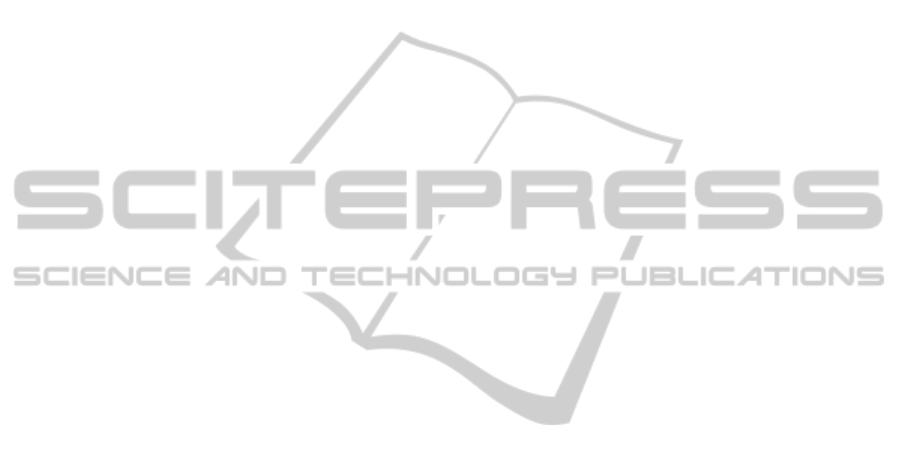
few studies in sleep apnea prediction. One of the
earliest work was published by Dagum and Galper
in 1995 (Dagum and Galper, 1995). This paper
developed a time series prediction by using belief
networks models and then this algorithm used in
sleep apnea. This paper used a multivariate data set
contains 34000 recordings, sampled at 2 Hz, of heart
rate (HR), chest volume (CV), blood oxygen con-
centration (SaO
2
), and sleep state from the time se-
ries competition of the Santa Fe Institute in 1991.
Based on this study, prediction with the CV signal
has more bias than HR and SaO2, because of rapid
and erratic oscillations of the CV series.
Another pioneer work in sleep apnea prediction,
we can can be found in Bock and Gough paper in
1998 . This study use 4.75 hz of heart rate, respira-
tion force, and blood oxygen saturation (SaO
2
) col-
lected from a chronic apnea patient. They use simple
recurrent networks (SRN) proposed by Elman
(Elman, 1991). Each of three time series variables
(heart rate, breathing, and blood oxygenation) were
used as inputs for network training and testing oper-
ations. Each variable was introduced to a unique
network node at the input layer; this network had 18
nodes in the hidden layer. «
One of the newest paper in this area is the work
of Waxaman, Graupe and Carley in 2010 (Waxman
et al., ). They predicted apnea 30 to 120 seconds in
advance. They use Large Memory Storage And Re-
trieval (LAMSTAR) neural network (Graupe and
Kordylewski, 1998). LAMSTAR is a supervised
neural network that can process large amount of data
and also provide detailed information about its deci-
sion making process. Input signals for this algorithm
are EEG, heart rate variability (HRV), nasal pres-
sure, oronasal temperature, submental EMG, and
electrooculography (EOG). It must be noted that
LAMSTAR has this ability to determine most im-
portant input (signal) in predicting process. In pre-
processing phase, data that segmented of 30, 60, 90
and 120 seconds was normalized. They trained sepa-
rate LAMSTAR for each 30, 60, 90 and 120 seconds
segment. Results show that best prediction belongs
to next 30 seconds and they obtained lower perfor-
mance for longer lead time, however, most of pre-
dictions up to 60 seconds in the future is correct.
Also, prediction of non-REM (NREM) events is
better than REM events, generally. For example, for
apnea prediction using 30-second segments and a
30-second lead time during NREM sleep, the sensi-
tivity was 80.6 ± 6 5.6%, the specificity was 72.78 ±
66.6%, the positive predictive values (PPV) was
75.16 ± 3.6%, and the negative predictive values
(NPV) was 79.4 ± 6 3.6%. REM apnea prediction
demonstrated a sensitivity of 69.36 ± 10.5%, a speci-
ficity of 67.46 ± 10.9%, a PPV of 67.4 6 ± 5.6%,
and an NPV of 68.8 ± 6 5.8%. Analyses also showed
that the most important signal for the predicting
apnea into the next second is submental EMG, and
RMS value of the first wavelet level is the most
important feature. But, for the 60 seconds prediction,
nasal pressure is most important signal.
This paper discusses the development of super-
vised artificial neural networks, Elman, cascade-
forward and feed-forward back propagation to pre-
dict sleep apnea. In the rest of this paper, the three
NNs are introduced. Then the issues related to net-
work design and training, especially how to avoid
over fitting, are addressed. The use of AUC as per-
formance measure of the models, and the statistical
comparison of the overall performance of the models
by means of cross-validation, are outlined. The re-
sults and conclusions are presented at the end of the
paper.
2 PRELIMINARIES
2.1 Elman Neural Networks
The Elman neural network is one kind of globally
feed-forward locally recurrent network model pro-
posed by Elman (Li et al., 2009). It occupies a set of
context nodes to store the internal states. Thus, it has
certain dynamic characteristics over static neural
networks, such as the Back-Propagation (BP) neural
network and radial-basis function networks. The
structure of an Elman neural network is illustrated in
Figure 1.
It is easy to observe that the Elman network con-
sists of four layers: input layer, hidden layer, context
layer, and output layer. There are adjustable weights
connecting every two adjacent layers. Generally, the
Elman neural network can be considered as a special
type of feed-forward neural network with additional
memory neurons and local feedback. The distinct
‘local connections’ of context nodes in the Elman
neural network make its output sensitive not only to
the current input data, but also to historical input
data, which is useful in time series prediction. The
training algorithm for the Elman neural network is
similar to the back-propagation learning algorithm, as
both based on the gradient descent principle. Howev-
er, the role that the context weights as well as initial
context node outputs play in the error back-
propagation procedure must be taken into considera-
tion in the derivation of this learning algorithm. Due
to its dynamical properties, the Elman neural network
ComparisonofNeuralNetworksforPredictionofSleepApnea
61