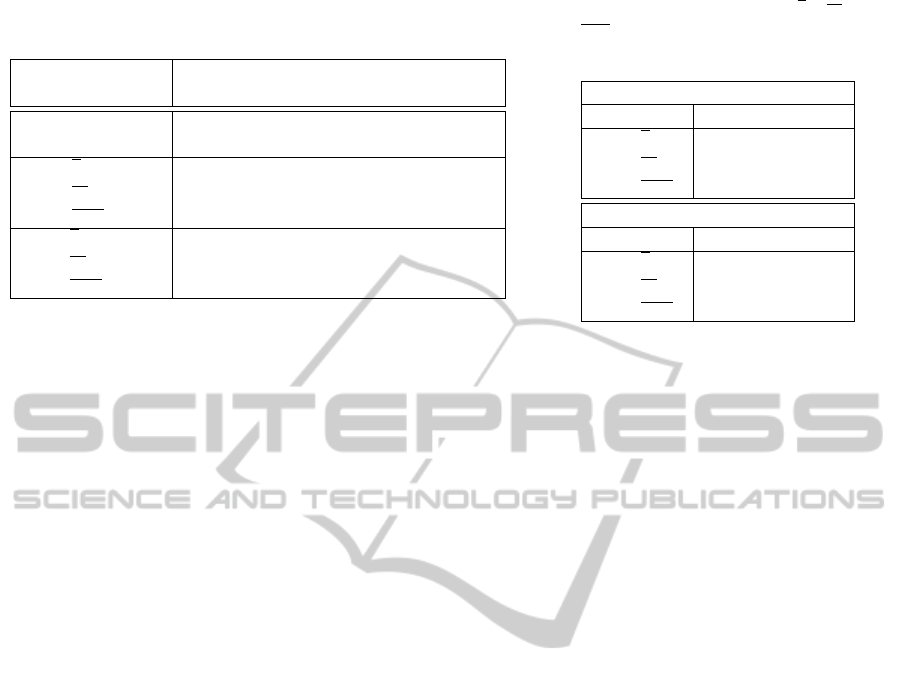
Table 2: mAP observed for various search strategies and re-ranking
techniques; Holidays, Oxford5k and Paris6k sets. k
0
= 1.
Method
Oxford5k Paris6k Holidays
BOF BOF BOF VLAD
baseline 0.598 0.691 0.549 0.571
(Qin et al., 2011) 0.814 0.803 - -
N
k
& j
k
0.701 0.752 0.582 0.606
N
k
& sc
k
0.700 0.748 0.581 0.602
N
k
& sgm
k
0.724 0.783 0.589 0.607
R
k
& j
k
0.737 0.768 0.685 0.655
R
k
& sc
k
0.734 0.765 0.684 0.654
R
k
& sgm
k
0.746 0.804 0.687 0.660
Table 3: mAP for R
k
with j
k
, sc
k
,
sgm
k
. Varying initial neighborhood
size k
0
. Oxford5k and Paris6k sets.
Oxford5k
Method k
0
= 1 k
0
= 20
R
k
& j
k
0.737 0.779
R
k
& sc
k
0.734 0.777
R
k
& sgm
k
0.746 0.761
Paris6k
Method k
0
= 1 k
0
= 80
R
k
& j
k
0.768 0.820
R
k
& sc
k
0.765 0.820
R
k
& sgm
k
0.804 0.812
5 CONCLUSIONS
This paper presented three measures of the similar-
ity between neighborhoods of images, suitable for use
in shared-neighbor similarity reranking of images in
a query result. Extensions integrating the values of
these measures across a range of neighborhood sizes
were also presented. Experimental evidence shows
that the extended measures improve significantly the
mean average precision scores observed over state-of-
the-art standard image benchmark datasets. This pa-
per also presents a reciprocal rank criterion allowing
the construction of shortlists containing highly rele-
vant images. Both techniques, used in isolation or in
a combined manner, outperform standard techniques.
Overall, compared to the work presented in (Qin
et al., 2011), our approach provides a quite simple
and uniform framework for integrating the structural
information that can be obtained from the neighbor-
hood of images into the overall assessment of simi-
larity to the query point. Furthermore, our reranking
procedure remains free of complex parameter tuning
(since k
0
can be set to a fixed value by default), and
does not involve any optimization process, keeping
its complexity low. The method does require, how-
ever, the computation and storage of ranked neighbor
lists. The memory overhead therefore grows linearly
with the database size, in the same was as for the main
competing method due to Qin et al. (Qin et al., 2011).
REFERENCES
Fischler, M. A. and Bolles, R. C. (1981). Random sample
consensus: a paradigm for model fitting with appli-
cations to image analysis and automated cartography.
Communications of the ACM, 24(6).
Hamzaoui, A., Letessier, P., Joly, A., Buisson, O., and Bou-
jemaa, N. (2013). Object-based visual query sugges-
tion. Multimedia Tools and Applications.
Houle, M. E. (2008). The relevant-set correlation model for
data clustering. Statistical Analysis and Data Mining,
1(3).
Houle, M. E., Kriegel, H. P., Kr
¨
oger, P., Schubert, E., and
Zimek, A. (2010). Can shared-neighbor distances de-
feat the curse of dimensionality? In Scientific and
Statistical Database Management.
J
´
egou, H., Douze, M., and Schmid, C. (2008). Hamming
embedding and weak geometric consistency for large
scale image search. In ECCV.
J
´
egou, H., Douze, M., Schmid, C., and P
´
erez, P. (2010).
Aggregating local descriptors into a compact image
representation. In CVPR.
J
´
egou, H., Harzallah, H., and Schmid, C. (2007). A con-
textual dissimilarity measure for accurate and efficient
image search. In CVPR.
Lowe, D. G. (2004). Distinctive image features from scale-
invariant keypoints. International journal of computer
vision, 60(2).
Nister, D. and Stewenius, H. (2006). Scalable recognition
with a vocabulary tree. In CVPR.
Perronnin, F., Liu, Y., S
´
anchez, J., and Poirier, H. (2010).
Large-scale image retrieval with compressed fisher
vectors. In CVPR.
Philbin, J., Chum, O., Isard, M., Sivic, J., and Zisserman, A.
(2007). Object retrieval with large vocabularies and
fast spatial matching. In CVPR.
Philbin, J., Chum, O., Isard, M., Sivic, J., and Zisserman,
A. (2008). Lost in quantization: Improving particu-
lar object retrieval in large scale image databases. In
CVPR.
Qin, D., Gammeter, S., Bossard, L., Quack, T., and Gool,
L. V. (2011). Hello neighbor: accurate object retrieval
with k-reciprocal nearest neighbors. In CVPR.
Sivic, J. and Zisserman, A. (2003). Video google: A text
retrieval approach to object matching in videos. In
ICCV.
VISAPP2014-InternationalConferenceonComputerVisionTheoryandApplications
328