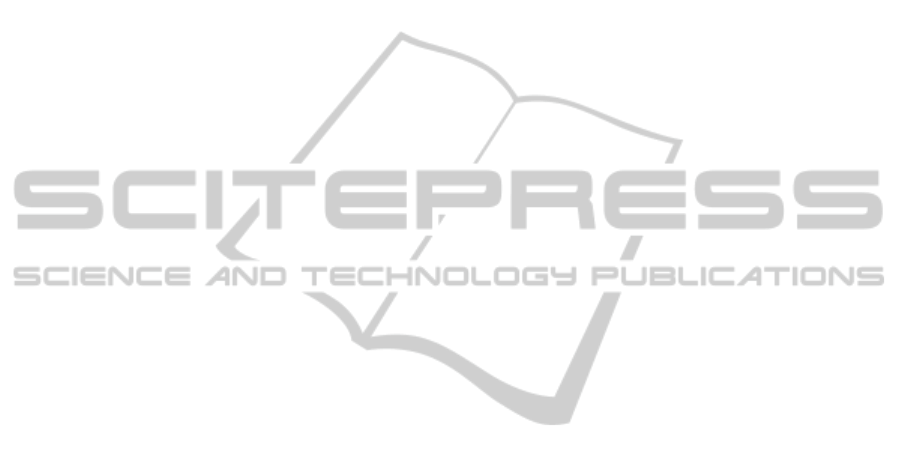
Bootstrap (c
b
) confidence measure detects if the
model is unstable, that is, when small perturbations
in the input data produce high variations in the out-
put. Thus, it detects points that do not satisfy model
assumptions like edges of object following different
motions and textureless regions.
Image Local Structure (c
k
) confidence measure
detects those pixels the image structure is not appro-
priate to solve optical flow, like textureless regions,
and straight lines. However, textured regions may
contain a lot of noise disturbing computations and,
also, failing of OF assumptions is not considered.
We conclude that c
s
is not the best suited for
bounding OF error. Besides, c
k
, c
b
, c
e
are able to
bound a different kinds of error and, in fact, they pro-
vide complementary bounds.
Concerning existing methods for evaluation
of confidence measures, EPP better reflect non-
decreasing profiles between CM and OF error and,
thus, it is better suited for detecting CM unable to
bound errors for a significant amount of cases. How-
ever, none of the evaluation methods provides enough
information about confidence measures failing cases
and thus, further research is required.
As a future work it would be interesting to view
examples for patches with more than two motion
areas and illumination changes. Also use a larger
database. And finally, check the confidence measure
performances on different optical flow methods.
ACKNOWLEDGEMENTS
Work supported by the Spanish projects TIN2009-
13618, TIN2012-33116 and TRA2011-29454-C03-
01 and first author by FPI-MICINN BES-2010-
031102 program.
REFERENCES
Baker, S., Scharstein, D., Lewis, J., and et al. (2011). A
database and evaluation methodology for optical flow.
IJCV, 92(1):1–31.
Barron, J. L., Fleet, D. J., and Beauchemin, S. S. (1994).
Performance of optical flow techniques. IJCV,
12(1):43–77.
Bruhn, A. and Weickert, J. (2006). A confidence mea-
sure for variational optic flow methods. In Geometric
Properties for Incomplete Data, pages 283–298.
Bruhn, A., Weickert, J., and Schn
¨
orr, C. (2005). Lu-
cas/Kanade meets Horn/Schunck: Combining local
and global opticflow methods. IJCV, 61(2):221–231.
Butler, D. J., Wulff, J., Stanley, G. B., and Black, M. J.
(2012). A naturalistic open source movie for optical
flow evaluation. In ECCV, pages 611–625.
Cheney, W. and Kincaid, D. (2008). Numerical Mathemat-
ics and Computing, Sixth edition. Bob Pirtle, USA.
Gehrig, S. and Scharwachter, T. (2011). A real-time multi-
cue framework for determining optical flow confi-
dence. In ICCV Workshops, pages 1978–1985. IEEE.
Horn, B. and Schunck, B. (1981). Determining optical flow.
AI, 17:185–203.
Kondermann, C., Mester, R., and Garbe, C. S. (2008). A
statistical confidence measure for optical flows. In
ECCV, pages 290–301.
Kybic, J. and Nieuwenhuis, C. (2011). Bootstrap optical
flow confidence and uncertainty measure. Computer
Vision and Image Understanding, pages 1449–1462.
Liu, C. (2009). Beyond pixels: exploring new representa-
tions and applications for motionanalysis. PhD thesis,
Cambridge, MA, USA.
Liu, C., Freeman, W., Adelson, E., and Weiss, Y. (2008).
Human-assisted motion annotation. In CVPR.
Lucas, B. and Kanade, T. (1981). An iterative image regis-
tration technique with an application to stereovision.
In DARPA IU Workshop, pages 121–130.
Mac Aodha, O., Humayun, A., Pollefeys, M., and Brostow,
G. J. (2013). Learning a confidence measure for op-
tical flow. IEEE Trans. Pattern Anal. Mach. Intell.,
35(5):1107–1120.
M
´
arquez-Valle, P., Gil, D., and Hern
`
andez-Sabat
´
e, A.
(2012). A complete confidence framework for opti-
cal flow. In ECCV Workshops, volume 7584 of LNCS,
pages 124–133. Springer.
McCane, B., Novins, K., Crannitch, D., and Galvin, B.
(2001). On Benchmarking Optical Flow. Computer
Vision and Image Understanding, 84(1).
Senst, T., Eiselein, V., and Sikora, T. (2012). Robust local
optical flow for feature tracking. IEEE Trans. Circuits
Syst. Video Techn., 22(9):1377–1387.
Shi, J. and Tomasi, C. (1994). Good features to track. In
CVPR, pages 593–600.
Singh, A. (1990). An estimation-theoretic framework for
discontinuous flow fields. In ICCV, pages 168–177.
Sundaram, N., Brox, T., and Keutzer, K. (2010). Dense
point trajectories by gpu-accelerated large displace-
ment optical flow. In ECCV, pages 438–451.
LocalAnalysisofConfidenceMeasuresforOpticalFlowQualityEvaluation
457