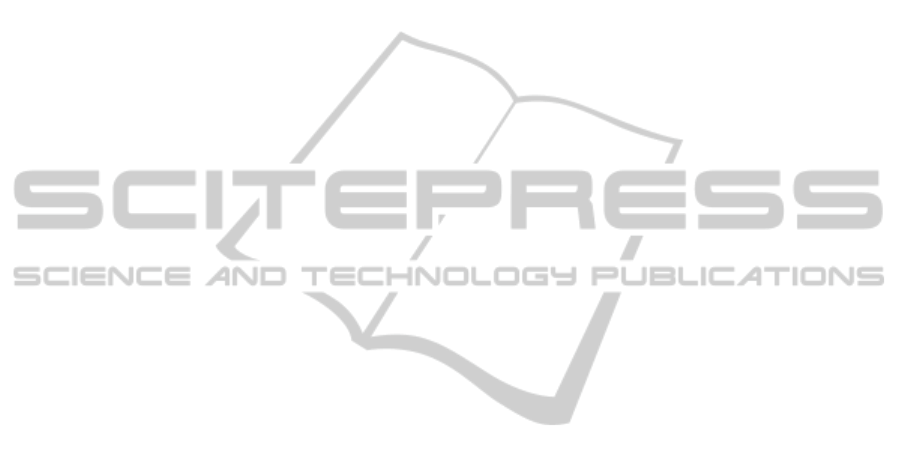
order features (bag-of-visual words i.e. H
1
). There-
fore, the performance of the 2
nd
order HOPR is better
than the bag-of-visual words. However, when we in-
crease the relationships feature order to 3 or 4, the
performance decreases. This can be explained by the
fact that 3
rd
and 4
th
order features are more sparse
than 2
nd
order features and hence, statistically less re-
liable.
5 CONCLUSIONS AND FUTURE
WORKS
We present a novel approach to egocentric video ac-
tivity representation based on the relationships be-
tween visual words. These pairwise relations are
encoded using Histogram of Oriented Pairwise Re-
lations (HOPR). The movement and interaction be-
tween objects and hands are captured by observing
the spatial relationships between features in video
frames. This representation does not require the de-
tection of objects or hands in comparison to other
common approaches. In addition, it can be used for
real-time activity detection which requires the recog-
nition of partial observations i.e. single frame to
few frames. In this work using egocentric data, we
show that by encoding the spatiotemporal relation-
ships between local features in activity representa-
tions improves performance over state-of-the-art ac-
tivity representation approaches such as the bag-of-
visual words. We would like to further investigate on
the hierarchical relationships structure using local vi-
sual features.
ACKNOWLEDGEMENTS
This research work is funded by the EU FP7-
ICT-248290 (ICT Cognitive Systems and Robotics)
grant COGNITO (www.ict-cognito.org), FP7-ICT-
287752 grant RACE (http://project-race.eu/) and FP7-
ICT-600623 grant STRANDS (http://www.strands-
project.eu/).
REFERENCES
Aggarwal, J. K. and Ryoo, M. S. (2011). Human activity
analysis: A review. ACM Comput. Surv., 43(3):1–16.
Aghazadeh, O., Sullivan, J., and Carlsson, S. (2011). Nov-
elty detection from an ego-centric perspective. In
CVPR, pages 3297–3304.
Allen, J. F. (1983). Maintaining knowledge about temporal
intervals. Commun. ACM, 26(11):832–843.
Balbiani, P., Condotta, J.-F., and del Cerro, L. F. (1999).
A new tractable subclass of the rectangle algebra. In
Proceedings of the 16th International Joint Confer-
ence on Artificial Intelligence (IJCAI), pages 442–
447.
Bay, H., Tuytelaars, T., and Gool, L. V. (2006). SURF:
Speeded up robust features. In ECCV, pages 404–417.
Behera, A., Cohn, A. G., and Hogg, D. C. (2012a). Work-
flow activity monitoring using dynamics of pair-wise
qualitative spatial relations. In MMM, pages 196–209.
Behera, A., Hogg, D. C., and Cohn, A. G. (2012b). Egocen-
tric activity monitoring and recovery. In ACCV, pages
519–532.
Blank, M., Gorelick, L., Shechtman, E., Irani, M., and
Basri, R. (2005). Actions as space-time shapes. In
ICCV, pages 1395–1402.
Carneiro, G. and Lowe, D. (2006). Sparse flexible models
of local features. In ECCV, pages 29–43.
Crandall, D. J. and Huttenlocher, D. P. (2006). Weakly su-
pervised learning of part-based spatial models for vi-
sual object recognition. In ECCV (1), pages 16–29.
Deselaers, T. and Ferrari, V. (2010). Global and efficient
self-similarity for object classification and detection.
In CVPR, pages 1633–1640.
Doll
´
ar, P., Rabaud, V., Cottrell, G., and Belongie, S. (2005).
Behavior recognition via sparse spatio-temporal fea-
tures. In Visual Surveillance and Performance Eval-
uation of Tracking and Surveillance, 2005. 2nd Joint
IEEE International Workshop on, pages 65–72.
Fan, R.-E., Chang, K.-W., Hsieh, C.-J., Wang, X.-R., and
Lin, C.-J. (2008). LIBLINEAR: A library for large
linear classification. Journal of Machine Learning Re-
search, 9:1871–1874.
Fathi, A., Farhadi, A., and Rehg, J. M. (2011a). Under-
standing egocentric activities. In ICCV, pages 407–
414.
Fathi, A., Ren, X., and Rehg, J. M. (2011b). Learning to
recognize objects in egocentric activities. In CVPR,
pages 3281–3288.
Gilbert, A., Illingworth, J., and Bowden, R. (2009). Fast
realistic multi-action recognition using mined dense
spatio-temporal features. In ICCV, pages 925–931.
Gupta, A. and Davis, L. S. (2007). Objects in action: An ap-
proach for combining action understanding and object
perception. In CVPR, pages 1–8.
Kitani, K. M., Okabe, T., Sato, Y., and Sugimoto, A. (2011).
Fast unsupervised ego-action learning for first-person
sports videos. In CVPR, pages 3241–3248.
Kuehne, H., Jhuang, H., Garrote, E., Poggio, T., and Serre,
T. (2011). HMDB: A large video database for human
motion recognition. In ICCV, pages 2556–2563.
Laptev, I. and Lindeberg, T. (2003). Space-time interest
points. In ICCV, pages 432–439.
Laptev, I., Marszalek, M., Schmid, C., and Rozenfeld,
B. (2008). Learning realistic human actions from
movies. In CVPR, pages 1–8.
Liu, D., Hua, G., Viola, P. A., and Chen, T. (2008). Inte-
grated feature selection and higher-order spatial fea-
ture extraction for object categorization. In CVPR,
pages 1–8.
EgocentricActivityRecognitionusingHistogramsofOrientedPairwiseRelations
29