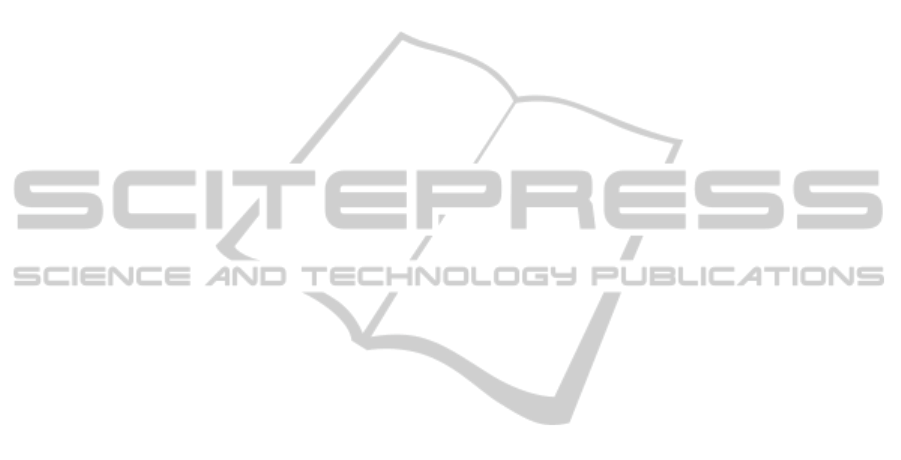
Branca, A., Simonian, P., Ferrante, M., Novas, E., Martin
Negri R., 2003. Electronic based discrimination of a
perfumery compound in a fragrance. In Sensors and
Actuators B, 92, 222-227.
Cho, J. H., Kim, Y. W., Na, K. J., Jeon G. J., 2008.
Wireless electronic nose system for real-time
quantitative analysis of gas mixtures using micro-gas
sensor array and neuro-fuzzy network. In Sensors and
Actuators B, 134, 104-111.
Delpha, C., Siadat, M., Lumbreras, M., 2001. An
electronic nose using time reduced modelling
parameters for a reliable discrimination of Forane
134a. In Sensors and Actuators B, 77, 517-524.
Distante, C., Leo, M., Siciliano, P., Persaud, K.C., 2002.
On the study of feature extraction methods for an
electronic nose. In Sensors and Actuators B, 87, 274-
288.
Falasconi, M., Pardo,M., Sberveglieri, G., Ricco, I.,
Bresciani, A., 2005. The novel EOS
835
electronic nose
and data analysis for evaluating coffee ripening. In
Sensors and Actuators B, 110, 73-80.
Gualdron O., Llobet E., Brezmes J., Vilanova X., Correig,
X., 2004. Fast variable selection for gas sensing
applications. In Sensors, 2004. Proceeding of IEEE, 2,
892-895.
Gutierrez-Osuna R., 2002. Pattern analysis for machine
olfaction: A review. In IEEE Sensor Journal, 2, 189-
202.
Ionescu, R., Vancu, A., Tomescu, A., 2000. Time-
dependent humidity calibration for drift corrections in
electronic noses equipped with SnO2 gas sensors. In
Sensors and Actuators B, 69, 283-286.
Jolliffe, I.T., 2002. Principal Component Analysis, Second
edition. New York: Springer.
Llobet, E., Ionescu, R., Al-Khalifa, S., Brezmes, J.,
Vilanova, X., Correig, X., Barsan, N., Gardner, J.W.,
2002. Multicomponent gas mixture analysis using a
single tin oxide sensor and dynamic pattern
recognition. In Sensors Journal, IEEE, 1 (3), 207-213.
Martin Negri R. and Reich S., 2001. Identification of
pollutant gases and its concentrations with a
multisensory array. In Sensors and Actuators B, 75,
172-178.
Martinelli, E., Falconia, C., D’Amico A., Di Natale, C.,
2003. Feature Extraction of chemical sensors in phase
space. In Sensors and Actuators B, 95, 132–139.
Pardo, M. and Sberveglieri. G., 2007. Comparing the
performance of different features in sensor arrays. In
Sensors and Actuators B, 123, 437–443.
Paulsson, N., Larsson, E., Winquist, F., 2000. Extraction
and selection of parameters for evaluation of breath
alcohol measurement with an electronic nose. In
Sensors and Actuators B, 84, 187-197.
Roussel, S., Forberg, G., Grenier P., Bellon-Maurel, V.,
1999. Optimisation of electronic nose measurements.
partII : Influence of experimental parameters. In
Journal of Food Engineering, 39, 9-15.
Sambemana, H., Siadat, M., Lumbreras, M., 2010. Gas
sensing evaluation for the quantification of natural oil
diffusion. In Chemical Engineering Transaction, 23,
177-183.
Savitzky, A. and Golay, M.J.E., 1967. Smoothing and
Differentiation of Data by Simplified Least
SquaresProcedures. In Anal. Chem., 36 (8), 1627–
1639.
Sysoev, V. V., Goschnick, J., Schneider, T., Strelcov, E.,
Kolmakov, A., 2007. A gradient microarray electronic
based on percolating SnO2 nanowire sensing
elements. In Nano letters, 7 (10), 3182-3188.
Szczurek, A. and Maciejewska, A., 2012. Gas Sensor
Array with Broad Applicability, Sensor Array, Prof.
Wuqiang Yang (Ed.), ISBN: 978-953-51-0613-5,
InTech. Available from: http://www.intechopen.com/
books/sensor-array/gas-sensor-array-with-broad-
applicability.
Zhang, S., Xie, C., Zeng, D., Zhang, Q., Li, H., Bi, Z.,
2007. A feature extraction method and a sampling
system for fast recognition of flammable liquids with a
portable E-nose. In Sensors and Actuators B, 124,
437-443.
Zhang, H. and Wang, J., 2007. Detection of age and insect
damage incurred by wheat, with an electronic nose. In
Journal of Stored Products Research, 43, 489-495.
SensorsandFeaturesSelectionforRobustGasConcentrationEvaluation
243