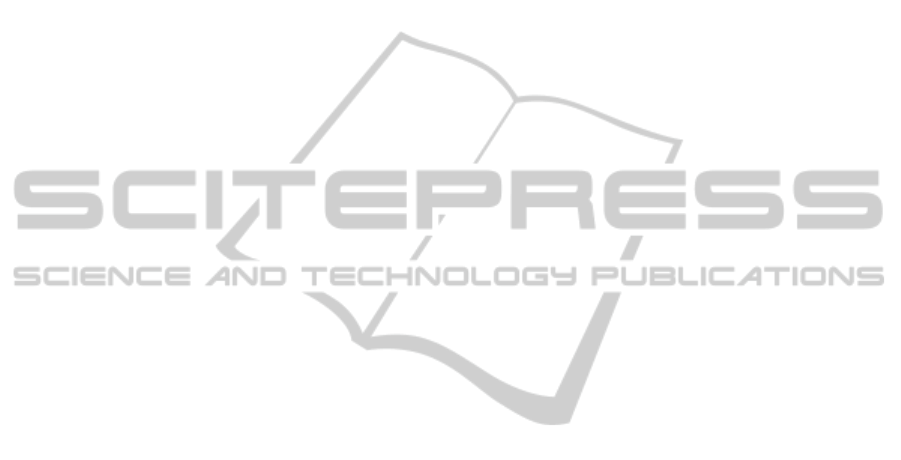
The influence of the frequency of the synaptic input
has been a traditional concern as to the usage of
metrics to assess motor unit synchronization using
the cross-correlograms (Nordstrom et al., 1992). A
recent study by Negro and Farina demonstrated that
such influence significantly distorts the results in
healthy subjects, based on simulation and
experimental data (Negro and Farina, 2012). The
authors of that paper showed that a method based on
the activities of several motor units provides a better,
unbiased indicator of the properties of common
synaptic input to motor neurons (Negro and Farina,
2012), as explained below.
2.3 Common Synaptic Inputs to Motor
Neurons
As previously mentioned, animal studies proved that
the presence of an input common to a population of
motor neurons increases the possibility of such units
firing synchronously (Kirkwood and Sears, 1978).
However, the estimation of this input is largely
influenced by the statistics of input current and the
discharge rate of the motor neurons (Negro and
Farina, 2012). Indeed, higher discharges rates imply
a better sampling of the input current, and thus allow
a better reconstruction.
Under the assumption that groups of motor unit
spike trains (referred to as composite spike trains)
increases the average sampling rate of the common
input to motor neurons (Negro and Farina, 2011,
2011b), Negro and Farina showed that the coherence
between such composite spike trains provides the
best estimate of the strength and frequency of
common synaptic inputs to motor neurons. Notice
that coherence is the normalized Fourier transform
of the cross-correlation function, and that it is
independent of filter functions.
Interestingly, traditional methods for the
estimation of motor unit synchronization (see
Section 2.2), and thus of common input properties,
consisted in applying certain filters to the cross-
correlogram (e.g., De Luca et al., 1982, Nordstrom
et al., 1992), which corresponds, in the frequency
domain, to considering a certain frequency band of
the coherence spectrum (Negro and Farina, 2012).
This clearly shows that such estimators are
intrinsically influenced by the input frequency.
2.4 Corticospinal Coupling
The projection of supraspinal, typically cortical,
oscillations to the muscle has been commonly
investigated by computing the coherence between
the supraspinal signal and the surface EMG. This
technique has allowed demonstrating the existence
of cortical involvement during different type of
muscle contractions in healthy subjects (Conway et
al., 1995, Raethjen et al., 2008), and also in the case
of tremor (Volkmann et al., 1996).
Remarkably, since coherence is a linear
technique, the existence of significant coherence
between the supraspinal signal and the EMG implies
that the transmission is partly linear, despite the non-
linearity of the motor neuron transfer function
(Gerstner and Kistler, 2002, Negro and Farina,
2011). However, due to the intrinsic limitations of
the surface EMG, it is not possible to gather further
insight on how such projection actually occurs.
A recent study showed that linearity of the
transmission can only be achieved if the supraspinal
inputs mediating voluntary contraction are a
common synaptic input to the motor neuron pool
(Negro and Farina, 2011). This was demonstrated
based on two facts. The first was that sampling a few
motor neurons already provided significant
coherence, which implies that a few motor neurons
are able of transmitting the cortical input. The
second observation was that the accuracy of such
estimation (magnitude of the coherence) did not
further increase after a few motor neurons were
sampled, which indicates to a saturation in sampling,
only possible in the case of common inputs.
The demonstration of the linearity of the
transmission also has physiological implications,
because due to the non-linear properties of
interneurons (Gerstner and Kistler, 2002), linear
transmission implies that direct pathways mediate
voluntary contractions. Thus, such finding further
supports the relevant role of the corticospinal tract in
voluntary movement control (Lemon, 2008).
3 CONCLUSIONS
In this paper we have reviewed traditional and novel
methods to assess motor unit properties and their
underlying determinants. These methods are
becoming increasingly important since they permit
to assess motor unit activities with an unprecedented
accuracy, thereby enabling advances in basic
neuroscience, muscle physiology and motor control,
thanks to a more accurate characterization of the
neural drive to muscle