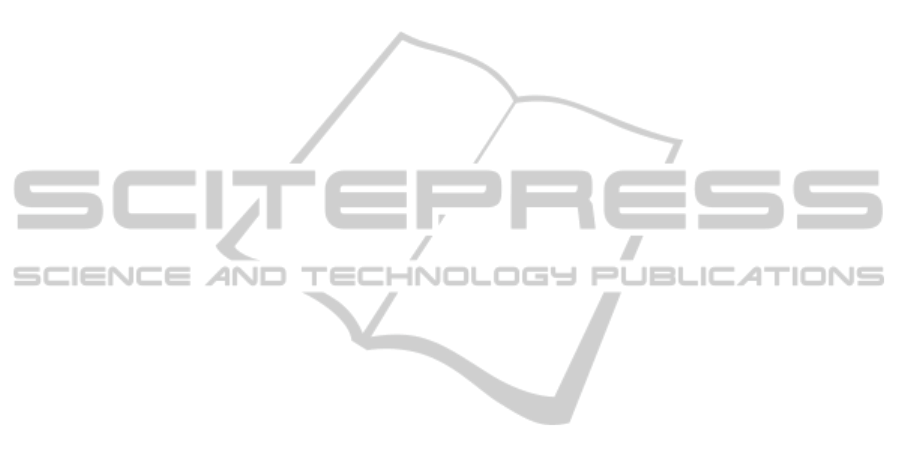
6 CONCLUSIONS
This paper describes how to learn the ocean surface
dynamics from an empirical model, EM, that sum-
marises the shallow water equations by an advec-
tion term and an additional term a. This last repre-
sents physical processes such as the Coriolis force,
the gravity force and the viscosity. A data assimila-
tion algorithm is defined for EM that estimates the
velocity field at the first acquisition date and the ad-
ditional term a(t) at each date of the studied inter-
val. a(t) is of major importance for correctly assess-
ing the hidden physical processes and accurately es-
timating motion on the whole image sequence. The
algorithm does not involve any parameter other than
those of the data assimilation framework (error co-
variance matrices). The method has been illustrated
on several SST sequences of Black Sea and has been
quantified by tracking feature points. Moreover, it has
been compared with state-of-the-art optical flow algo-
rithms. The conclusion is that a model of dynamics,
even if simple, improves motion estimation and al-
lows tracking of structures.
This approach may be seen as a first step to model
the physical processes occurring at the ocean surface
from image data. The short-term perspectives will be
to compare the additional term a(t) with forces in-
volved in the shallow water model, in order to further
validate the ability of the empirical model to assess
geophysical processes.
ACKNOWLEDGEMENTS
Data have been provided by E. Plotnikov and G. Ko-
rotaev from the Marine Hydrophysical Institute of
Sevastopol, Ukraine.
REFERENCES
Byrd, R. H., Lu, P., and Nocedal, J. (1995). A limited
memory algorithm for bound constrained optimiza-
tion. Journal on Scientific and Statistical Computing,
16(5):1190–1208.
Dee, D. (2005). Bias and data assimilation. Quaterly Jour-
nal of the Royal Meteorological Society, 131:3323–
3343.
Hasco
¨
et, L. and Pascual, V. (2004). Tapenade 2.1 user’s
guide. Technical Report 0300, INRIA.
Hasco
¨
et, L. and Pascual, V. (2013). The Tapenade Au-
tomatic Differentiation tool: Principles, Model, and
Specification. ACM Transactions On Mathematical
Software, 39(3).
Hundsdorfer, W. and Spee, E. (1995). An efficient hori-
zontal advection scheme for the modeling of global
transport of constituents. Monthly Weather Review,
123(12):3,554–3,564.
Huot, E., Herlin, I., Mercier, N., and Plotnikov, E. (2010).
Estimating apparent motion on satellite acquisitions
with a physical dynamic model. In International Con-
ference on Image Processing (ICPR), pages 41–44.
Korotaev, G., Huot, E., Le Dimet, F.-X., Herlin, I.,
Stanichny, S., Solovyev, D., and Wu, L. (2008). Re-
trieving Ocean Surface Current by 4-D Variational As-
similation of Sea Surface Temperature Images. Re-
mote Sensing of Environment, 112:1464–1475.
Le Dimet, F. and Talagrand, O. (1986). Variational algo-
rithms for analysis and assimilation of meteorological
observations: theoretical aspects., pages 97–110. Tel-
lus.
LeVeque, R. (1992). Numerical Methods for Conserva-
tive Laws. Lectures in Mathematics. ETH Z
¨
urich,
Birkha
¨
user Verlag, 2nd edition.
Lions, J.-L. (1971). Optimal Control of Systems Governed
by Partial Differential Equations. Springer-Verlag.
Oguz, T., La Violette, P., and Unluata, U. (1992). The
upper layer circulation of the black sea: Its vari-
ability as inferred from hydrographic and satel-
lite observations. Journal of geophysical research,
78(C8):12,569–12,584.
Papadakis, N., Corpetti, T., and M
´
emin, E. (2007). Dynam-
ically consistent optical flow estimation. In Proceed-
ings of International Conference on Computer Vision
(ICCV), Rio de Janeiro, Brazil.
Sasaki, Y. (1970). Some basic formalisms in numeri-
cal varational analysis. Monthly Weather Review,
98(12):875–883.
Sun, D., Roth, S., and Black, M. (2010). Secrets of optical
flow estimation and their principles. In Proceedings
of European Conference on Computer Vision (ECCV),
pages 2432–2439.
Suter, D. (1994). Motion estimation and vector splines. In
Proceedings of Conference on Computer Vision and
Pattern Recognition (CVPR), pages 939–942.
Titaud, O., Vidard, A., Souopgui, I., and Le Dimet, F.-X.
(2010). Assimilation of image sequences in numerical
models. Tellus A, 62:30–47.
Tr
´
emolet, Y. (2006). Accounting for an imperfect model in
4D-Var. Quaterly Journal of the Royal Meteorological
Society, 132(621):2483–2504.
Vallis, G. K. (2006). Atmospheric and oceanic fluid dynam-
ics. Cambridge University Press. 745 pp.
Valur H
´
olm, E. (2008). Lectures notes on assimilation
algorithms. Technical report, European Centre for
Medium-Range Weather Forecasts Reading, U.K.
Wolke, R. and Knoth, O. (2000). Implicit-explicit Runge-
Kutta methods applied to atmospheric chemistry-
transport modelling. Environmental Modelling and
Software, 15:711–719.
Image-basedModellingofOceanSurfaceCirculationfromSatelliteAcquisitions
295