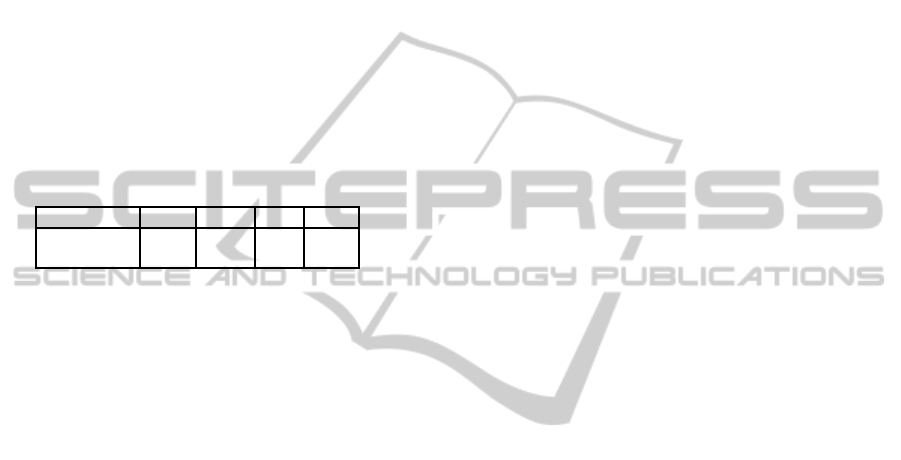
Influence of the shape and texture on the accuracy.
We now verify the influence of texture or shape on
the pose and shape estimation quality. To this end, we
used two new sets of synthetic data:
• base B
shape
: ten sequences, changing only the
shape from one sequence to another one, all other
parameters remaining fixed;
• base B
tex
: ten sequences, changing only the tex-
ture from one sequence to another one.
We evaluated the pose and shape estimation using the
Levenberg-Marquardt algorithm. The accuracy vari-
ation for each of these bases is given in Table 2. We
report only the 2D errors (Err
2D
) using the 3-cameras
configuration 3A.
Table 2: Error variations depending on shape or texture
variations only. The Levenberg-Marquardt optimization has
been used on configuration 3A.
Variation Mean Sigma Min Max
Shape (B
shape
) 7.65 1.8 5.72 11.87
Texture (B
tex
) 7.57 0.45 7.03 8.46
The results are significantly more stable with the
base B
tex
than with the base B
shape
. This can be ex-
plained by the fact that texture variations slightly al-
ter the detector quality at fixed pose and shape. For
instance, the appearance of an eye corner does not
change considerably for different facial textures. The
detected points are therefore almost the same for all
sequences of B
tex
, leading to very similar estimations.
For the base B
shape
, the texture and the poses are
fixed for all sequences, so we can assume that the
quality of the detections is equivalent for all of them.
Nevertheless, the errors obtained for this base are
more varied than for B
tex
, which is due to the shape
variability in the sequences. Indeed, some real shapes
cannot be generated because of the model constraints.
Some faces will therefore be easy to represent and
lead to low errors, but for others, it will not be possi-
ble to fit correctly the model to the data. This explains
why it is important to use real head scans when gen-
erating the synthetic sequences, in order to reproduce
this problem when evaluating the pose and estimation
algorithms.
6 CONCLUSIONS AND FUTURE
WORK
We have presented a complete workflow to evalu-
ate configurations of face recognition gates in terms
of 3D fitting quality. The methodology we propose
is based on synthetic data, which can be generated
with any number and configuration of cameras, light-
ing condition and resolution, while maintaining other
conditions fixed (identities, face poses). This allows
us to test an unlimited number of alternatives, with-
out bias introduced by people behavior and trajectory
variations, or constraints related to real campaign ac-
quisitions and material conception. The evaluation is
based on the accuracy measure of the 3D head fitting,
which is easily computable as we benefit from the
groundtruth used to generate the sequences. The gen-
eral trend shows that increasing the number of cam-
eras improves the accuracy of the estimation. More-
over, for a fixed number of cameras, their position
also impacts the accuracy: diversifying the points of
view increases the estimation quality (two crossed
cameras are better than two vertical cameras...). This
factor can be optimized with simulations, thus limit-
ing the number of real systems to build when making
the real data evaluation (for instance, evaluation of
the configuration 3C is not available with the initial
4-cameras system). In the future, such studies could
be extended to other factors, such as lighting and ex-
pression.
We limited our evaluation to geometrical results
on synthetic data. Another extension to this work
would be to develop the following aspects. First, it
would be interesting to compute geometrical mea-
sures on real data. The difficulty of this point is to
get the real position of each face vertex during a se-
quence. Additional depth sensors should be used to
this aim, or, at least, the ground truth of the face
should be known (using a 3D scanner for instance).
Besides, the relation between biometric performances
and errors on the estimation (3D pose and shape)
should be deepened, with respect to different face
comparison algorithms.
REFERENCES
Amberg, B., Blake, A., Fitzgibbon, A., Romdhani, S., and
Vetter, T. (2007). Reconstructing High Quality Face-
Surfaces using Model-Based Stereo. In International
Conference on Computer Vision, pages 1–8.
Blanz, V., Grother, P., Phillips, P., and Vetter, T. (2005).
Face Recognition Based on Frontal Views Generated
from Non-Frontal Images. In Conference on Com-
puter Vision and Pattern Recognition, pages 454–461.
Blanz, V. and Vetter, T. (1999). A Morphable Model for the
Synthesis of 3D Faces. In SIGGRAPH, pages 187–
194.
Herold, C., Despiegel, V., Gentric, S., Dubuisson, S., and
Bloch, I. (2012). Head Shape Estimation using a Par-
ticle Filter including Unknown Static Parameters. In
International Conference on Computer Vision Theory
and Applications, pages 284–293.
VISAPP2014-InternationalConferenceonComputerVisionTheoryandApplications
304