.e. the correction of the time
delay between the two independent data acquisition
systems.
3.1 Morphological Analysis
In this paper, morphological analysis is understood to
be the comparison of the shape similarity between in-
dividual heartbeat waveforms, as measured by a spec-
ified metric. This requires the use of a QRS detection
algorithm, which enables the segmentation and ex-
traction of the individual heartbeat waveforms from
the ECG time series. For that purpose, we used the
modified Engzee algorithm described in (Lourenc¸o
et al., 2012), which identifies the positions of the R
peaks in the ECG. We then extract the signal seg-
ment corresponding to 200ms before and 400 ms after
each R peak, amounting to a total of 600ms, which
corresponds to the RR interval with a heart rate of
100bpm (see Figure 2). We applied this segmenta-
tion algorithm to the signals from both devices, col-
lecting, from each subject, all the identified heartbeat
waveforms across both sessions. Note that in the case
of the Philips device, segmentation was performed us-
ing the signal from lead I, using it as reference for the
other leads, i.e. the positions of the R peaks found
using lead I were used to extract the individual heart-
beats from the other leads.
10 .0 10 .5 11 .0
11 .5
12 .0 12 .5 13 .0
Tim e (s)
15 0
10 0
50
0
50
10 0
15 0
20 0
25 0
Am p lit u d e
200 ms 400 ms
Figure 2: Example of the segmentation and heartbeat wave-
form extraction procedure; the positions of the R peaks are
plotted in red vertical lines; the limits of one of the heart-
beats are shown in green.
Figure 3 shows the heartbeat waveforms obtained
for one of the subjects, comparing the BITalino heart-
beats with each of the leads from the Philips system.
We can observe that the most visually similar Philips
lead to the BITalino heartbeats is lead I, as intended
by the design of the sensor described in the previous
section, with an almost complete overlap between the
two. It is also possible to distinguish two groups of
heartbeats in the Figure, identifiable, for example, by
looking at the T waves. This is due to the fact that
the Figure shows the heartbeats from both acquisition
sessions, for which the subject happened to have dif-
ferent resting heart rates. As it is known (Simoons
and Hugenholtz, 1975), the heart rate influences the
relative position of the ECG waves, in particular the
T wave.
For the quantitative analysis, given the use of dif-
ferent electrode types and gains between the Philips
and BITalino devices, we adopted the cosine distance
as similarity metric (Equation 1), which is bounded
between 0 and 1, respectively representing complete
similarity (vectors with same orientation) and com-
plete dissimilarity (vectors at π/2).
ComparativeStudyofMedical-gradeandOff-the-PersonECGSystems
117