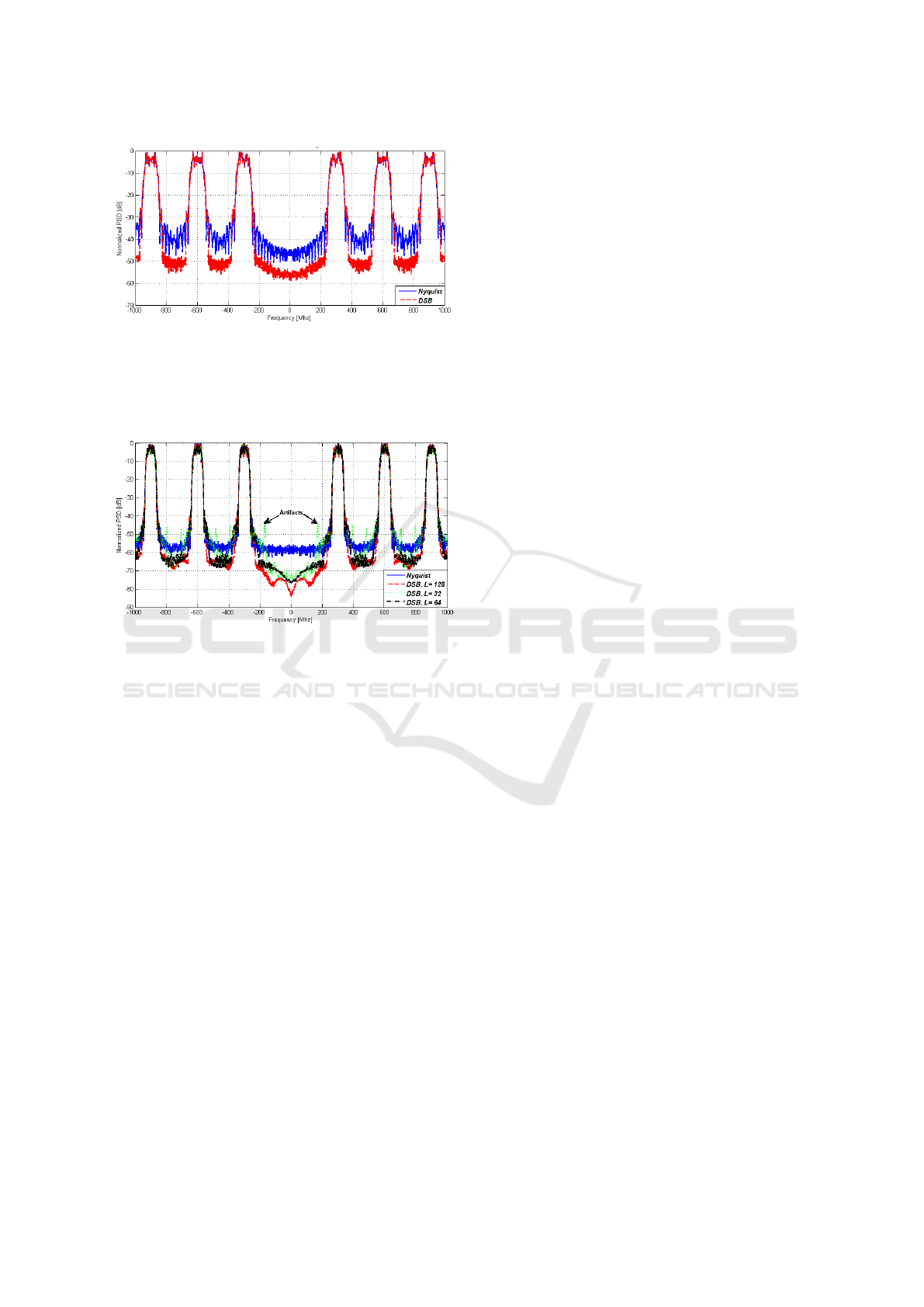
Figure 8: PSD with Lomb-Scargle method
input signal. From Figure 8, it can be seen that the
results obtained using DSB sampler are quite good.
Figure 9: Influence of the parameter L in the variation of the
PSD after DSB
Figure 9 shows the effect of the parameter L on
the estimated PSD from DSB samples. For L = 32,
spectral artifacts are observed in the estimated PSD,
as shown in Figure 9. But as L is increased these ar-
tifacts are eliminated. Therefore, it is clear that the
reconstructed spectrum is better when L increases.
6 CONCLUSIONS
In this paper we have presented some best known non-
uniform sampling techniques. JRS and ARS mode
gives approximately the same PSD without spectral
replicas but with a much smaller dynamic than Uni-
form samples PSD. These two techniques can be used
for spectrum sensing, condition not having a large dif-
ference in power between the bands. We dissassed
about a new sampler based on Multi-Coset sampling
scheme, which adjusts its sampling rate according to
the changes in the frequency spectrum of the input
signal. That is, if the signal is sparse in the frequency
domain, it samples at sub-nyquist rate, else it samples
at nyquist rate. We also saw the effect of the parame-
ter L on the estimated PSD from DSB samples.
ACKNOWLEDGEMENTS
This work is supported by the French “Region Bretagne”
for the projects “FUI AMBRUN” and “PME SoftRF”.
REFERENCES
Ben Romdhane, M. (2009). chantillonnage non uniforme
appliqu la numrisation des signaux radio multistan-
dard. PhD thesis.
Bourdoux A., Pollin S., D. A. and der Perre L., V. (2011).
Sparse signal sensing with non-uniform undersam-
pling and frequency excision. pages 246–250.
Bresler, Y. (2008). Spectrum-blind sampling and compres-
sive sensing for continuous-index signals. Informa-
tion Theory and Applications Workshop, 2008, page
547554.
Feng, P. and Bresler, Y. (1996). Spectrum-blind minimum-
rate sampling and reconstruction of multiband signals.
ICASSP, 3:16881691.
Landau, H. (1967). Necessary density conditions for sam-
pling and interpolation of certain entire functions.
Acta Mathematica, 117(1):37–52.
Miar, Y., D’Amours, C., and Aboulnasr, T. (2012). A
novel reduced power compressive sensing technique
for wideband cognitive radio. EURASIP J. Wireless
Comm. and Networking, page 281.
Mishali, M. and Eldar, Y. C. (2010). From theory to prac-
tice: Sub-nyquist sampling of sparse wideband analog
signals. Selected Topics in Signal Processing, IEEE
Journal of, 4(2):375–391.
Palicot, J. and al (June 2010). Radio Engineering: from
Software Radio to Cognitive Radio. John Wiley and
Sons.
Rashidi Avendi, M. (2010). Non-uniform sampling and re-
construction of multi-band signals and its application
in wideband spectrum sensing of cognitive radio.
Shapiro, H. S. and Silverman, R. A. (1960). Alias-free sam-
pling of random noise. Journal of the Society for In-
dustrial & Applied Mathematics, 8(2):225–248.
Tian, Z. and Giannakis, G. (2006). A wavelet approach to
wideband spectrum sensing for cognitive radios.
Tibshirani, R. (1996). Regression shrinkage and selection
via the lasso. Journal of the Royal Statistical Society.
Series B (Methodological), page 267288.
Traor
´
e, S., Aziz, B., and Guennec, D. L. (2013). Dynamic
single branch non-uniform sampler. DSP, Santoriri,
Greece, International Conference on Digital Signal
Processing.
Venkataramani, R. and Bresler, Y. (2000). Perfect recon-
struction formulas and bounds on aliasing error in
sub-nyquist nonuniform sampling of multiband sig-
nals. Information Theory, IEEE Transactions on,
46(6):21732183.
Venkataramani, R. and Bresler, Y. (2001). Optimal sub-
nyquist nonuniform sampling and reconstruction for
multiband signals. Signal Processing, IEEE Transac-
tions on, 49(10):23012313.
Wojtiuk, J. J. (2000). Randomised sampling for radio de-
sign. PhD thesis, Citeseer.
Zhang, H. (2010). Filter bank multicarrier (fbmc) for cogni-
tive radio systems. These de doctorat, Conservatoire
National des Arts et Metiers et Wuhan Universite.
Non-Uniform Sampling for Spectral Analysis of Multi-Band Signals