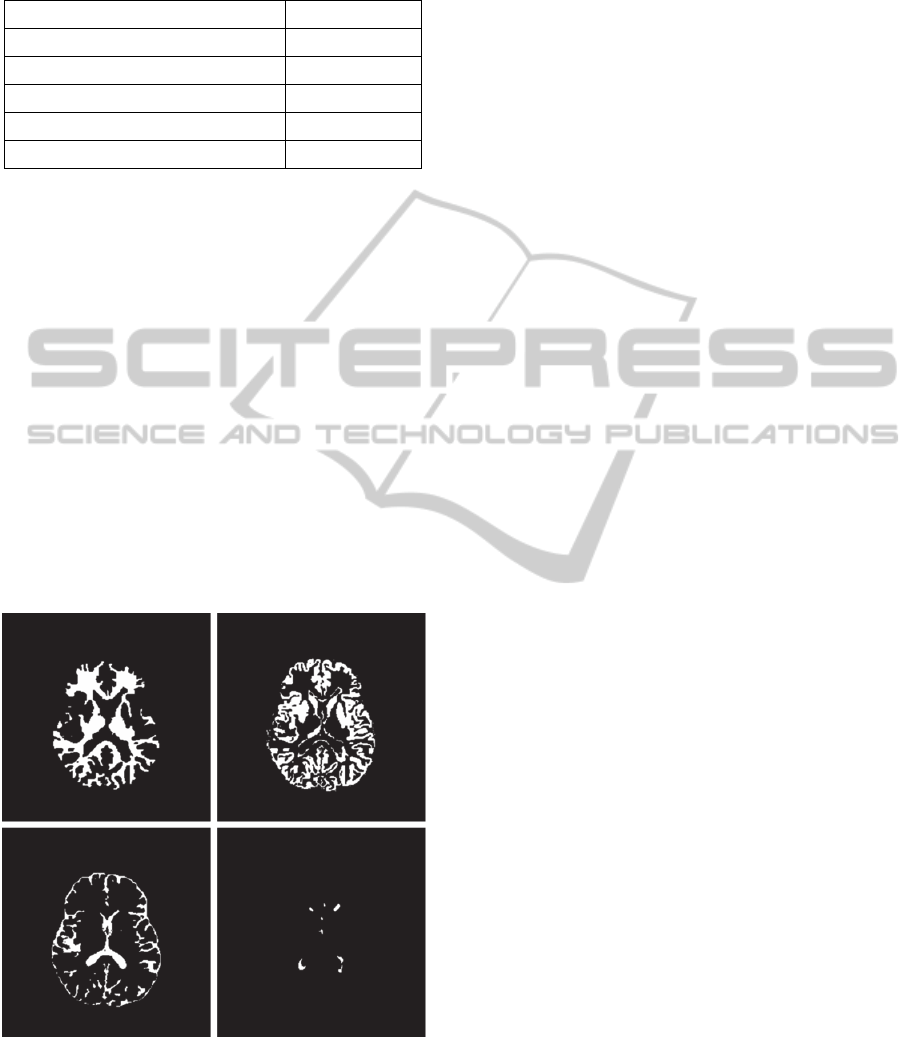
Table 1: Execution times (mean ± standard deviation) for
different modules in the online image analysis pipeline
measured in six datasets.
Processing module Time (sec)
Registration 43 ± 9
Brain extraction 3.2 ± 0.2
Nonuniformity correction 39 ± 13
Tissue and lesion segmentation 38 ± 2
Total time 123 ± 18
quantitative analysis. The quantitative results can
also help determine the optimum parameters for
subsequent scans. The proposed online analysis can
be applied for online longitudinal evaluation to
detect changes in MS lesion activity relative to a
previous scan using subtraction MRI, which has
shown a potential to predict the course of the disease
(Liguori et al., 2011).
Although the 2-min processing time is
considerably short compared to the acquisition time
of the same data (~8 min), shorter processing times
are still desirable, especially when the protocol
requires the segmentation results before executing
the next imaging sequence. Parallel processing using
graphical processing units (GPU) is becoming
increasingly popular for medical image analysis
(Pratx and Xing, 2011), and will be adopted in future
work for further speedup.
Figure 4: Segmentation results showing masks of white
matter (top left), grey matter (top right), CSF (bottom left),
and WM lesions (bottom right) corresponding to the slice
shown in Fig. 3.
5 CONCLUSIONS
We have developed and implemented an online
system for MRI image analysis and demonstrated its
application for brain tissue classification and WM
lesion segmentation in MS patients. The online
image analysis pipeline was integrated into a clinical
MRI system that allowed a seamless workflow
wherein the results of quantitative analysis were
easily incorporated into the patient database and the
scanner’s user interface. We expect the proposed
framework to have an impact on patient
management, bringing what has been largely an
isolated research activity to be part of the general
imaging practice.
ACKNOWLEDGMENTS
The authors thank Vipulkumar Patel for assistance in
acquiring the MRI data. This work was supported by
NIH/NINDS grant R01 NS078244.
REFERENCES
Caramanos, Z., Francis, S. J., Narayanan, S., Lapierre, Y.
and Arnold, D. L. (2012) 'Large, nonplateauing
relationship between clinical disability and cerebral
white matter lesion load in patients with multiple
sclerosis', Archives of neurology, vol. 69, no. 1, p. 89.
Collignon, A., Maes, F., Delaere, D., Vandermeulen, D.,
Suetens, P. and Marchal, G. (1995) 'Automated multi-
modality image registration based on information
theory', Information processing in medical imaging,
263-274.
Datta, S. and Narayana, P. A. (2011) 'Automated brain
extraction from T2-weighted magnetic resonance
images', Journal of Magnetic Resonance Imaging, vol.
33, no. 4, pp. 822-829.
Datta, S. and Narayana, P.A. (2013) 'A comprehensive
approach to the segmentation of multichannel three-
dimensional MR brain images in multiple sclerosis',
NeuroImage: Clinical, vol. 2, pp. 184-196.
Datta, S., Sajja, B. R., He, R., Wolinsky, J. S., Gupta, R.
K. and Narayana, P.A. (2006) 'Segmentation and
quantification of black holes in multiple sclerosis',
Neuroimage, vol. 29, no. 2, p. 467.
Fisniku, L., Brex, P., Altmann, D., Miszkiel, K., Benton,
C., Lanyon, R., Thompson, A. and Miller, D. (2008)
'Disability and T2 MRI lesions: a 20-year follow-up of
patients with relapse onset of multiple sclerosis',
Brain, vol. 131, no. 3, pp. 808-817.
Karimaghaloo, Z., Shah, M., Francis, S. J., Arnold, D. L.,
Collins, D. and Arbel, T. (2012) 'Automatic detection
of Gadolinium-enhancing multiple sclerosis lesions in
OnlineBrainTissueClassificationinMultipleSclerosisusingaScanner-integratedImageAnalysisPipeline
109