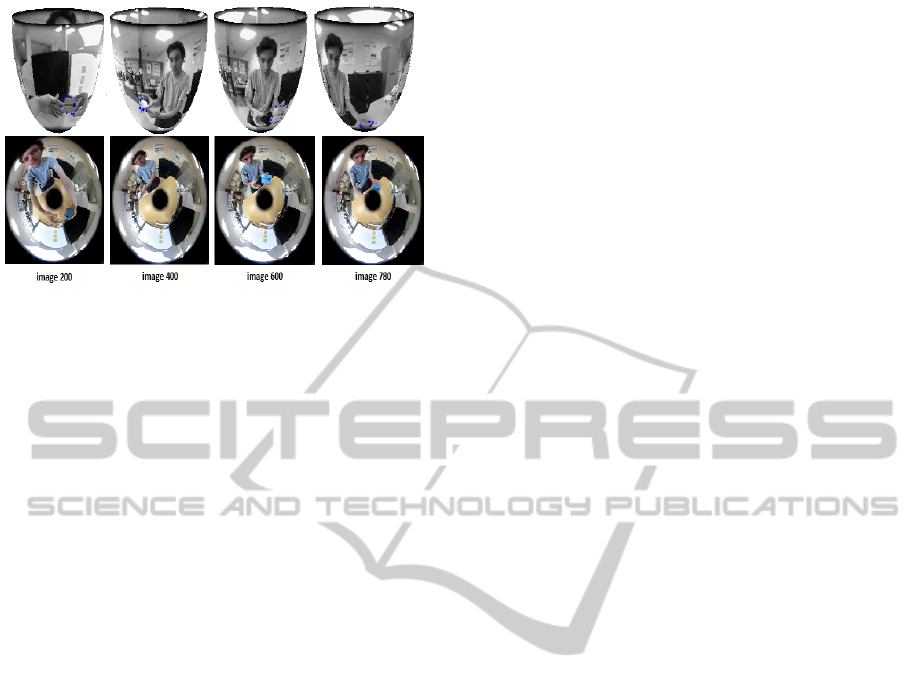
Figure 9: Tracking result -3-.
6 CONCLUSIONS
In our spherical tracking, a first object detection based
on background subtraction is applied to the starting
image. The minimization algorithm to the others im-
ages is applied. Using the adapted process and projec-
tion approach, the object contour is perfectly tracked
in the equivalent half sphere. The tracking time is
about 0.41 seconds and the detection consumes 0.34
seconds. We conclude from the obtained results, that
using of snake in tracking gives results promoting in
terms of edge detection and tracking such as the al-
gorithm convergence in minimization of the energy
functional. For the minimization algorithm, we want
to specify two stop criterion, corner number and im-
age energy threshold. The corner conditions are veri-
fied when the coefficient of rigidity β is equal to zero.
Using snake in tracking is the most used approachs in
video surveillance due to the extensibility and ability
of recognition for both object shape and orientation.
In addition, it’s adapted well to object size changes in
catadioptric image. However, this method is limited
mainly by the choosing of the energy parameters and
also occurred error by the minimization algorithm.
REFERENCES
Mikhael Kass, Andrew Witkin, Demetri terzopoulos
”Snakes: Active Contour Models”, International
Journal of Computer Vision, page :321−331(1988).
Lucas, B. D., and Kanade, T. ”An iterative image registra-
tion technique with an application to stereo vision”.
Proc. Int. Joint Conf. on Artificial Intelligence, Van-
couver, Canada, 1981, pp. 674−859.
Comaniciu, D., Ramesh, V., and Meer, P. ”Real-time track-
ing of non-rigid objects using mean shift”. Proc. IEEE
Conf. on Computer Vision and Pattern Recognition,
Hilton Head Kland, SC, USA, 2000, pp. 142−149.
Isard, M., and Blake, A. ”Condensation conditional density
propagation for visual tracking”, Int. J. Comput. Vis. ,
1998, 28, (1), pp.5−28.
Donna J. Wiliams, Mubarak Shah. ”A Fast Algorithm For
Active Contours and Curvature Estimation”,CVGIP
:image understanding, Vol.55, No.1, January, pp.14-
26,1992.
Geyer C., Daniilidis K. , ”Catadioptric Projective Geome-
try”, International Journal of Computer Vision, 2001.
David G. Lowe, ”Distinctive Image Features from Scale-
Invariant Keypoints”, International Journal of Com-
puter Vision, vol. 60, no 2, 2004, p. 91-110, 2010.
F. Rameau, D. Sidibe, C. Demonceaux, D. Fofi. ”Visual
Tracking with Omnidirectional Cameras : An Effi-
cient Approach”. IET Electronic Letters, Vol.47, Issue
21, pp.1183-1184, October 2011.
N. Ikoma,R. Yamaguchi, H. Kawano, and H. Maeda.
”Tracking of Multiple Moving Objects in Dynamic
Image of Omnidirectional Camera Using PHD Filter”.
Journal of Advanced Computational Intelligence and
Intelligent Informatics Vol.12 No.1, 2008.
David Hurych, Karel Zimmermann, Tomas Svoboda. ”Fast
learn able Object tracking and detection in high-
resolution omnidirectional images”, visapp2011.
K. Daniilidis, A. Makadia, T. Bulow, ”Image processing
in catadioptric planes: Spatiotemporal derivatives and
optical flow computation”, Omnidirectional Vision,
Third Workshop on, 3-10 2002.
Simon Baker and Shree K. Nayar, ”Single Viewpoint Cata-
dioptric Cameras,” Panoramic Vision: Sensors, The-
ory, Applications, Ryad Benosman and Sing Bing
Kang, ed., Springer-Verlag, 2001.
J.-P. Antoine and P. Vandergheynst. ”Wavelets on the 2-
sphere: A group-theoretical approach. Applied and
Computational Harmonic Analysis”, 7:262291, 1999.
Guillaume Caron, El Mustapha Mouaddib, Eric Marchand.
”3D model based tracking for omnidirectional vision:
A new spherical approach”,In International Journal
on Robotics and Autonomous Systems 60, 10561068,
2012.
C. Mei, E. Malis, S. Benhimane, P. Rives. ”Constrained
Multiple Planar Template Tracking for Central Cata-
dioptric Cameras.” In British Machine Vision Confer-
ence, Edinburgh, United Kingdom, September 2006.
VISAPP2014-InternationalConferenceonComputerVisionTheoryandApplications
440