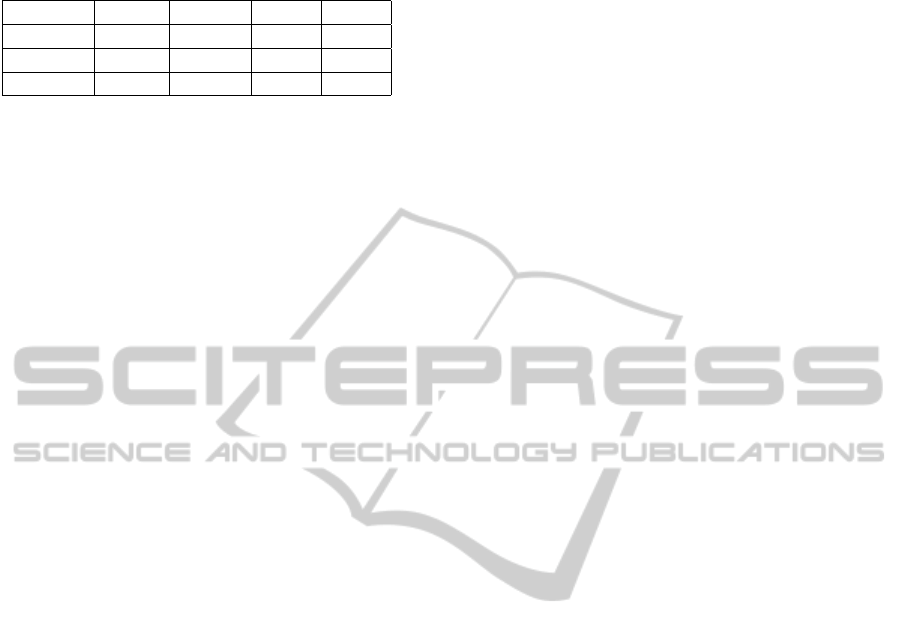
Table 5: Experimental results for 2-class categorization of
the second dataset (500 images).
F. sets Perf. CCE
−1
CCE
0
CCE
1
F
1
55.3% 110 275 115
F
2
, F
3
58.6% 99 294 107
F
3
...F
6
63.0% 98 315 87
techniques in this dataset, making the features less ef-
fective. Moreover, it contains almost no blurry images
and performing 2-class categorization using only the
blur value computed on the foreground region leads to
an average performance of only 52.3% (71% for the
previous dataset). Pictures have a lower score vari-
ance (0.75 on a 1 to 10 scale) which makes the cate-
gorization difficult.
5 CONCLUSIONS
In this work we proposed a method based on im-
age segmentation to explore aesthetics in portraits. A
few features were extracted, and image segmentation
techniques improved evaluation performance in both
categorization and score ranking tasks.
In the experiments, only a couple of hundred im-
ages have been used and it is not sufficient to cre-
ate accurate models, especially for aesthetic scoring.
Gathering more images from different sharing portals
and other datasets may help a lot.
The features described and computed are simple
descriptors. They can be combined with generic im-
age descriptors, other data related to portraits, etc. Fa-
cial features like hair color, background composition
and textures, make-up and facial expressions, as well
as presence of hats and glasses can be used to provide
more accurate scoring.
Implementing new relevant features will be part
of future work. Comparison between several learning
techniques will be performed and additional regions
explored (e.g. eyes and mouth locations).
This will be a first step in evaluating facial por-
traits with respect to other criteria like attractiveness,
competence, aggressiveness.
REFERENCES
Beucher, S. and Meyer, F. (1993). The morphological ap-
proach to segmentation: the watershed transforma-
tion. Mathematical Morphology in Image Processing,
pages 433–481.
Crete, F. and Dolmiere, T. (2007). The blur effect: percep-
tion and estimation with a new no-reference percep-
tual blur metric. Proc. of the SPIE, 6492.
Cronbach, L. (1951). Coefficient alpha and the internal
structure of tests. Psychometrika, 16(3).
Datta, R., Joshi, D., Li, J., and Wang, J. Z. (2006). Studying
Aesthetics in Photographic Images Using a Computa-
tional Approach. ECCV, pages 288–301.
Datta, R., Li, J., and Wang, J. Z. (2007). Learning the
consensus on visual quality for next-generation image
management. Proc. of the 15th international confer-
ence on Multimedia, pages 533–536.
Huang, G. and Mattar, M. (2008). Labeled faces in the wild:
A database for studying face recognition in uncon-
strained environments. Workshop on Faces in ’Real-
Life’ Images: Detection, Alignment, and Recognition,
pages 1–11.
Jones, M. and Rehg, J. (1999). Statistical color models with
application to skin detection. CVPR, 1:274–280.
Ke, Y., Tang, X., and Jing, F. (2006). The design of high-
level features for photo quality assessment. CVPR,
1:419–426.
Khan, S. and Vogel, D. (2012). Evaluating visual aesthetics
in photographic portraiture. Proc.of the Eighth Annual
Symposium on Computational Aesthetics in Graphics,
Visualization, and Imaging (CAe ’12), pages 1–8.
Li, C. and Gallagher, A. (2010). Aesthetic quality assess-
ment of consumer photos with faces. ICIP, pages
3221 – 3224.
Luo, Y. and Tang, X. (2008). Photo and video quality eval-
uation: Focusing on the subject. ECCV, pages 386–
399.
Murray, N. (2012). AVA: A large-scale database for aes-
thetic visual analysis. CVPR, 0:2408–2415.
Viola, P. and Jones, M. (2001). Rapid object detection using
a boosted cascade of simple features. CVPR, 1:511–
518.
Willis, J. and Todorov, A. (2006). Making Up Your Mind
After a 100-Ms Exposure to a Face. Psychological
Science, 17(7):592–598.
VISAPP2014-InternationalConferenceonComputerVisionTheoryandApplications
336