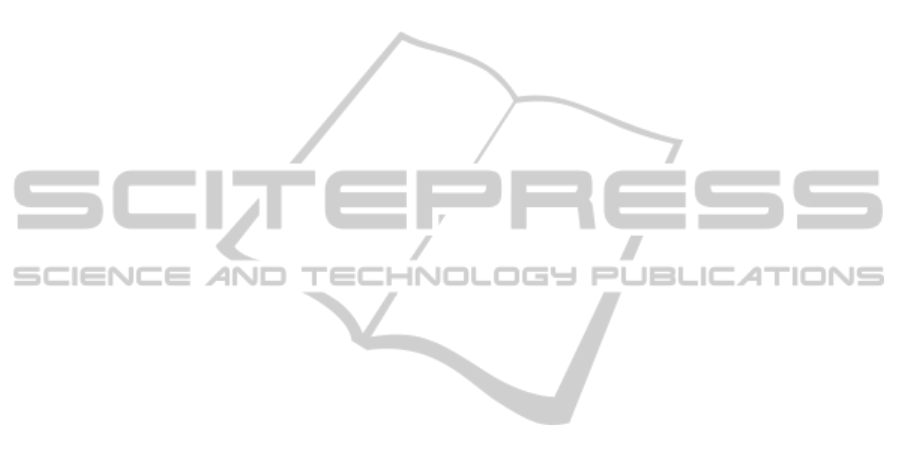
Future work will focus on the study of more emo-
tional states for a better understanding of the quality
of human movements and the intentions of the per-
former. In addition, more performances from differ-
ent actors will be captured for better evaluation of the
results; some captures will take place at dance schools
to reduce the potential influences of the laboratory en-
vironments. We are also planning to study how the
gender, age, weight and height affect the emotion ex-
pression and recognition and whether these factors
can be correlated with motion and emotional state.
Furthermore, we will study the performance of the
classifier in relation to the size of the window used for
motion clips’ segmentation, as well as the weight of
influence of each feature in the classification of move-
ments. Besides, the results of this paper will be re-
ferred to establish a similarity function that measures
the correlation between different actions. In contrast
to the existing techniques, we intend to compare ev-
ery movement based, not only on the position, posture
or the rotation of the limbs, but on the motion qualita-
tive and quantitative characteristics, such as the effort
and the purpose that has been executed. In addition,
the motion graphs (Zhao and Safonova, 2009) that in-
dicate possible future action paths will be enriched,
apart from whether a movement is well-matched to
another, with the qualitative and quantitative charac-
teristics of the action.
ACKNOWLEDGEMENTS
This project (DIDAKTOR/0311/73) is co-financed by
the European Regional Development Fund and the
Republic of Cyprus through the Research Promotion
Foundation. The authors would also like to thank Mrs
Anna Charalambous for her valuable help in explain-
ing LMA, as well as all the dancers who performed at
our department.
REFERENCES
Alaoui, S. F., Jacquemin, C., and Bevilacqua, F. (2013).
Chiseling bodies: an augmented dance performance.
In Proceedings of ACM SIGCHI Conference on Hu-
man Factors in Computing Systems, Paris, France.
ACM.
Arikan, O., Forsyth, D. A., and O’Brien, J. F. (2003).
Motion synthesis from annotations. ACM Trans. of
Graphics, 22(3):402–408.
Barbi
ˇ
c, J., Safonova, A., Pan, J.-Y., Faloutsos, C., Hodgins,
J. K., and Pollard, N. S. (2004). Segmenting motion
capture data into distinct behaviors. In Proceedings of
Graphics Interface, GI ’04, pages 185–194.
Chan, J. C. P., Leung, H., Tang, J. K. T., and Komura, T.
(2011). A virtual reality dance training system using
motion capture technology. IEEE Trans. on Learning
Technologies, 4(2):187–195.
Chao, M.-W., Lin, C.-H., Assa, J., and Lee, T.-Y. (2012).
Human motion retrieval from hand-drawn sketch.
IEEE Trans. on Visualization and Computer Graph-
ics, 18(5):729–740.
Chi, D., Costa, M., Zhao, L., and Badler, N. (2000). The
emote model for effort and shape. In Proceedings of
SIGGRAPH ’00, pages 173–182, NY, USA. ACM.
Cimen, G., Ilhan, H., Capin, T., and Gurcay, H. (2013).
Classification of human motion based on affective
state descriptors. Computer Animation and Virtual
Worlds, 24(3-4):355–363.
CMU (2003). Carnegie Mellon Univiversity: MoCap
Database. http://mocap.cs.cmu.edu/.
Deng, Z., Gu, Q., and Li, Q. (2009). Perceptually consistent
example-based human motion retrieval. In Proceed-
ings of I3D ’09, pages 191–198, NY, USA. ACM.
Fang, A. C. and Pollard, N. S. (2003). Efficient synthe-
sis of physically valid human motion. ACM Trans. of
Graphics, 22(3):417–426.
Gleicher, M. (1998). Retargetting motion to new characters.
In Proceedings of SIGGRAPH ’98, pages 33–42, NY,
USA. ACM.
Hartmann, B., Mancini, M., and Pelachaud, C. (2006). Im-
plementing expressive gesture synthesis for embod-
ied conversational agents. In Proceedings of GW’05,
pages 188–199. Springer-Verlag.
Hecker, C., Raabe, B., Enslow, R. W., DeWeese, J., May-
nard, J., and van Prooijen, K. (2008). Real-time
motion retargeting to highly varied user-created mor-
phologies. ACM Trans. of Graphcis, 27(3):1–27.
Ikemoto, L. and Forsyth, D. A. (2004). Enriching a motion
collection by transplanting limbs. In Proceedings of
SCA ’04, pages 99–108, Switzerland.
Kapadia, M., Chiang, I.-k., Thomas, T., Badler, N. I., and
Kider, Jr., J. T. (2013). Efficient motion retrieval in
large motion databases. In Proceedings of I3D ’13,
pages 19–28, NY, USA. ACM.
Keogh, E., Palpanas, T., Zordan, V. B., Gunopulos, D.,
and Cardle, M. (2004). Indexing large human-motion
databases. In Proceedings of VLDB, pages 780–791.
Kovar, L. and Gleicher, M. (2004). Automated extraction
and parameterization of motions in large data sets.
ACM Trans. of Graphics, 23(3):559–568.
Kovar, L., Gleicher, M., and Pighin, F. (2002). Motion
graphs. ACM Trans. of Graphics, 21(3):473–482.
Kr
¨
uger, B., Tautges, J., Weber, A., and Zinke, A. (2010).
Fast local and global similarity searches in large mo-
tion capture databases. In Proceedings of SCA ’10,
pages 1–10, Switzerland. Eurographics Association.
Kwon, T., Cho, Y.-S., Park, S. I., and Shin, S. Y.
(2008). Two-character motion analysis and synthesis.
IEEE Trans. on Visualization and Computer Graph-
ics, 14(3):707–720.
Lamb, W. (1965). Posture & gesture: an introduction to the
study of physical behaviour. G. Duckworth, London.
GRAPP2014-InternationalConferenceonComputerGraphicsTheoryandApplications
286