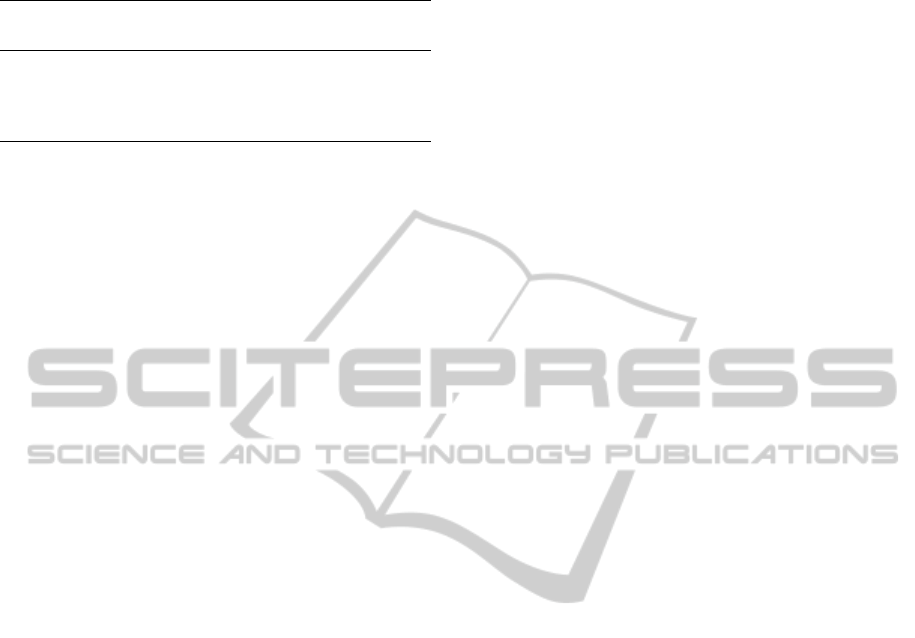
Table 3: Dropped Object detection results in Paris metro
dataset for n
s
= 90 seconds.
Method Footbridge Platform
Prec. Rec. Prec. Rec.
ML-DOD 0.43 1 0.03 1
ML-DOD + Geometry 1 1 0.2 1
ML-DOD-HD 0.43 1 0.04 1
ML-DOD-HD + Geometry 1 1 0.5 1
4 objects are annotated in both videos (3 objects on
the first video and 1 on the second).
ML-DOD is run with all the possible configura-
tions and α
w
∈ [0.02, 0.05], n
s
= 90 seconds (similarly
to Torino, some objects are removed just after 2 min-
utes, thus requiring extra time for triggering alarms
robustly). Since larger n
s
are targeted, lower α
w
val-
ues are chosen. Regarding the geometric constraints,
we allow objects of maximum width and height of 80
cm. As in the Torino experiments, we report the best
precision at maximum recall ( see Table 3).
This dataset serves to evaluate the ability of the
proposed approach to deal with scenes where people
normally stay still, such as the metro platform (see
Fig. 6). Both the geometry constraints and the filter-
ing of humans are very important in order to success-
fully deal with these cases. Clearly, in an area where
people is transiting, human filtering does not help in
increasing the precision (see Table 3). On the con-
trary, it gives a performance boost on the platform.
While the proposed approach is generally very ac-
curate in estimating the set down time, it predicts one
of the dropping times almost 1 minute in advance,
hence yielding an average of 11.5±9.9 seconds. Such
a failure is caused by mode creation times generated
by the owner (who remains in the same position be-
fore dropping the object). The average set down time
error for the remaining cases is 1.4 ± 1.2 seconds.
5 CONCLUSIONS
We have presented an automatic approach for detect-
ing dropped objects in surveillance scenarios. Our
method leverages on multiple elements to address this
problem. First, objects are characterized by their ap-
pearance and temporal statistics that allow to accu-
rately retrieve the dropping time. Secondly, an effi-
cient implementation of a set of position and size con-
straints that allow removing a number of false alarms.
Finally, the proposed approach leverages on state-of-
the-art detectors to filters spurious objects produced
by known classes such as still humans. Experimen-
tal results conducted on several datasets show the ef-
fectiveness of our approach that, in addition, runs in
real-time.
ACKNOWLEDGEMENTS
This work was supported by the Integrated Project
VANAHEIM (248907) of the European Union under
the 7th framework program.
REFERENCES
Caro Campos, L., SanMiguel, J., and Martinez, J. (30 2011-
Sept. 2). Discrimination of abandoned and stolen ob-
ject based on active contours. In AVSS 2011, pages
101–106.
Comaniciu, D. and Meer, P. (2002). Mean shift: a ro-
bust approach toward feature space analysis. TPAMI,
24(5):603–619.
Dalal, N. and Triggs, B. (June). Histograms of oriented gra-
dients for human detection. In CVPR 2005, volume 1,
pages 886–893 vol. 1.
Dubout, C. and Fleuret, F. (2012). Exact acceleration of
linear object detectors. In ECCV 2012, pages 301–
311, Berlin, Heidelberg. Springer-Verlag.
Fan, Q. and Pankanti, S. (30 2011-Sept. 2). Modeling of
temporarily static objects for robust abandoned object
detection in urban surveillance. In AVSS 2011, pages
36–41.
Fan, Q. and Pankanti, S. (Sept.). Robust foreground and
abandonment analysis for large-scale abandoned ob-
ject detection in complex surveillance videos. In AVSS
2012, pages 58–63.
Felzenszwalb, P., Girshick, R., McAllester, D., and Ra-
manan, D. (2010). Object detection with discrimina-
tively trained part-based models. TPAMI, 32(9):1627
–1645.
Heikkila, M. and Pietikainen, M. (April 2006). A texture-
based method for modeling the background and de-
tecting moving objects. TPAMI, 28(4):657–662.
INRIA. http://pascal.inrialpes.fr/data/human/.
Liao, H.-H., Chang, J.-Y., and Chen, L.-G. (Sept.). A lo-
calized approach to abandoned luggage detection with
foreground-mask sampling. In AVSS 2008, pages 132–
139.
PETS 2006. http://www.cvg.rdg.ac.uk/PETS2006/data.html.
Smith, K. C., Quelhas, P., and Gatica-Perez, D. (2006). De-
tecting abandoned luggage items in a public space. In
IEEE PETS.
Tian, Y., Feris, R., Liu, H., Hampapur, A., and Sun, M.-T.
(Sept. 2011). Robust detection of abandoned and re-
moved objects in complex surveillance videos. TSMC-
C, 41(5):565–576.
Yao, J. and Odobez, J.-M. (2007). Multi-layer background
subtraction based on color and texture. In CVPR 2007,
pages 1–8.
ExploitingSceneCuesforDroppedObjectDetection
21