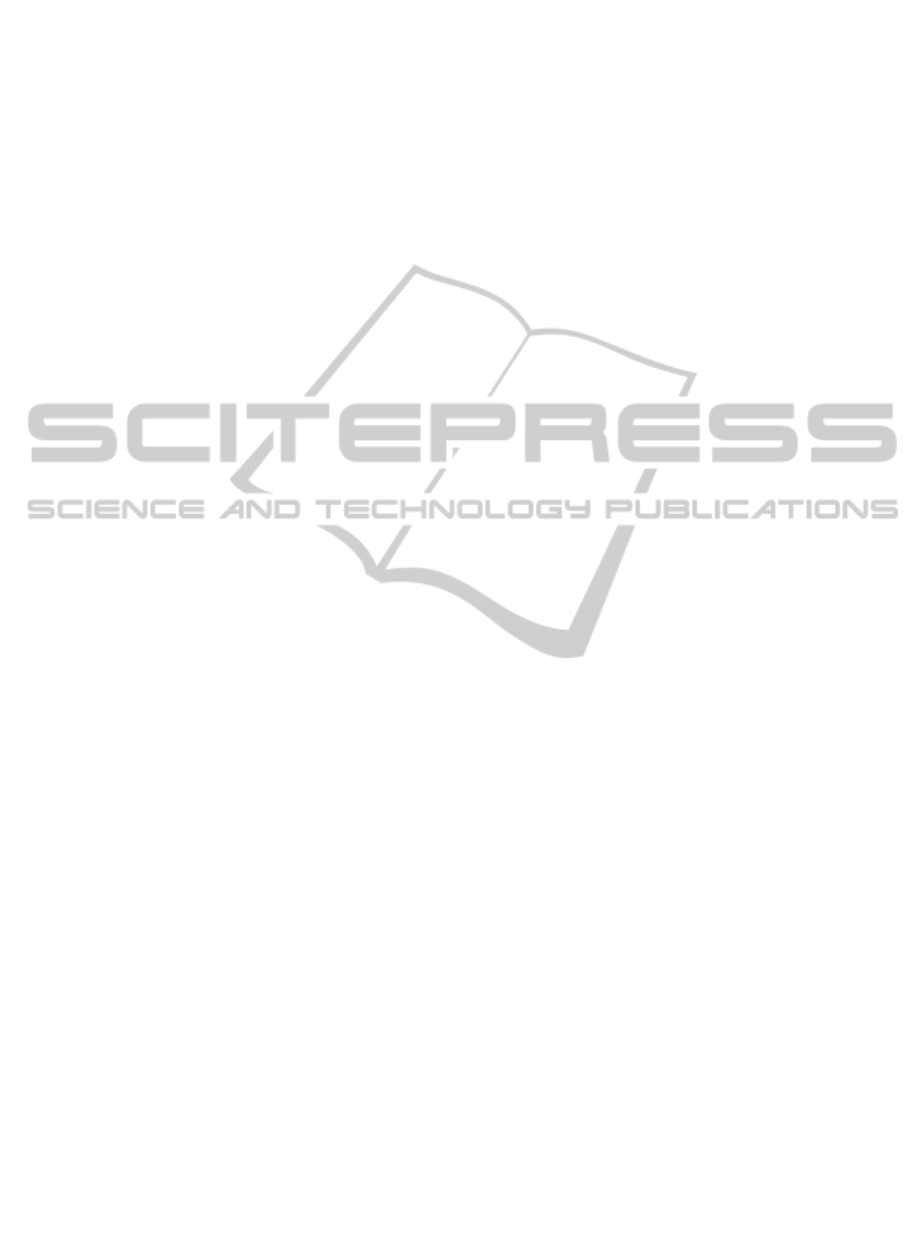
values were high as the amount of noise was very
little. The highest value obtained was by LET
(99.89%). Vesselness filtered image was much
similar to the expected result and it obtained the best
accuracy (99.78%) and precision (
74.68%).
4 CONCLUSIONS
We have reviewed and analysed a number of vessel
enhancement and segmentation algorithms using
both 2D and 3D image. Vesselness filter can be used
to detect vessels of varying scales. A potential
application of this method is to extract the brain
microvasculature and compare healthy and diseased
brains. LET has produced the highest sensitivity in
2D experiment but this method is recommended
only when the vessels are large and on a simple
background. Although WTMM
and level set method
failed the performance tests, they are capable of
detecting edges of large objects, such as brain
tumours. The main issue in this work is that the
performance test was not technically accurate due to
the poorly made ground truth and insufficient test
images so the 3D segmentation result has not been
100% validated. For further work we aim to produce
valid ground truth images for testing segmentation
algorithms. We will also continue to develop robust
wavelet filters and in combination with other
mathematical methods and metrics such as high-
order flows (Lim et al, 2013) non-Euclidean distance
functions (Pujadas et al, 2013) for handling
multiscale vessels and improving segmentation
speed and accuracy for microvascular analysis
(Ward et al, 2013).
ACKNOWLEDGEMENTS
We would like to thank S. Nakagawa and the late
Terry Parker in Biomedical Sciences; Lee Buttery
and Lisa White in Biomedical Sciences, University
of Nottingham, UK for providing the 3D images.
REFERENCES
Bedford, L., Hay, D., Devoy, A., Paine, S., Powe, D. G.,
Seth, R. & Mayer, R. J. (2008). Depletion of 26S
proteasomes in mouse brain neurons causes
neurodegeneration and Lewy-like inclusions
resembling human pale bodies. The Journal of
Neuroscience, 28(33), 8189-8198.
Chanwimaluang, T., & Fan, G. (2003, May). An efficient
blood vessel detection algorithm for retinal images
using local entropy thresholding. In Circuits and
Systems, 2003. ISCAS'03. Proceedings of the 2003
International Symposium on (Vol. 5, pp. V-21). IEEE.
Daugman, J. G. (1988). Complete discrete 2-D Gabor
transforms by neural networks for image analysis and
compression. Acoustics, Speech and Signal Processing,
IEEE Transactions on, 36(7), 1169-1179.
Ding, Y., Ward, W.O.C., Parker, T., Nakagawa, S.,
Buttery, L., White, L., Bai, L., (2013). Segmentation
of Mouse Brain Microvasculature from Micro-CT
Images Using Gabor filter and Local Entropy
Thresholding, International Symposium on Cerebral
Blood Flow, Metabolism and Function.
Frangi, A. F., Niessen, W. J., Vincken, K. L., & Viergever,
M. A. (1998). Multiscale vessel enhancement filtering.
In Medical Image Computing and Computer-Assisted
Interventation—MICCAI’98 (pp. 130-137). Springer
Berlin Heidelberg.
Fraz, M. M., Remagnino, P., Hoppe, A., Uyyanonvara, B.,
Rudnicka, A. R., Owen, C. G., & Barman, S. A.
(2012). Blood vessel segmentation methodologies in
retinal images–A survey. Computer methods and
programs in biomedicine.
Lathen, G., Jonasson, J., & Borga, M. (2008). Phase based
level set segmentation of blood vessels. In 19th IEEE
International Conference on Pattern Recognition.
Lim, P. L., Bagci, U., Bai, L. (2013). Introducing Wilmore
Flow into Level Set Segmentation of Spinal Vertebrae,
IEEE Transactions on Biomedical Engineering, Vol. 60,
No. 1.
Mallat, S., & Zhong, S. (1992). Characterization of signals
from multiscale edges. IEEE Transactions on pattern
analysis and machine intelligence, 14(7), 710-732.
Osher, S., & Sethian, J. A. (1988). Fronts propagating with
curvature-dependent speed: algorithms based on
Hamilton-Jacobi formulations. Journal of
computational physics, 79(1), 12-49.
Pal, N. R., & Pal, S. K. (1989). Entropic thresholding.
Signal processing, 16(2), 97-108.
Pająk, R. (2003). Use of two-dimensional matched filters
for estimating a length of blood vessels newly created
in angiogenesis process. Opto-Electronics Review,
11(3), 237-241.
Pujadas, E. R., & Bai, L. (2013). Non-Euclidean basis
function based level set segmentation with statistical
shape prior, IEEE EMBC2013, Osaka, Japan.
Sato, Y., Nakajima, S., Shiraga, N., Atsumi, H., Yoshida,
S., Koller, T., & Kikinis, R. (1998). Three-
dimensional multi-scale line filter for segmentation
and visualization of curvilinear structures in medical
images. Medical image analysis, 2(2), 143-168.
Staal, J., Abràmoff, M. D., Niemeijer, M., Viergever, M.
A., & van Ginneken, B. (2004). Ridge-based vessel
segmentation in color images of the retina. Medical
Imaging, IEEE Transactions on, 23(4), 501-509.
Rorden, C., Karnath, H. O., & Bonilha, L. (2007).
Improving lesion-symptom mapping. Journal of
cognitive neuroscience, 19(7), 1081-1088.
Ward, W., & Bai, L., (2013). Multifractal Analysis of
Microvasculature in Health and Diseases, IEEE
EMBC2013, Osaka, Japan.
VISAPP2014-InternationalConferenceonComputerVisionTheoryandApplications
432