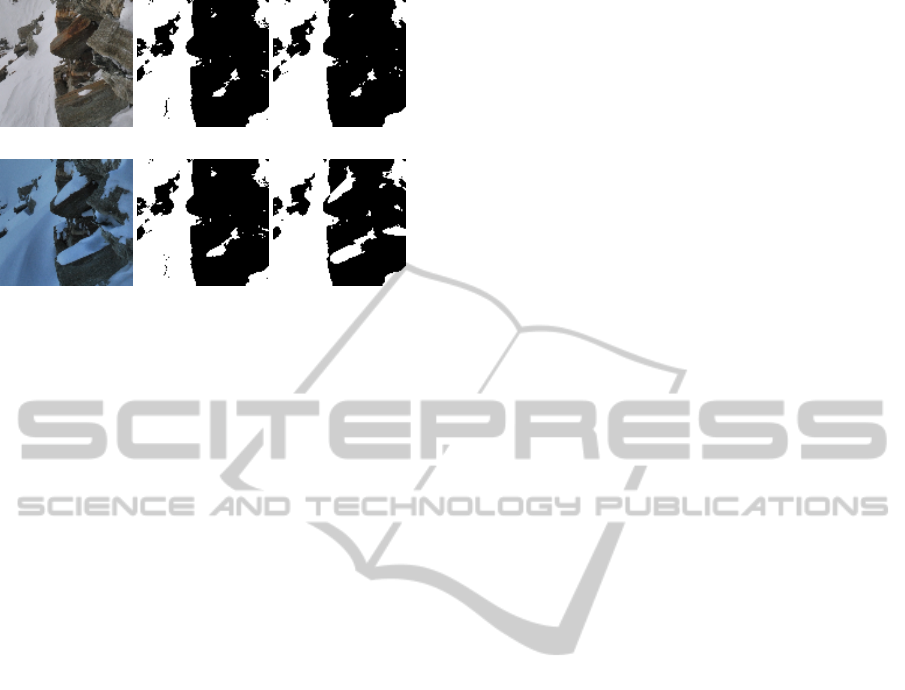
Original L
t
M
t
1,8
H
t
100
Original L
t+1
M
t+1
1,8
H
t+1
100
Figure 10: Crop of two consecutive snow maps, before and
after a major snow fall. (a) and (d) are the originals, (b) and
(e) the median filtered maps M
t
, and (c) and ( f ) the MRF
H
t+1
. The MRF almost completely reacts to the change in
snow coverage.
the database are shown.
The image at instant t (2. February 2010,
11.30am) is the last one taken just before the one at
t + 1 (3. February 2010, 2.26pm). A major snow fall
happened in-between, which explains the important
changes in snow coverage. Note how the median filter
approach is unable to adapt to the changes and under-
estimates the amount of snow coverage at time instant
t + 1, whereas the MRF approach is adapting almost
completely.
7 CONCLUSIONS AND FUTURE
WORK
We propose a technique for robust snow cover estima-
tion from time-lapse imagery. Since many of the im-
ages are uninformative, single image snow segmen-
tation using GMM of color is insufficient to get tem-
porally consistent results. We use Markov Random
Fields (MRF) and formulate the temporal consistency
problem as an energy minimization, where we use a
penalty term to penalize neighboring pixels (spatially
and temporally) with different labels. Due to the na-
ture of the image data, the weight of the penalty term
has to be quite large in order to provide temporally
consistent results. The higher the weight, the less re-
active the model is to changes in snow coverage. Us-
ing domain-knowledge, we propose a classifier to ex-
clude most of the uninformative images. Using only
the good images allows to relax the temporal con-
straints, making the model more reactive to changes.
The proposed model is both robust to outliers as well
as very reactive to changes in snow coverage.
Future work includes attempting the joint optimiza-
tion over space and time of Equation (10), and the
implementation of a snow deposition model, which
would be useful for long periods of uninformative
and/or missing images. This model could be used in-
stead of the zero-order hold we applied, resulting in
smoother transitions between two good images. An-
other interesting path to follow is to have different
models for different weather states, which could help
improve the initial snow cover estimations.
ACKNOWLEDGEMENTS
This work is in part supported by the National Com-
petence Center in Research on Mobile Information
and Communication Systems (NCCR-MICS), a cen-
ter supported by the Swiss National Science Founda-
tion under grant number 5005-67322.
REFERENCES
Blake, A., Rother, C., Brown, M., Perez, P., and Torr, P.
(2004). Interactive image segmentation using an adap-
tive GMMRF model. European Conference on Com-
puter Vision (ECCV), pages 428–441.
Boykov, Y., Veksler, O., and Zabih, R. (2001). Fast ap-
proximate energy minimization via graph cuts. IEEE
Transactions on Pattern Analysis and Machine Intel-
ligence, 23(11):1222–1239.
Breitenstein, M. D., Grabner, H., and Van Gool, L. (2009).
Hunting Nessie - Real-Time Abnormality Detection
from Webcams. IEEE International Conference on
Computer Vision (ICCV), pages 1243–1250.
Floros, G. and Leibe, B. (2012). Joint 2D-3D temporally
consistent semantic segmentation of street scenes.
IEEE Conference on Computer Vision and Pattern
Recognition (CVPR).
Hasler, A., Talzi, I., Beutel, J., Tschudin, C., and Gruber,
S. (2008). Wireless sensor networks in permafrost
research-concept, requirements, implementation and
challenges. Proceedings of the 9th International Con-
ference on Permafrost (NICOP).
Jacobs, N., Roman, N., and Pless, R. (2007). Consistent
temporal variations in many outdoor scenes. IEEE
Conference on Computer Vision and Pattern Recog-
nition (CVPR).
Keller, M., Beutel, J., and Thiele, L. (2009a). Demo
Abstract: MountainviewPrecision Image Sensing on
High-Alpine Locations. Adjunct Proceedings of the
6th European Workshop on Sensor Networks (EWSN),
pages 15–16.
Keller, M., Y
¨
ucel, M., and Beutel, J. (2009b). High-
Resolution Imaging for Environmental Monitoring
Applications. Proc. International Snow Science Work-
shop, pages 197–201.
VISAPP2014-InternationalConferenceonComputerVisionTheoryandApplications
282