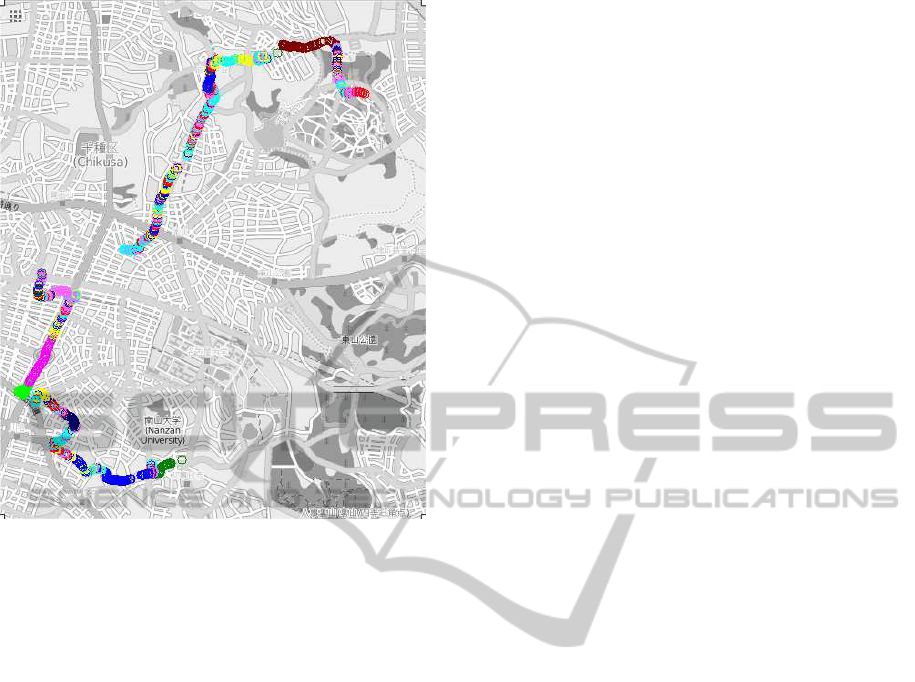
Figure 12: The result of driving scene classification drawn
on the map. Each circle with a different color plotted on the
road corresponds to a scene class.
bined with any conventional learning based pedes-
trian detection methods.
For future work, we will extend the method so
that it can be adapted to other factors of the driv-
ing environment such as time, weather conditions, or
season changes. In addition, we will improve the
scene classification by replacing the current BoVW
and k-means scheme, and the training image collec-
tion methods.
ACKNOWLEDGEMENTS
Parts of this research were supported by a Grant-in-
Aid for Young Scientists from MEXT, a Grant-In-Aid
for Scientific Research from MEXT, and a CREST
project from JST.
REFERENCES
Bay, H., Tuytelaars, T., and Gool, L. V. (2008). Surf:
Speeded up robust features. Computer Vision and Im-
age Understanding (CVIU), 110(3):346–359.
Broggi, A., Cerri, P., Ghidoni, S., Grisleri, P., and Jung,
H. G. (2009). A new approach to urban pedestrian
detection for automatic braking. IEEE Transactions
on Intelligent Transportation Systems, 10(4):594–605.
Csurka, G., Dance, C. R., Fan, L., Willamowski, J., and
Bray, C. (2004). Visual categorization with bags of
keypoints. In Proceedings of Workshop on Statistical
Learning in Computer Vision in the The 8th European
Conference on Computer Vision (ECCV), pages 1–22.
Dalal, N. and Triggs, B. (2005). Histograms of oriented gra-
dients for human detection. In Proceedings of 2005
IEEE Computer Society Conference on Computer Vi-
sion and Pattern Recognition, volume 1, pages 886–
893.
Fan, R.-E., Chang, K.-W., Hsieh, C.-J., Wang, X.-R., and
Lin, C.-J. (2008). LIBLINEAR: A library for large
linear classification. Journal of Machine Learning Re-
search, 9:1871–1874.
Mitsumori, R., Uchiyama, H., Saito, H., Servi`eres, M., and
Moreau, G. (2009). Change detection based on SURF
and color edge matching. In Proceedings of Workshop
on Vision and Control for Access Space (VCAS) in the
9th Asian Conference on Computer Vision (ACCV).
12p.
Nair, V. and Clark, J. J. (2004). An unsupervised, on-
line learning framework for moving object detection.
In Proceedings of 2004 IEEE Computer Society Con-
ference on Computer Vision and Pattern Recognition,
volume 1, pages 317–324.
Pang, J., Huang, Q., Yan, S., Jiang, S., and Qin, L. (2011).
Transferring boosted detectors towards viewpoint and
scene adaptiveness. IEEE Transactions on Image Pro-
cessing, 20(1):1388–1400.
Sand, P. and Teller, S. (2004). Video matching. ACM Trans-
actions on Graphics, 23(3):592–599.
Vinicius, P., Borges, K., Tews, A., and Haddon, D. (2012).
Pedestrian detection in industrial environments: See-
ing around corners. In Proceedings of 2012 IEEE In-
ternational Conference on Intelligent Robots and Sys-
tems, pages 4231–4232.
Wang, M. and Wang, X. (2011). Automatic adaptation of a
generic pedestrian detector to a specific traffic scene.
In Proceedings of 2011 IEEE Computer Society Con-
ference on Computer Vision and Pattern Recognition,
pages 3401–3408.
W¨ohler, C. (2002). Autonomous in situ training of clas-
sification modules in real-time vision systems and its
application to pedestrian recognition. Pattern Recog-
nition Letters, 23(11):1263–1270.
EnvironmentAdaptivePedestrianDetectionusingIn-vehicleCameraandGPS
361