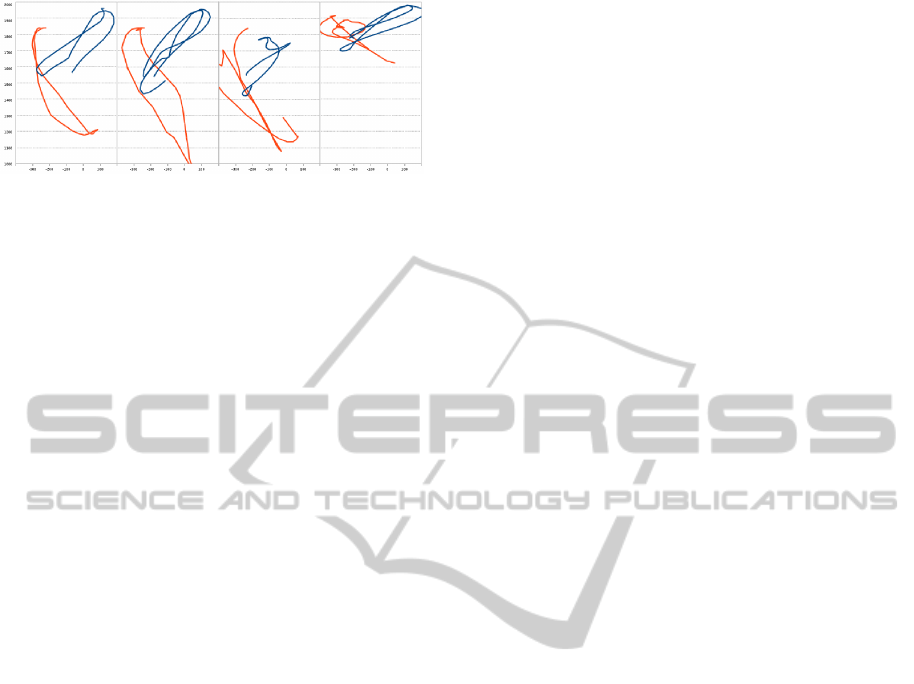
Figure 10: Trajectories of the moving wrist for the two per-
sons on our estimation projected in the XZ plane during the
four quarters of the sequence S
3
. The two arms often over-
lap in the recorded 2D image but they are correctly tracked
in the 3D space.
turbs the movement estimation of each target. Con-
sequently, we have displayed the evolution over time
of the 3D location of the wrists (well-descriptive of
the arm movement) of the two persons in sequence S
3
(figures 9 and 10). We notice that the method keep
tracking the arm regularly in case of occlusion and
that the two trajectories have independant evolutions.
5 CONCLUSIONS
In this paper we have proposed a new 3D track-
ing method based on the well known particle filter
method. To be efficient in the particular case of the
top view, the new Asus camera records the depth and
color cue. Then we have introduced a particle filtering
where the elements of the state vector are weighted
separately but linked by the complete 3D model. Ex-
perimental results show that this part-based process
improves the efficiency of the tracking. The resulting
application is real time and works for multi-person
tracking by the application of the exclusion principle.
The color of the skin visible for the hands is well
descriptive of the human class. The detection of the
hands could constraint the model in future works and
reduce the number of degrees of freedom. Then, the
tracking is only the first step of the human behav-
ior. Our method could be introduced in an action
recognition process. A camera pose estimation (Di-
dier et al., 2008; Ababsa and Mallem, 2008; Ababsa,
2009) could insert our work in a Augmented Real-
ity context with a moving camera. Finally a coupled
tracking and segmentation method would give more
information for the following of the processing and
prevent wrong estimations of each of the two treat-
ments.
REFERENCES
Ababsa, F. (2009). Robust extended kalman filtering for
camera pose tracking using 2d to 3d lines correspon-
dences. IEEE/ASME Conference on Advanced Intelli-
gent Mechatronics, pages 1834–1838.
Ababsa, F. and Mallem, M. (2008). A robust circular
fiducial detection technique and real-time 3d cam-
era tracking. International Journal of Multimedia,
3(4):34–41.
Bray, M., Koller-Meier, E., and Van Gool, L. (2004). Smart
particle filtering for 3d hand tracking. IEEE Inter-
national Conference on Automatic Face and Gesture
Recognition, pages 675–680.
Breitenstein, M., Reichlin, F., Leibe, B., Koller-Meier,
E., and Van Gool, L. (2011). Online multiper-
son tracking-by-detection from a single, uncalibrated
camera. IEEE Transaction on Pattern Analysis and
Machine Intelligence, XXXIII:1820–1833.
Brox, T., Rosenhahn, B., Gall, J., and Cremers, D. (2010).
Combined region and motion-based 3d tracking of
rigid and articulated objects. IEEE Transaction on
Pattern Analysis and Machine Intelligence, pages
402–415.
Cai, Y., De Freitas, N., and Little, J. (2006). Robust visual
tracking for multiple targets. European Conference on
Computer Vision, pages 107–118.
Canton-Ferrer, C., Salvador, J., Casas, J. R., and Pard
`
as,
M. (2008). Multi-person tracking strategies based on
voxel analysis. Multimodal Technologies for Percep-
tion of Humans, pages 91–103.
Deutscher, J. and Reid, I. (2005). Articulated body motion
capture by stochastic search. International Journal of
Computer Vision, 2:185–205.
Didier, J., Ababsa, F., and Mallem, M. (2008.). Hybrid
camera pose estimation combining square fiducials
localisation technique and orthogonal iteration algo-
rithm. International Journal of Image and Graphics,
8(1):169–188.
Escalera, S. (2012). Human behavior analysis from depth
maps. In International Conference on Articulated Mo-
tion and Deformable Objects, pages 282–292.
Gonzalez, M. and Collet, C. (2011). Robust body parts
tracking using particle filter and dynamic template. In
IEEE International Conference on Image Processing,
pages 529 – 532.
Hauberg, S., Sommer, S., and Pedersen, K. S. (2010).
Gaussian-like spatial priors for articulated tracking. In
European Conference on Computer Vision, pages 425
– 437.
Horaud, R., Niskanen, M., Dewaele, G., and Boyer, E.
(2009). Human motion tracking by registering anartic-
ulated surface to 3d points and normals. IEEE Trans-
action on Pattern Analysis and Machine Intelligence,
XXXI:158–163.
Isard, M. and Blake, A. (1998a). Condensation - conditional
density propagation for visual tracking. International
Journal of Computer Vision, XXIX:5 – 28.
Isard, M. and Blake, A. (1998b). Icondensation - unifying
Part-based3DMulti-personTrackingusingDepthCueinaTopView
425